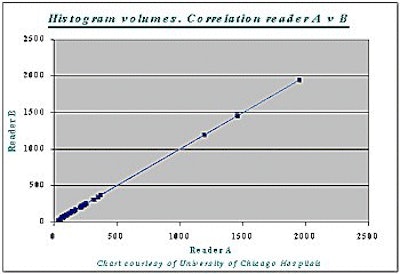
Precise tumor volume measurement is critical in cancer therapy, both for routine staging and for measuring treatment response. Yet even the most accurate techniques are slow and labor-intensive enough to rank as "the bane of our collective existence," according to Dr. Abraham Dachman, associate professor of radiology and director of CT at the University of Chicago Hospitals.
In a study presented at the November 2000 RSNA meeting, Dachman concluded that semiautomated tumor measurements based on histograms were accurate compared to a gold standard, and highly reproducible among readers. Once the segmentation process is automated as well, tumor measurement will speed up enough to have a real impact on patient care, he said.
A variety of techniques and equations can be used to measure tumors, and many of them yield accurate results. However, the accuracy of any method can depend on how and where it's applied, and standardized techniques still don't exist for many applications. The World Health Organization's criteria for treatment response are based on bidimensional measurement of tumor diameter, for example, while the U.S. National Cancer Institute recommends the use of unidimensional techniques.
Tumor volume can be measured in many ways too, using equations based on the z-axis diameter, or on standard shapes such as the sphere, ellipsoid, or cube. Each method requires painstaking labor to produce accurate results.
On the other hand, histograms can be produced rapidly, using software that's available on most commercial workstations. Histogram software has improved markedly in recent years too -- and histograms could be paired with automated segmentation software that could be developed to speed up tumor measurement. But first things first. The accuracy of histograms hasn't been well studied, Dachman said.
Thus began the team's effort to eventually speed up tumor measurement by measuring the accuracy and interobserver variability of histographic representations of segmented liver voxels. In the study, measurements gleaned from histograms were compared with those obtained via manual segmentation of liver using the summation-of-areas technique as the gold standard, Dachman said.
Two readers each evaluated the data from 30 spiral CT scans. All patients had biopsy-proven primary colorectal cancer and evidence of progressive hepatic metastatic disease.
The CT scans of the abdomen were performed on a GE 9800 HiSpeed Advantage scanner (GE Medical Systems, Waukesha, WI) using 7-mm collimation, and a pitch of 1.1; following administration of nonionic intravenous contrast material (140 cc iohexol 300 mg/ml, IV2-2.5 cc/sec, 70 sec. delay). The data was reconstructed and viewed on a GE Advantage Windows Workstation (version 3.2).
Manual segmentation was performed by viewing all images on a soft-tissue window (W=340, L=40). The readers outlined each lesion on each axial slice with a computer mouse. Lesion volume was calculated automatically by mutiplying the sum of the areas from each slice by the reconstruction interval (the summation-of-areas technique).
"We established certain guidelines. For example, if greater than 50% of the vessel diameter was interhepatic it was included, whereas if it was extrahepatic it was excluded," Dachman said. "We excluded fat, the portal vein, the gallbladder...and hyperattenuating tumors. We excluded transient hyperattenuating defects that were around the lesions, and for those few cases that didn't have well-defined margins, the observer had to give his best guess for the margins."
The data from each case was then applied to histograms, which were evaluated at different times from the manually segmented data to reduce the possibility of memory bias. Tumor volume for the histograms was based on what fell between the upper and lower points of a data curve created by the tumor.
"After several experiments we focused on looking at the inflection curve for the upper threshold, and a fixed value of 10 HU for the lower threshold of liver volumes. Basically everything on the curve is included for the volume of the liver."
According to the results, the median liver volume was 1,676 cc, minimum 1,131 cc, maximum 4,274 cc. The median tumor volume was 137 cc, the minimum was 9.8 cc, and the maximum was 1,891 cc.
Correlation between the histogram measurement and the gold standard measurement was 99% (r=0.99) -- for both readers A and B, and for liver as well as tumor volumes (see chart below).
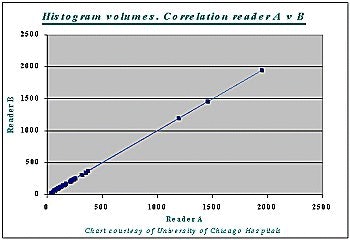
At the same time, differences in tumor burden between cases produced wide variations in accuracy.
"We actually did two analyses, one looking at all the cases, and ... another looking at cases over 100 cc of tumor volume," Dachman said. "If you look at the second analysis of histogram data, the percentage error for tumors greater than 100 cc vs. less than 100 cc of tumor, it's obvious that where the lesions are smaller, a small change in the diameter of the tumor will produce a major change in volume. Whereas in tumors greater than 100 cc, the percentage error was small."
Specifically, the percentage error of the histogram method for all lesions for reader A (reader B) was median 0%, range -21% to 546% (median 3%, range -64% to 959%). The percentage error of the histogram method for cases with a tumor burden > 100 cc for reader A (reader B) was median -3%, range -17% to 11% (-6%, range -63% to 35%). For cases with a tumor burden < 100 cc, the percentage error of the histogram method for reader A (reader B) was median -3%, range 79% to 546% (203%, range -4% to 959%).
There was excellent agreement between the observers, Dachman concluded. He also noted potential study bias based on differences in size, shape, and distribution of tumors, and in the exclusion of hyperattenuating tumors. And while the metastases were not proven histologically, every single tumor was followed up in subsequent scans, he said.
"Certainly the results are software-specific, but there's no reason to believe that software from different vendors wouldn't perform the same way. If someone develops a software program that can speed up the segmentation of the liver, then the histogram can be applied very rapidly, even by a non-radiologist."
In the future look for fully automated hepatic lesion segmentation, and automated measurement in other tumors, organs, and modalities, Dachman said.
By Eric BarnesAuntMinnie.com staff writer
January 31, 2001
Click here to post your comments about this story. Please include the headline of the article in your message.
Copyright © 2001 AuntMinnie.com