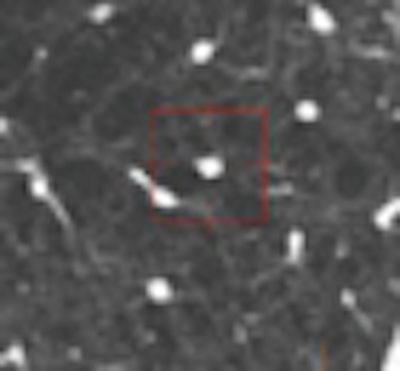
A painstakingly compiled database of CT lung scans was no match for a university's computer-aided detection (CAD) system. The CAD system found more lung nodules in more patients than the original expert reader panel, which had reviewed each scan twice.
The results suggest that CAD is more efficient at finding nodules than human readers, and more diligent than humans when faced with multiple findings in the same patient. The availability of multiplanar views by the university team that evaluated the CAD output may also have significantly aided detection sensitivity and specificity.
Perhaps the most surprising aspect of the results from Stanford University was the particular data their CAD algorithm and readers had outperformed: a National Cancer Institute (NCI)-sponsored database of CT lung scans, double-read by expert thoracic radiologists participating in the Lung Imaging Database Consortium (LIDC).
LIDC, a lung imaging database project funded by the NCI in cooperation with five academic centers, is tasked with building a repository of expertly reviewed lung scanning cases to test lung CAD systems.
LIDC's unique two-phase data collection process allows each radiologist to review and annotate the others' results via the Internet. Approximately 100 initial cases are now available on the National Cancer Imaging Archive (NCIA) Web site, for use by researchers developing lung CAD applications. That much of the database is ready for testing.
"The next extension of the LIDC is what happens when scientists in the public try to work with the [cases] for the purpose for which they're intended," said lead researcher Dr. Geoffrey Rubin, professor of radiology at Stanford University in Stanford, CA.
The study aimed to measure how well the LIDC's exert panel detections worked with the university group's previously validated surface normal overlap CAD (SNO-CAD) algorithm on thin-section CT datasets.
Because only 36 of the available LIDC datasets had been acquired with section thicknesses of 2 mm or less, the university-based radiologists limited their study to that subset of 36 cases, each of which was associated with an XML file incorporating the color-coded markings of all four LIDC readers. In an effort to preserve the variability of findings among the expert LIDC readers, no single reference standard had been compiled for the database.
In their study, the Stanford team assessed the 36 cases with CAD and their own team of four experienced radiologists to review the CAD output.
"We elected to assign each [nodule] detection a value from one to four, corresponding to the number of LIDC readers who determined that the final detection was a true nodule," Rubin said in a presentation at the 2008 International Symposium on Multidetector-Row CT in San Francisco.
The 36 cases were then analyzed by the SNO-CAD algorithm. Then the CAD output plus the LIDC detections were presented randomly to four experienced Stanford radiologists, using a multiplanar imaging application the group had previously developed for assessing nodules.
"That platform, in addition to showing transverse sections and highlighting the area of detection, also presents multiplanar reformations like coronal views and volume rendering, which we feel are critical for the confident characterization and assessment of nodules," Rubin said. "The readers of LIDC only assessed transverse sections; they don't have a multiplanar tool that allows them to look from a multidimensional perspective."
The Stanford radiologists recorded the size, shape, and lesion composition for each detected noncalcified lesion that was 3 mm or larger and deemed true-positive with the aid of CAD.
While the database included 150 nodules considered positive by the LIDC readers, the team of CAD plus the university readers called 251 nodules positive. After eliminating an additional nine LIDC-positive nodules deemed false-positive by the Stanford team, a total of 110 "true-positive" nodules remained that had not been detected by the LIDC readers, Rubin said.
Looking at the numbers of positive nodules agreed on by one, two, three, and four readers from each team, respectively, shows that "the availability of CAD resulted in more nodules being detected than by LIDC," Rubin said.
