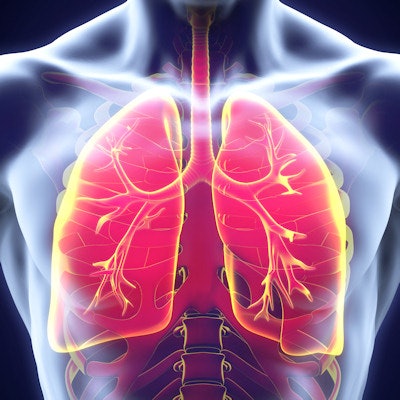
Recent studies have demonstrated the power of artificial intelligence (AI) software to detect disease, but how well can it predict future adverse events? The extent to which AI can assess smokers' risk for lung disease on CT was reported in a new study published in the American Journal of Respiratory and Critical Care Medicine.
A team of researchers from Brigham and Women's Hospital trained a deep-learning algorithm based on convolutional neural networks (CNNs) to provide a holistic evaluation of the lung condition of smokers using CT images. The algorithm correctly detected chronic obstructive pulmonary disease (COPD) on the CT scans of smokers, and it predicted the likelihood of acute respiratory disease (ARD) events and mortality (Am J Respir Crit Care Med, September 11, 2017).
The algorithm allows clinicians to fully utilize available CT data to make predictions, and for particular cases, it has been shown to be as good as an expert, co-lead author Germán González, PhD, told AuntMinnie.com.
"Usually the clinician looks at a CT scan and says, 'You're probably at moderate risk based on my interpretation,' which is good since it's based on clinical acumen. But to be able to add a number [to the prediction] would be beneficial in understanding risk," said co-lead author Dr. Samuel Ash.
Holistic interpretation
Previous research has demonstrated the usefulness of interpreting CT images to assess lung function and predict outcomes in patients with various lung diseases. A major challenge to this process, however, is that it requires a substantial understanding of the anatomic and physiologic implications of each disease.
Recently, machine-learning techniques have been used to bypass this step and jump directly to disease categorization. Prior research by Lakhani et al used a model of deep learning to accurately classify tuberculosis in chest x-rays.
Taking this concept one step further, the researchers conducting the current study developed a convolutional neural network model not only to detect COPD based on the CT images of smokers, but also to assess the risk for ARD and death.
"There is complex biology that is happening that you have to take into account [when assessing risk], and these networks can leverage that," said co-senior author Raúl San José Estépar, PhD. "You could take a holistic view of the image to go and predict the outcome you're targeting -- even mortality."
Before putting their CNN model to the test, the researchers used an algorithm to automatically extract four slices from preselected locations on the CT images and joined them into a single montage. The full collection of CT images was not used due to its immense file size.
The model was then "trained" to identify COPD and predict ARD using the CT scans of 7,983 smokers who had already been diagnosed with COPD in the COPDGene project, an ongoing study aimed at defining the causes and genetic risk factors associated with COPD. Once trained, the CNN algorithm evaluated a separate set of CT scans of 1,000 COPDGene participants, followed by scans of 1,672 different smokers participating in a similar COPD study known as ECLIPSE (Evaluation of COPD Longitudinally to Identify Predictive Surrogate Endpoints).
Detect, stage, predict
The CNN model correctly identified the presence or absence of COPD in 773 of the 1,000 COPDGene participants, with a "reasonably fit" prediction model compared with real-life patient outcomes.
Accuracy of CNN algorithm for detecting COPD and predicting ARD | |||
Detecting COPD (COPDGene) | Predicting ARD (COPDGene) | Predicting ARD (ECLIPSE) | |
Area under the curve | 0.856 | 0.64 | 0.55 |
Although not quite impeccable (a perfect prediction would score a "1"), the CNN model was able to detect COPD and predict ARD events relatively accurately. Participants were considered as having an ARD event if at least one took place within a year after the first scan.
In terms of staging, the model was able to accurately stage COPD in the COPDGene group in 51.1% of cases and was either correct or off by one stage 74.9% of the time. In the ECLIPSE group, the model accurately staged COPD in 29.4% of cases and was either correct or off by one stage 74.6% of the time.
The researchers were further able to use the CNN algorithm to predict the mortality of smokers with COPD. The model fared about as well as other, more rigorous methods of prediction -- the body mass index, airflow obstruction, dyspnea, and exercise capacity (BODE) index, as well as examining low attenuation areas.
Accuracy of techniques to predict mortality of smokers with COPD | |||
BODE index | Low attenuation area | Convolutional neural network | |
Area under the curve: COPDGene | 0.66 | 0.68 | 0.68 |
Area under the curve: ECLIPSE | 0.59 | 0.54 | 0.60 |
All in all, the deep-learning algorithm was able to identify individuals with COPD, characterize their disease severity, and predict other clinical outcomes including ARD events and death using just the CT imaging data, according to the researchers.
Old questions, new technology
The authors acknowledged several limitations of the study, including the need for more data and more powerful computational and memory capacities to optimize the model. But these shortcomings in no way disheartened the researchers.
What they may be seeing with the CNN model is enhanced imaging using only one data source and not multiple types of clinical data, co-senior author Dr. George Washko told AuntMinnie.com.
"These [deep-learning] techniques, when they reach widespread clinical practice, will expand what we already have, not replace the visual analysis techniques that have been honed for over a century," Ash added. "They allow for a different way of interpreting data and getting to a more quantitative approach that augments the already impressive ability for visual interpretation."
On a population level, such models may also be useful for spotting subgroups of patients who are at a higher risk of developing severe lung disease and targeting them for intervention, as well as assessing overall risk, the researchers suggested.
"I think it's exciting what this can bring to research and clinical care," Washko said. "In some ways, it's like starting over from scratch -- looking at old questions with new technology."