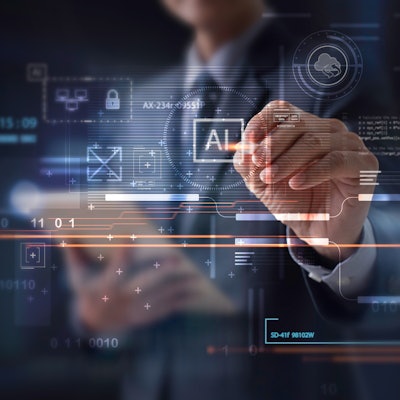
Radiologists at Lahey Hospital and Medical Center in Burlington, Massachusetts, have integrated six AI algorithms into their clinical workflow.
Christoph Wald, MD, PhD, told AuntMinnie.com. "Thankfully, our vendors were eager to collaborate and collectively I think we got to a good place."
Lahey and colleagues initially worked with a couple of vendors to incorporate U.S. Food and Drug Administration (FDA)-approved AI algorithms to triage stroke cases and to prioritize radiologists' work, given the number of images the overnight team had to read.
Triage of the cases was important in patients with potentially life-threatening conditions such as stroke due to intracerebral hemorrhage. The team integrated the AI technology with third-party radiology workflow orchestration software that's separate from the hospital's RIS and PACS.
Case prioritization
This created a new priority category that promotes those exams with AI-positive diagnosis to the top of the worklist so they can be interpreted first and then acted upon soonest by clinicians.
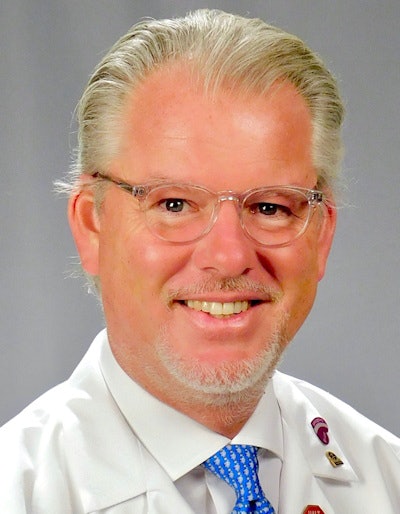
"While this occasionally results in an inappropriate prioritization of a false-positive case we generally had a good experience with this approach," said Wald, who also chairs the American College of Radiology (ACR) Commission on Informatics.
The system enables radiologists to see colored badges that indicate whether a case was subjected to AI processing and if it had a positive or negative diagnosis.
Wald said that in the early phases of the rollout, radiologists did not know when an AI algorithm was still processing a study because only the final AI state was shown.
"While this did not cause any harm it's essentially a missed opportunity," he said. "We therefore implemented a little gray badge to indicate that an imaging study was being processed. Radiologists can now decide whether or not to wait for the outcome."
Implementing enhancements
"If you use the AI processing for a diagnostic purpose or you want to quality-control your AI you'll need to review some representation of the actual AI output," Wald said. "We have the option to consult a color-coded heat map which is optionally displayed in a dedicated little widget that was provided by the AI company. It's separate from the PACS but activates and opens to the radiologist's view in context to the study which is being displayed in PACS."
Wald said other enhancements were also achieved during integration, such as a simultaneous display of the report impression if already available at the time of viewing.
"We also wanted to make sure that radiologists would always get the benefit of knowing the AI result and taking appropriate action, combining the human and machine efforts," he said.
When the system receives an AI result when a report is already in final status, the radiologist receives a warning containing a link that allows them to officially return to the study and reconcile the new AI finding with the actual imaging study.
Safety warning
Wald said the AI has worked well and improved workflow but it's important not to assume that FDA clearance of an AI tool means it will be safe and effective to use anywhere.
"It's absolutely critical for practices to evaluate algorithms before and after deployment as many local factors can seriously impact AI performance," he said.
