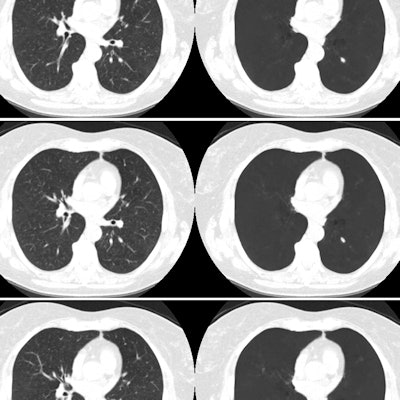
By suppressing vessels from lung cancer screening CT scans, a computer-aided detection (CAD) algorithm helped radiologists improve their ability to spot nodules as well as reduce their interpretation times, according to an article published in the March issue of the American Journal of Roentgenology.
In an examination of CT scans drawn from various lung cancer screening trials, radiologists correctly identified an average of 15.5% more malignant nodules when reading the scans with a CAD scheme than without it. What's more, using this CAD software decreased the amount of time they spent reading the scans by 26% (AJR, March 2018, Vol. 210:3, pp. 480-488).
"Radiologists using the computerized pulmonary vessel image-suppressed function with a built-in CAD (VIS/CAD) system significantly increased their detection of cancers on lung screening CT with somewhat lower specificity," first author ShihChung Lo, PhD, from Virginia Polytechnic Institute and State University told AuntMinnie.com. "Our study suggests that the technique would assist radiologists in the detection of additional clinically actionable nodules on thoracic CT."
Clearing extraneous vessels
Myriad studies have probed ways to improve the detection of lung cancer in its earliest stages and in its smallest form on CT scans, including using well-established strategies such as CAD and, recently, more avant-garde methods involving artificial intelligence algorithms. Research has shown that CAD can spot subsolid lung nodules more accurately than human readers can, find lung cancer metastases, and identify cancers originally missed during CT lung cancer screening.
Yet even with the assistance of these techniques, radiologists still occasionally miss lung cancers and incorrectly label benign nodules as potentially malignant during screening, according to the authors. One of the chief causes of overlooking or misidentifying small lung lesions is the presence of extraneous vessels nearby.
Addressing this specific obstacle, Lo and colleagues developed a function for CAD software (ClearRead CT, Riverain Technologies) that subtracts vessels from chest CT scans as it searches for lung nodules. The software received 510(k) clearance from the U.S. Food and Drug Administration (FDA) in 2016.
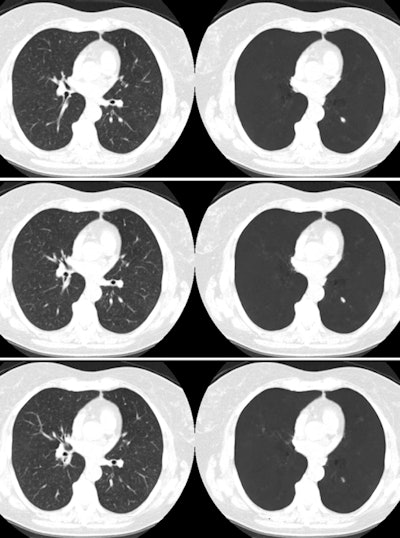
"Whereas CAD systems focus on the characteristics of the nodule to make a determination of a positive result, vessel subtraction removes vessel clutter and structural complexities surrounding the nodule, potentially decreasing the likelihood that a [false-positive] result will occur," they wrote.
To determine the benefit of using the vessel-suppression function in lung cancer screening, the group compared the lung cancer detection rates of 12 radiologists with and without the software for 324 chest CT exams. A panel of three experts in thoracic radiology randomly selected 273 cases from the National Lung Screening Trial (NLST) database, 45 from University Hospital Cleveland, and six from the University of Maryland Hospital. The final ratio of CT exams with no nodules detected to exams with any nodules detected was 2:1.
Among the 93 malignant nodules found after long-term follow-up, 53 were actionable nodules that required further investigation, and nearly 70% of those nodules were in contact with vessels.
Better detection, faster interpretation
Upon examining the results, the researchers found that the radiologists detected just shy of 90% of the malignant nodules and 82% of all nodules between 5 mm and 20 mm with the CAD software. Using CAD led to a statistically significant increase in the area under the curve for detecting lung cancer by 14% and the sensitivity by 15.5%.
Detecting lung cancer on CT with and without CAD | |||
Radiologist | Radiologist with CAD | p-value | |
Area under the curve | 63.3% | 77.3% | 0.000073 |
Sensitivity | 64.5% | 80% | 0.000025 |
Specificity | 89.9% | 84.4% | 0.0025 |
Interpretation time | 132.3 seconds | 98 seconds | < 0.01 |
In contrast to the increase in sensitivity, the specificity of detecting lung cancer decreased by 5.5% with CAD compared to without CAD. In addition, the average false-positive rate for malignant nodules was 17% higher with CAD than without it (39% versus 22%, p < 0.01), although this false-positive rate is still lower than published rates using other CAD software, the authors noted.
Implementing any CAD algorithm generally improves the detection of lung cancer by approximately 11% or 12%, Lo said. In this case, adding a vessel subtraction function to a CAD algorithm may have helped boost the improvement even more -- to the 15.5% increase in detection rate in this study.
Furthermore, using CAD resulted in a 34.3-second, or 26%, drop in interpretation time. This was the case even after excluding any outlier reading times from the data.
"This substantial savings in interpretation time may in part be caused by the addition of a vessel subtraction component, which may facilitate a more rapid interpretation by permitting nodules to stand out prominently in the absence of vessels," the authors wrote. "Thus, vessel suppression might allow a quicker survey [of CT scans] and may take even less time on average to interpret with [CAD] compared with an unaided approach."
Favorable reviews
In this dataset, many of the nodules were subtle, stage IA cancers and, thus, easily overlooked, Lo said. So even though there was a high detection rate with the CAD software, the radiologists ignored about 9.5% of the cancer cases, possibly due to the shape and image characteristics of the cancers.
"We hope that when radiologists gain more experience in the detection of lung nodules/cancer on low-dose CT and their vessel-suppressed counterparts with CAD, its [potential] can be further realized," he said.
The primary limitations of the study were its artificial testing conditions compared with a real-life clinical setting and the dip in specificity associated with the CAD software, according to the authors.
"[Recently], we have tested the software in routine clinical use, and it received favorable reviews from thoracic radiologists," said co-author Dr. Charles White, a professor of radiology and internal medicine at the University of Maryland School of Medicine. "We have been looking at further research projects, which will perhaps involve our lung cancer screening population when we have sufficient patients."