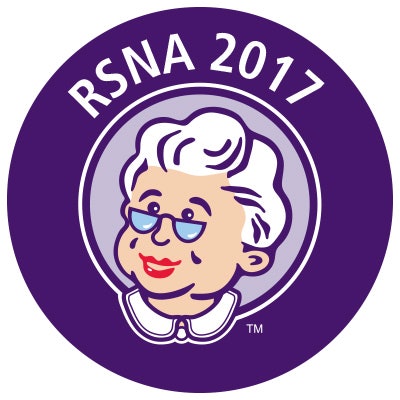
CHICAGO - A deep-learning algorithm can automatically calculate coronary artery calcium (CAC) scores from lung cancer screening CT exams and facilitate risk stratification in these patients, according to research presented on Tuesday at RSNA 2017.
Dutch researchers have developed a deep-learning algorithm that can directly quantify CAC scores from lung cancer screening CT studies. In testing, the algorithm yielded high performance for directly quantifying CAC scores and for stratifying patient risk.
"We can provide direct quantification of coronary artery calcifications and can even provide feedback on the decision to the radiologist," said Nikolas Lessmann of University Medical Center Utrecht. "Most importantly, we can provide risk stratification in real-time."
Lessmann presented the results on behalf of first author Bob D. de Vos.
Predictor of cardiovascular disease
CAC is a strong and independent predictor of cardiovascular disease, and it's an especially notable finding on lung cancer screening chest CT exams. However, reporting of the exact CAC burden on chest CT has been limited, mostly due to the cumbersome nature of manual scoring, Lessmann said.
"That is especially the case in ungated scans or low-dose CT scans, because the image quality hinders the manual scoring," he said.
A number of automatic methods are currently available for CAC scoring and can achieve close to a human level of performance. But many of these techniques have a high computational load and require dedicated servers, Lessmann said.
"They cannot be run on workstations," he said.
These automatic methods typically receive an input CT image, locate candidate lesions, and identify CAC. They then usually calculate a calcium score -- typically the Agatston score. This process can take five to 45 minutes to perform the detection and classification tasks, however.
Direct quantification
The Dutch researchers sought instead to quantify the Agatston score directly from the input CT image.
After first localizing the heart, their deep-learning algorithm extracts axial image slices. A regression convolutional neural network then analyzes the slices and calculates a calcium score for each slice. These scores are combined to provide a total patient calcium score, which can then be used for risk stratification purposes, according to Lessmann.
He said they used a relatively simple convolutional neural network with 60,000 trainable weights, six layers of convolutions and max pooling, two fully connected layers, and a single output. The algorithm was trained using 1,546 CT scans from the National Lung Screening Trial. Of these exams, 50% were used for training and 25% were utilized for validation. The remaining 25% served as the evaluation dataset.
In testing, the algorithm yielded excellent correlation with the reference Agatston score:
- Pearson's correlation coefficient: 0.97
- Spearman's rank correlation coefficient: 0.96
- Intraclass coefficient (ICC): 0.96
"Overall, the correlation was very high," Lessmann said.
When the calcium scores were used for risk stratification, the algorithm had 85% accuracy and a linearly weighted kappa of 90%.
Lessmann noted that their algorithm can automatically quantify a patient's calcium score in less than two seconds.
"This method is also computationally efficient and allows for implementation on workstations," he said.