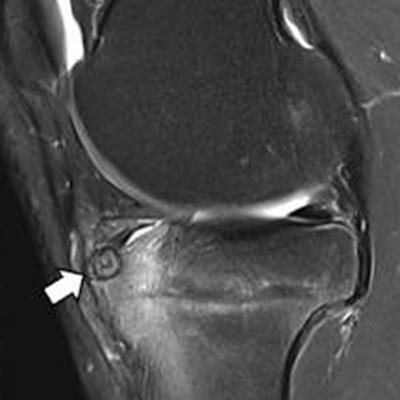
Artificial intelligence (AI)-based image reconstruction can deliver real-world benefits for MRI, enabling faster acquisition times and opening up new clinical applications for the modality.
And researchers are continuing efforts to demonstrate the viability of the technology. For example, a multivendor multisite study assessing the performance of an AI algorithm for speeding up MR image reconstruction has now reached the analysis stage.
The study, organized by researchers from New York University (NYU), seeks to show that accelerated AI-reconstructed images are interchangeable and generalizable across multiple vendors and sites, with the aim of making MRI globally more accessible to patients.
Years of NYU research into AI to make MRI faster while safeguarding quality have culminated in the current study, noted Dr. Michael Recht, chair of radiology at NYU Grossman School of Medicine.
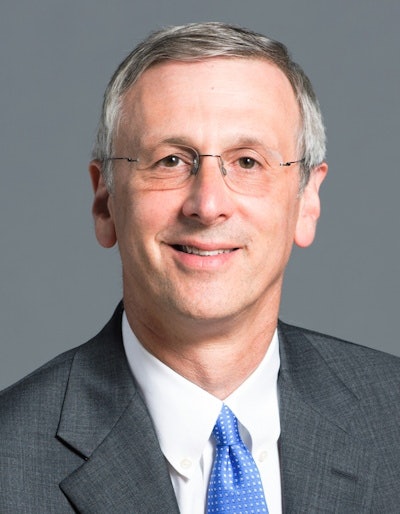
He explained that to ensure optimal AI image quality, NYU collaborated with Meta AI (formerly FaceBook) in open-source research. As a result, a deep-learning algorithm was shown to accelerate MRI of the knee by a factor of four with excellent image quality. NYU subsequently made a huge amount of MRI data publicly available and extended an official "fastMRI challenge" in 2018 to further research into AI image reconstruction.
After the challenge, NYU wanted to test its original algorithm, which had not yet been proven to be generalizable with a multivendor study.
The study has now been running for two years across multiple sites. Radiologists from across the country are reading the same cases with both accelerated, AI-reconstructed images and standard reconstructed images to see if they arrive at the same diagnosis. The readers are also rating image quality.
The final results of the study will hopefully show that the images and image quality of the two types of images are interchangeable. So far, previous NYU studies suggest that readers prefer the image quality of the deep-learning images, according to Recht.
Gaining ground
"For accelerated deep-learning MRI to really take off we need to prove that it can be used by all vendors, as we are aiming to do with our study," Recht told AuntMinnie.com. "Another exciting development is that all of the major MR vendors have now developed their own algorithms and incorporated them into their own software now used in clinical routine -- the original goal of the collaboration."
He also believes there is scope to go further. At NYU, researchers led by Dr. Jan Fritz, chief of MSK radiology at NYU Langone Health, are using a variety of techniques, for example, that combine acceleration with simultaneous multislice imaging, and techniques such as super-resolution and denoising, to create better AI images. Furthermore, instead of accelerating by a factor of two or four, acceleration can take place at much higher levels such as by a factor of six and eight, he noted.
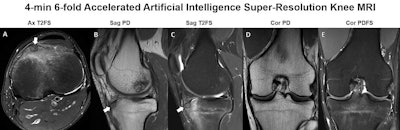
"Now we're doing shoulder exams in three to four minutes whereas until recently they were taking 15 to 20 minutes," Recht said. "These techniques have the potential to completely disrupt current practice. We know that for sports injuries, MRI provides more information than x-ray so if it can be faster, it will be cheaper and more accessible, and my hope is we'll start using it as a first-line treatment."