Acquiring and acting on clinical feedback is another key consideration that Wald said Lahey has made easy to do to help with future AI research and vendor developments.
"We all have an obligation to conduct ongoing real-world performance assessment of AI in practice," he said.
Good news
Looking to the future, he told AuntMinnie.com, "I am excited about quantitative AI, multifunctional AI suites, and opportunistic screening. However, I think the biggest future workflow enhancements for radiologists will actually come from GPT-based generative AI use, much more than the single-trick pony-style AI we have seen to date."
These new types of models are likely to transform how radiologists consume upstream clinical information, as well as generate and output future radiologist work products – much of which has natural language processing as its core and as its currency, according to Wald.
"That's good news for both radiologists and their patients and referring MDs since the status quo is barely manageable, especially in areas of the world where there is a radiologist shortage," Wald said.
Demonstrating value
Many AI algorithms are already "entering the mainstream" in radiology workflow but other, even very "exciting" models may be more challenging to implement, said Greg Zaharchuk, MD, PhD, who is a professor of radiology (neuroimaging and neurointervention) at Stanford University in Stanford, California.
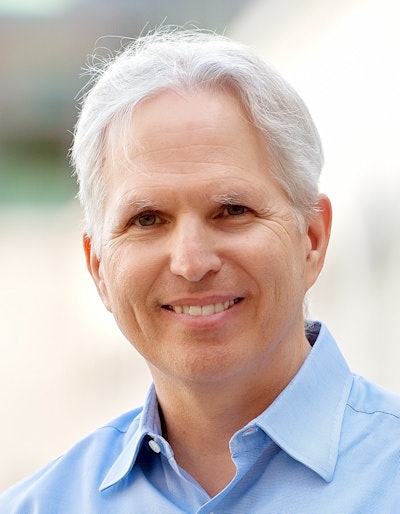
"A lot of things are possible but the opportunity for widespread clinical adoption is limited by the inability to show either a cost-neutral effect or some sort of cost-savings," noted Zaharchuk, who is past president of the American Society of Functional Neuroradiology and a deputy editor at the Journal of Magnetic Resonance Imaging. "Many fantastic AI algorithms being developed today struggle frankly to prove their value."
Zaharchuk, along with scientists and engineers at AI software developer Subtle Medical, is working with AI software to reduce the use of gadolinium-based contrast agents for contrast-enhanced MRI exams while maintaining excellent image quality, he said. Their deep-learning method learned to approximate full-dose scans from zero-dose and low-dose images so that gadolinium dose could be reduced -- by some 90% without sacrificing diagnostic quality, research suggested.
Zaharchuk pointed out how AI is already gaining ground as a means of improving efficiency in radiology, particularly in MRI given the high cost of running scanners. The technology is enabling quicker image acquisition and low-dose exams, he said.
Unappreciated functional benefits?
"One really useful value of AI is to decrease the amount of tedious things that people do and that people can get wrong -- whether that's a technologist or a radiologist," he said.
"So there are opportunities to improve not only image acquisition but also a lot of mundane tasks that require a lot of personal attention and effort."
Zaharchuk cited AI-assisted automation of "back-office type" functions in billing and scheduling, and the potential for natural language processing to reduce the time it takes to produce a report for radiologists.
"I think these are all very good uses that aren't necessarily the first things that come to mind when you think about AI in radiology," he said.
Demonstrating value
Zaharchuk noted, "If something's not going to make you more efficient, if it's something for better patient care, you have to justify it based on outcomes and, for that, the documentation has to be really clear and really obvious so that you can't ignore it."
He said outcome benefits may be challenging to demonstrate but evidence can be produced, usually when the questions AI algorithms are being deployed to address are "clear and simple."
It is possible, he said, in areas such as mammography, that lesion detection algorithms can show benefit when using AI.
"Once this is settled, you can work on getting such uses reimbursed," he said.
