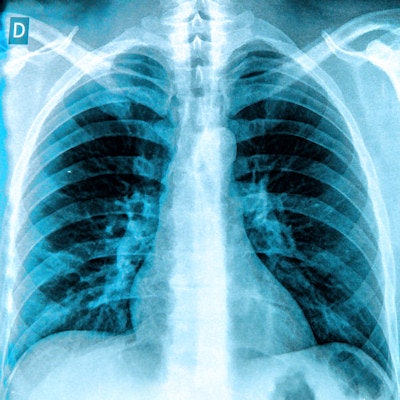
Rad-Aid International investigators have described a successful strategy for deploying chest x-ray AI to help diagnose tuberculosis in low- and middle-income countries, according to a recent study in the Journal of the American College of Radiology.
Ultimately, the approach can help avoid unintended consequences to patients and healthcare systems, noted first author Samantha Singh, MD, of Georgetown Public Hospital Corporation in Georgetown, Guyana, and colleagues at the University of Pennsylvania.
"It is critical for radiologists to remain as gatekeepers for effective and ethical use of AI in medical imaging worldwide by providing guidance on its implementation," the group wrote.
Rad-Aid is a not-for-profit global health radiology organization established in 2008 with a mission to improve and optimize access to medical imaging and radiology in low-resource regions of the world. In this study, the group used a three-pronged approach that focused on education, infrastructure implementation, and phased introduction, and used it successfully in hospitals in Guyana and Nigeria.
During a similar period, the group first installed a picture archiving and communication system (PACS) with a cloud component at both institutions. Radiology residents and attendings at the hospitals then completed an introduction to AI course to prime them to use the AI software, an algorithm developed by Qure.ai. Finally, they compared AI outputs to standardized prospectively generated reports by local radiologists and study team members.
Thirty participants completed the introduction to AI course, with an average preknowledge test score of 75% and post-test score of 95%, according to the findings. The focus of the validation process in various sites was reflective of the intended use of the AI software, the group wrote.
Specifically, in Guyana, it revealed an 87% concordance rate between radiologists and the AI model for triaging normal versus abnormal chest x-rays. In Nigeria, the researchers noted an 85% concordance rate between radiologists and the AI model for reporting tuberculosis on chest x-rays.
"The AI software was successfully deployed and is being used as intended in both institutions," the team wrote.
The group noted an important takeaway -- that having a specific task for an AI model to perform in these settings was crucial because this likely improved its use, they wrote. Conversely, AI algorithms that perform multiple tasks typically don't have the same accuracy across all tasks, and so it is prudent to limit its output to high-accuracy tasks, especially in low resource settings where supervision might be limited, they suggested.
Moving forward, the authors said they intend to conduct periodic quality assurance reviews of the AI outputs.
"Implementation of other AI solutions at these sites and expansions to other sites are in the pipeline for further work," the group concluded.
The full article can be found here.