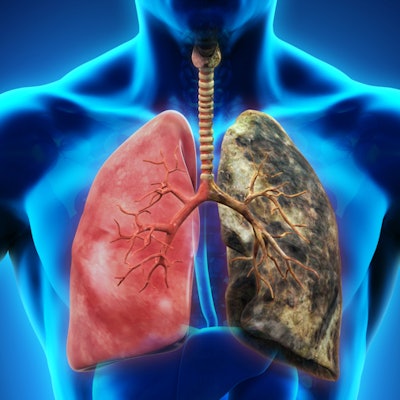
A Canadian model for predicting lung cancer risk based on nodule and patient characteristics distinguished benign from malignant lung nodules in patients in the National Lung Screening Trial (NLST), according to a study presented at RSNA 2016. A second study in the same session, however, found that radiologists slightly outperformed the model.
Called the Vancouver Lung Cancer Risk Prediction Model, the algorithm's high accuracy for predicting malignancy in the NLST dataset persisted despite important differences between the NLST patient data and those used in earlier studies. For example, NLST had fewer nodules per patient but more emphysema than two earlier studies.
Receiver operating characteristic (ROC) analysis showed that the Vancouver model did a good job in discriminating benign and malignant nodules similar to a second Canadian study, and it would be valuable to assess the approach in a clinical environment to determine its value, said presenter Dr. Charles White from the University of Baltimore in his RSNA talk.
Validated in smaller trials
Annual low-dose lung cancer screening with CT generates large quantities of suspicious nodules, creating headaches for clinicians who need definitive answers regarding which nodules are more likely to be malignant and, therefore, require invasive follow-up with biopsy to determine malignancy or benignity. A strong model for predicting which patients might develop cancer could offer important clues in borderline cases, potentially reducing the number of invasive biopsies and the cost of care.
The Vancouver prediction technique assesses the risk of malignancy based on several patient and nodule characteristics, including solid or nonsolid, smooth or speculated, nodule height and location, and presence or absence of emphysema, as well as patient demographics such as gender and age. The model worked well in two previous datasets: the Pan-Canadian Early Detection of Lung Cancer Study (PanCan) study of nearly 2,000 patients and an analysis performed by the British Columbia Cancer Agency (BCCA), which involved about 1,000 participants in a study sponsored by the U.S. National Cancer Institute.
Would the Vancouver model work with the far-larger NLST study and its patients with different nodule characteristics and demographics? The study presented at RSNA 2016 aimed to assess the likelihood of malignancy among a subset of nodules in NLST using. The study included only the initial prevalence scan; follow-up incidence scans were excluded, White said. The data were analyzed and entered into the Vancouver model for each of the nodule and non-nodule parameters.
"For the risk calculator, there were quite a number of patients who had to be excluded for two major reasons: patients who had multiple nodules, but it wasn't indicated which were malignant," White said.
Based on output from the risk calculator, the investigators tested multiple thresholds to distinguish benign from malignant nodules using the NLST dataset. They used an optimized threshold value to determine positive and negative predictive values, and a full logistic regression-model was applied to the NLST data.
When the researchers tested various cancer risk thresholds ranging from 20% to 30% likelihood of malignancy, they found that a 10% threshold separating nodules designated benign from malignant gave the best accuracy at 94%, he said. They used the chi-square test, t-test, and ROC analysis (area under the curve [AUC]) for statistical analysis.
"Because of NLST data entry limitations, only 39% of subject CT scans were includable," White said. Still, the analysis boasted 2,819 studies with 4,431 nodules -- a larger number of patients than the PanCan study but a smaller number of nodules. Overall, fewer in the NLST cohort had nonsolid nodules at 8% versus 20% for PanCan and 10% for the BCCA study.
The reduced number of nodules per study in NLST was likely due to its higher cutoff size to be deemed a positive nodule, White said. For the PanCan study, any nodule larger than 1 mm to 2 mm qualified for inclusion versus a 4-mm minimum diameter for NLST.
In all, the study evaluated 4,431 nodules (including 4,315 benign and 116 malignant). Along with fewer nodules per case in NLST, the researchers found more nodule spiculation and emphysema. A threshold value of 10% risk of malignancy was determined to be optimal, revealing sensitivity of 85.3%, specificity of 85.9%, positive and negative predictive values of 27.4%, and 99.6%, respectively. The ROC curve for the full regression model applied to the NLST database revealed an AUC of 0.963.
Performance of Vancouver risk model 3 datasets | ||
Dataset | AUC | 95% confidence interval |
PanCan development dataset (full model) | 0.942 | 0.909-0.967 |
BCCA validation dataset (full model without spiculation) | 0.970 | 0.945-0.986 |
NLST validation dataset | 0.963 | 0.945-0.974 |
As a standalone tool, the prediction model yielded a high discriminant value for benign and malignant nodules in NLST similar to that of the Vancouver study, and the results suggest that "it would be valuable to assess this approach in a clinical environment to determine its value," White said.
Among the study's limitations were the low number of positive cases and the lack of an economic analysis of the results of following the model for patient management.
Controlled study
The second study presented during the lung cancer screening session at RSNA 2016 seemed less promising. University of Chicago researchers looked at the performance of the Vancouver model for predicting the likelihood of malignancy in a simulated clinical environment with three different reader configurations: model alone, radiologists, and radiologists using the model.
"We conducted an observer performance test to compare the accuracy of the model with that of radiologists in a simulated clinical environment," said Dr. Heber MacMahon, a professor of radiology at the university. "We deliberately chose both junior residents who had less than eight weeks' training and experienced radiologists."
In this study, researchers tested the model using both automated and manual feature extraction on 100 NLST cases that included 20 proven cancers and 80 matched benign nodules, using six observers.
The model worked well in the controlled setting -- but not quite as well as experienced radiologists and trainees, who were significantly more accurate in estimating the risk of malignancy in size-matched, screen-detected nodules, he said.
"This, of course, could not affect the area under the ROC curve for how the model acted alone," McMahon said in his talk. "It could only affect how the observers reacted to the model. ... We compared the standalone model, the unaided radiologist, and the radiologist using the model."
Predictive accuracy of the Vancouver prediction model | ||
Model alone (mean of 6 observers) |
Radiologists (mean of 6 observers) |
Radiologists plus model (mean of 6 observers) |
0.80 | 0.85 | 0.84 |
"The model alone was substantially less accurate than all the radiologists together," he said. "When we tested the chest radiologist against the junior radiologists, the chest radiologists do better as one would expect, but it looks like the residents still have some advantage over the model alone. The ROC curves showed advantages for the radiologists alone versus radiologists with the model."
Here, the Vancouver model had good overall accuracy based mainly on nodule size, but it was less accurate than experienced or trainee radiologists for estimating cancer risk on size-matched nodules, McMahon noted. This is likely because, in a size-matched setting, size is no longer a discriminating feature, while details of the morphology remain important, he explained. Also, the Vancouver model uses only limited variables for nodule morphology.
Finally, because most cancers in the NLST dataset are larger than the benign nodules, a size-matched cohort of nodules is sure to be larger than average, and larger models have more distinctive morphology needed to determine nodule type accurately, the researchers noted. As a result, the model did not improve the accuracy of radiologists for risk prediction in the setting of a controlled environment, McMahon said.