![]() |
CT lung scans from 36 patients included 200 nodule candidates detected by CAD and 150 nodules considered positive by LIDC radiologists working without CAD or multiplanar image reconstructions. A second group of university researchers working with CAD and multiplanar reconstructions called 251 nodules positive. After the university group eliminated nine LIDC-detected nodules as false-positive, 110 "true-positive" nodules remained that had not been called by the LIDC researchers. CAD chart (above) shows the number of nodules deemed positive by one, two, three, and four readers from each research team, respectively. The availability of CAD resulted in more nodules being detected by the university researchers compared to the LIDC team. All images courtesy of Dr. Geoffrey Rubin. |
"So despite the tremendous scrutiny applied by highly experienced LIDC readers over the course of two readings, these data would suggest that CAD is finding additional nodules within these datasets," he said.
What is a nodule, and how is it viewed?
Unfortunately, the data also reveal a high level of discordance among the readers in choosing positive nodules -- among the university readers as well as the LIDC team.
Even in the university evaluation, determined with the aid of 3D evaluation and presumably more conclusive, "well over half of the nodules called positive by one reader were not agreed upon by all four," Rubin said. "This highlights an important challenge in these studies, and that is the definition of what a true nodule is. CAD detections are being shown to four readers, but there's a lot of variability in terms of whether they're willing to agree and accept them or not."
After CAD had detected the nodules, multiplanar reconstructions seemed to help the Stanford group characterize them as well -- a second key advantage for the university readers.
One example, a nodule that looked like an adjacent vessel in the transverse plane, was seen clearly as a lung nodule in coronal, sagittal, and volume-rendered views. "I'm quite certain that the LIDC group is missing nodules because of the limitation of only transverse section presentation," Rubin said.
![]() |
CT finding (left) has the appearance of an adjacent vessel in transverse-section reconstruction and was not called by any of the four LIDC readers. After viewing transverse, coronal, sagittal, and volume-rendered reconstructions (right), all four university readers called the finding a lung nodule. |
Satisfaction of search
The results also suggest that one element of CAD's success relates to the satisfaction of search among radiologists. "As radiologists find a certain number of nodules they tend to fall off on their detectability curve," Rubin said, adding that the clinical significance of the incremental findings by radiologists is debatable.
![]() |
Study results suggest that satisfaction of search among radiologists may contribute to CAD's success in finding additional nodules. X-axis on chart (above) indicates the number of detected nodules for a given scan; y-axis shows additional CAD-detected nodules. The high correlation coefficient (r = 0.803) suggests that the availability of CAD resulted in more nodules being detected (p < 0.001). |
Different features
The study revealed differences in the features of CAD-detected nodules compared to the LIDC findings -- some of them counterintuitive, Rubin said.
For example, CAD-detected lesions were generally larger than the reader-detected findings, though one might have expected them to be smaller. The average diameter of incremental detections by CAD was 4.7 mm, versus 3.3 mm for nodules found by the LIDC team (p < 0.001). LIDC-detected nodules also tended to be more solid with fewer ground-glass opacities (p < 0.001). And they were more sharply marginated (p < 0.001) compared to the CAD-detected findings. What does it all mean?
"First, CAD seems to detect more true-positive nodules than the [LIDC] search," Rubin said. And because most lung CT findings aren't proven, it's important to "appreciate that we have no true reference standard," he said. "Advanced [multiplanar] visualization probably helps validate CAD detections. Nodule agreement among readers is pretty lousy, and I think that's demonstrated in the LIDC reader group as well as the Stanford group."
Finally, a better definition of what constitutes a nodule will be needed to confidently assess the performance of independent CAD systems.
By Eric Barnes
AuntMinnie.com staff writer
August 12, 2008
Related Reading
CT databases aimed at lung imaging research, August 8, 2008
CAD helps residents identify lung nodules on DR, May 20, 2008
Criteria determine CAD mark sensitivity, March 20, 2008
Divergent research on CT lung screening sparks more debate, fewer answers, April 19, 2007
Management strategies evolve as CT finds more nodules, August 19, 2005
Copyright © 2008 AuntMinnie.com