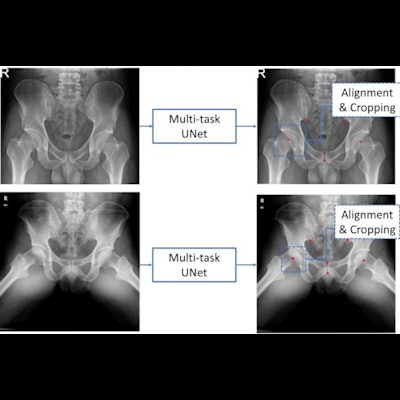
An artificial intelligence (AI) deep-learning model trained on x-rays may provide orthopedic surgeons with an accurate second opinion when diagnosing hip disorders, according to a study published online March 31 in Osteoarthritis Imaging.
A group led by researchers at Shanghai Jiao Tong University tested a model developed by Fosun Aitrox for assessing the severity of primary hip osteoarthritis and femoral head osteonecrosis. The AI model performed better than specialists and could provide a second opinion in these cases, the authors suggested.
"This is the first study to investigate AI-assisted detection of two types of hip diseases on digital radiography, which is much more challenging and a step closer to routine clinical scenarios," wrote first author Chen Chen, PhD.
Hip osteoarthritis is the most prevalent and debilitating of hip disorders around the world and can be divided into primary hip osteoarthritis -- when neither an anatomic abnormality nor any specific disease process can be identified -- and secondary hip osteoarthritis, which results from several different patterns of joint failure, such as osteonecrosis of the femoral head, the authors explained.
Detecting early subtle differences among these disorders is challenging on x-ray for orthopedic surgeons, but crucial, given that osteonecrosis of the femoral head can result in rapid deterioration of the hip joint, the group added.
To develop AI that could help determine the severity of these disorders, the researchers trained a deep-learning model on a dataset of 56,597 hip x-ray images. X-rays were labeled as normal or primary hip osteoarthritis ranging in severity from I to III or osteonecrosis of the femoral head ranging from II to IV by a panel of 10 orthopedic surgeons.
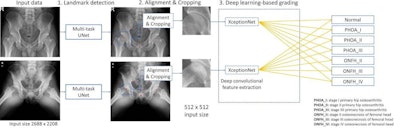
On the testing dataset of 811 hip joint x-rays that included 615 normal hips, 22 osteonecroses of the femoral head hips, and 174 primary hip osteoarthritis hips, the model achieved an overall score of 94.9% for correctly identifying the disorders, the investigators found. Average sensitivity for identifying all classes of the deep learning model was better in comparison with board-certified orthopedic surgeons (78% vs. 77%), they reported.
"Our study demonstrated that we could train a single deep learning model to grade the severity of multiple hip disorders on digital radiographs," the group noted. "The model may be used to provide a second opinion for severity grading hip disorders on digital radiographs."
In the end, despite the growing role of MRI and CT in diagnosing hip disorders, pelvic radiography remains the most cost-effective and widely accepted screening tool, especially in remote or low-resource areas, the authors wrote. And given that both primary hip osteoarthritis and osteonecrosis of the femoral head require surgical intervention in later stages, methods for early diagnosis remain a priority.
While this study provided promising results for use of a DL model in such settings, further research is warranted, according to the authors.
"Future, multicenter prospective clinical trials are now needed to ensure clinical applicability of the DL model," they concluded.