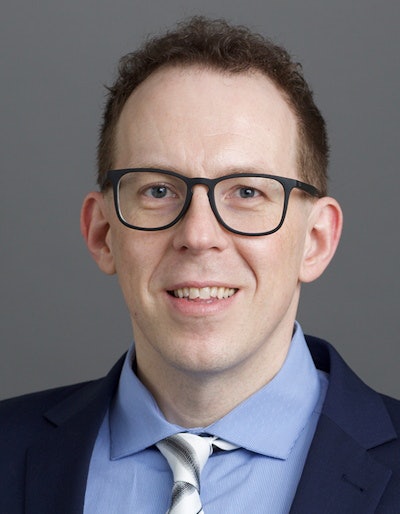
Because neither the algorithm nor the research in the study is proprietary, this translates to global use in clinical practice, noted NYU's Dr. Jan Fritz. Furthermore, as more vendors incorporate it, the algorithm will evolve further, he added.
"Before speed was inversely proportional to image quality," Fritz said. "With AI we've broken the curve and can go faster with the same or even better quality. There is no sacrifice or trade-off."
As AI algorithms develop they may allow lower field strengths to produce excellent image quality and this may make units cheaper.
"There could be a change in how machines are built and maintained, making total cost of ownership lower and more accessible to poorer countries," Fritz said.
True reflection
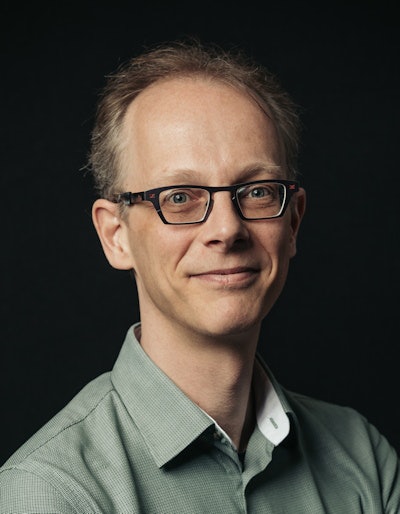
One of the main worries with AI was that it would make up information, but the solution of continuously going back to measured data, and only using AI to accelerate and fill in the gaps ensures algorithms provide the radiologist with an image that really reflects the subject and that has extracted fact from fiction, according to Matthias van Osch, PhD, a MRI physicist at Leiden University Medical Center (LUMC) in the Netherlands.
Capacity to constantly refer to measured data in an iterative cycle process is embedded in the Philips' SmartSpeed algorithm, based on one of the winning models from the LUMC and Philips team for the fastMRI challenge in 2019. Co-creation started that year with a multidisciplinary group and product development progressed beyond the challenge with SmartSpeed receiving U.S. Food and Drug Administration (FDA) clearance in May 2022. This collaboration continues to be implemented at the AI4MRI lab in Leiden, which is funded by a grant from the Dutch Research Council including financial support by Philips.
Third wave
Initially MRI speed increased through parallel imaging, and then via compressed sensing in which sparsity, as present in natural images and jpeg-compressions, allows reconstruction with much fewer, pseudo-randomly sampled measurements.
"In this third wave, AI takes acceleration to the next step. Besides using the 'sparsity' of a natural image, the algorithm learns what to expect in terms of texture and structure," van Osch said. "By incorporating this kind of prior knowledge, we can accelerate further."
Starting with the acquired MRI data, AI then generates a likely reconstruction. The algorithm continually compares this reconstruction to the measured data and then updates the estimate by an additional AI prediction step, making it increasingly realistic while still reflecting the measured data correctly, he explained.
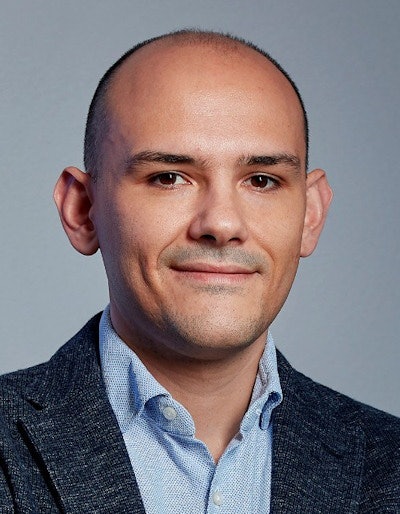
SmartSpeed can be applied on 95% of clinical protocols and on any hardware, noted Philips' Nicola Pezzotti, PhD, who was the fastMRI challenge team leader as well as expert member of the internal production team for SmartSpeed at Philips.