Zaharchuk's experience in addressing gadolinium use is relevant to other future potential uses of AI. Gadolinium is a heavy metal that shows up in higher concentration in wastewater downstream of MRI areas and has inevitably raised environmental concerns.
Future AI solutions that can address environmental issues such as these "may find a niche in clinical practice," he speculated.
In addition, Zaharchuk said he is excited about the potential for AI to "standardize image naming," ensuring that millions of images are appropriately identified and labeled so they can be read by practitioners more efficiently and used for downstream algorithms in a seamless fashion.
A helping hand
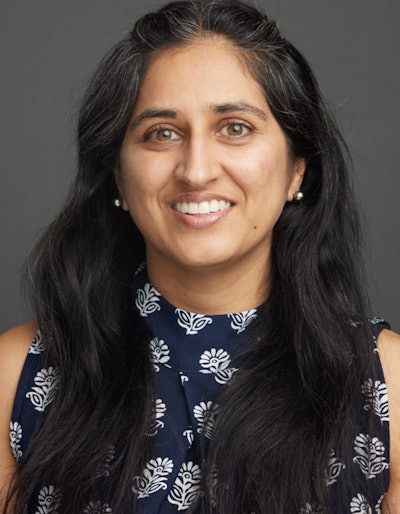
Kirti Magudia, MD, an assistant professor of radiology at Duke University School of Medicine, in Durham, NC, co-chairs the Imaging AI in Practice (AIIP) Task Force for the Radiological Society of North America (RSNA), which was set up to foster collaboration between developers of radiology systems, including AI applications.
At its annual meeting, the RSNA ran a demonstration project exploring the technology's many practical uses in case studies it posted online -- these show results of AI projects across the radiology workflow in a simulated clinical environment -- and the organization has analyzed the lessons to be learned so far.
Learning lessons
Among the key takeaways is the need for consistent and coordinated interoperability standards and orchestration profiles, and the importance of collaboration between radiologists and vendors in a fast-moving environment, said Magudia and colleagues.
In a report, published in Radiology in late 2021, they noted that whereas many imaging-focused AI vendors were expected to work exclusively with Digital Imaging and Communications in Medicine (DICOM) objects, many of the reporting-focused vendors were expected to work with Fast Healthcare Interoperability Resources (FHIR).
At the time of the demonstration project, Integrating the Healthcare Enterprise (IHE) profiles for AI Workflow Integration (AI-WI) and AI Results (AIR) were in development but not yet published. So there was "no consensus for conversion between DICOM and FHIR resources in this context," the study reported.
Another key lesson was the need for radiologist interaction with AI results. Even when AI results are not optimal, the mechanism of integration into a structured report can still provide efficiency gains if the results can be adjusted before generating structured data, according to the study.
Integration tips
Magudia urges any radiology practices that are seeking to integrate AI into the clinical workflow to use the resources provided by radiological societies such as the RSNA and the ACR Data Science Institute with their listing of FDA-approved imaging AI products.
"Think hard about what data was used to train the product you are considering and strongly consider testing the product on local data and continuous monitoring after that," she advised. "Also, look for products that use semantic and interoperability standards as well as orchestration profiles for successful clinical integration including report generation. Furthermore, carefully consider how AI results are going to be displayed."
What workflow applications does she look forward to seeing taken further in future?
"Opportunistic screening is an area that I am particularly interested in as an AI application," Magudia told AuntMinnie.com. "There is significant latent value in medical imaging that is not being harnessed generally because it would be too labor intensive to do so. These include coronary calcium scoring, body composition analysis, incidental pulmonary embolism detection, etc. Many of these tasks have been correlated with patient outcomes and have the potential to impact patient care."
Related Reading:
1. ACR case studies acr.org/Practice-Management-Quality-Informatics/Imaging-3/Case-Studies/Information-Technology/Integrating-AI-into-the-Clinical-Workflow
2. The Informatics e-Learning Hub: Bringing AI to Practice (acr.org)