The method works better on 3D acquisitions, as acceleration takes place in two directions. Acceleration is still possible on 2D acquisitions, although at a more moderate level, according to Pezzotti.
"A key measure of success is forgetting there is AI in it," Pezzotti said. "One thing we paid attention to in the technique was not adding extra parameters which might confuse MR-technicians."
Faster MRI
"Starting from a really developed optimized protocol using compressed sensing, conservatively we can get 60% faster with SmartSpeed and we have examples of spine imaging protocols reduced from three minutes down to one minute," noted van Osch. "A key advantage is faster acquisition and the same reconstruction time as with compressed sensing."
Future applications
Current excitement now hinges on how AI will open niche clinical applications. For example, the AI4MRI lab is focussing on further acceleration in cardiac applications.
"Cardiac is tricky as it's a moving organ so you must scan even faster," Pezzotti said. "The idea of getting a full image in one heartbeat is very compelling and would also allow patients to breathe freely in the scanner. These are foreseeable scenarios."
Besides research into how AI can make MRI more resilient against patient motion, and multicontrast reconstructions that make use of the multiple contrast scans present in one examination, other projects will include taking the diagnostic confidence into consideration while scanning, with algorithms helping to decide whether or not the radiologist needs different contrast scans or higher resolution or whether the acquired scans are sufficient for answering the clinical question.
Hardware catch-up
One barrier to widespread AI for image reconstruction is that the original equipment manufacturers (OEMs) only offer their proprietary deep-learning reconstruction packages on the latest generation machines, noted Dr. Lawrence Tanenbaum of imaging services provider RadNet.
"Unfortunately, many of the scanners on the market wouldn't qualify without a substantial upgrade," Tanenbaum said. "Fortunately, there are vendor-agnostic solutions which are applicable to older machines regardless of vendor or field strength."
Synthetic images
AI reconstruction supports another new technology: synthetic image generation. Now approaching clinical use, synthetic imaging uses existing scans to create extra scans synthetically. Tanenbaum explained further:
"If a radiologist performs four three-minute scans normally, one can be removed, and exam time is reduced from 12 minutes to nine minutes when one series is synthetically generated," he said. "Furthermore, if one of the scans doesn't work out, in the traditional scenario the patient may need to be recalled for additional scanning. However, with the new technology, the missing scan could be synthesized from the others and this improves not only scan time but also patient experience."
Patient-centric MRI
"Patients really don't like being in scanners, with around 30% experiencing severe anxiety," Tanenbaum said. "If we can reduce the scan time from 20 minutes to six or seven minutes, that will make the difference. And if one scan can be synthesized that will make more of a difference too."
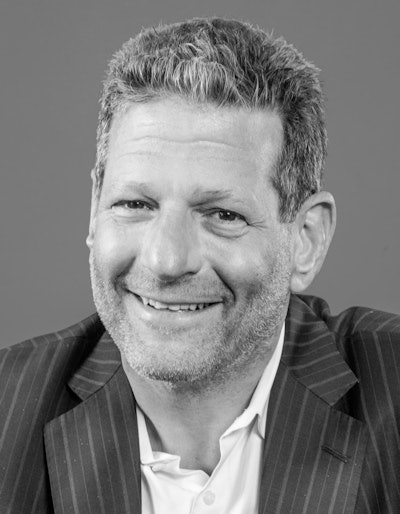
He pointed out that even though less comfortable than the institute's 1.5T scanner, the 3T scan experience is roughly three minutes shorter overall and net results from the patient satisfaction surveys are always higher than from the 1.5T, presumably due to the shorter scan times.
RadNet has rolled out the novel reconstruction packages offered by the OEMs on later-generation machines. When coupled with vendor-agnostic technology from Subtle Medical on older machines, RadNet is processing roughly 50,000 exams per month. With an average saving of about five minutes per exam, patients are spending around 250,000 fewer minutes a month in the scanners.
"AI reconstruction is not just about going faster, it's about creating a new standard of care involving better MR images with lower noise, higher resolution, and thinner slices," Tanenbaum said.