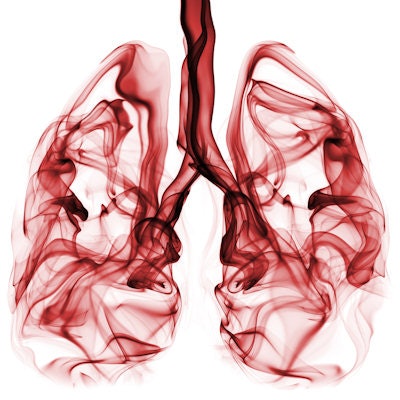
How might a lung cancer risk-prediction model improve follow-up CT screening? A model that incorporates patient characteristics and CT data could allow many individuals to skip the first annual follow-up screening exam -- reducing costs and radiation exposure, according to research published online recently in Thorax.
Dr. Anton Schreuder of Radboud University Medical Center.
There are numerous factors that determine the ideal time for a person to undergo follow-up screening for lung cancer, first author Dr. Anton Schreuder of Radboud University Medical Center in Nijmegen, the Netherlands, told AuntMinnie.com. The polynomial model sought to integrate the best combination of patient characteristics and CT scan variables.
"One rigid CT lung cancer screening follow-up protocol is not ideal; it will lead to either too much or insufficient diagnostic testing," he said. "By applying our model, the number of unnecessary scans can be greatly reduced, though at the expense of a higher relative proportion of delayed diagnoses."
Accounting for variation
Whereas analyses of various large-scale trials such as the NLST and the Continuous Observation of Smoking Subjects (COSMOS) have affirmed the value of CT lung cancer screening, discussions concerning appropriate follow-up screening intervals are ongoing, according to the authors. There is considerable variation in the progression of lung cancer in different people, and the prevailing one-size-fits-all strategy for follow-up screening may not be optimal for many individuals.
"In the case of CT lung cancer screening, participants with lung cancer benefit, but those who don't may come out more 'damaged' than when they began," Schreuder said. "Besides the potential adverse effects of additional diagnostic tests on the body, psychological stress should not be taken lightly. The question remains: How many people should we be allowed to keep awake at night at the expense of missing one early lung cancer diagnosis?"
Moving toward a more personalized approach for follow-up CT screening, Schreuder and colleagues developed their polynomial model to predict the individual risk of having a diagnosis of lung cancer between the first and second year after a negative baseline screen.
They developed the model by evaluating data from participants in the NLST who did not receive a diagnosis of lung cancer on an initial CT screening exam or a one-year follow-up exam. Almost 20 variables were associated with lung cancer risk, including specific patient characteristics as well as observations made on CT scans acquired during baseline screening.
Out of these variables, they selected the 11 most relevant and used polynomial transformation to model the nonlinear relationship between these variables and lung cancer. They further limited the model to second-power polynomials to minimize "overfitting," or oversimplification of the complex interaction among the variables.
Comparing risk models
Among the 24,542 individuals examined, 174 (0.7%) received a diagnosis of lung cancer between the first and second years of follow-up screening. The investigators compared the effectiveness of their polynomial model in identifying the cancer-free individuals relative to several other lung cancer risk-prediction models that have emerged in recent years:
- Patz model. Patz and colleagues determined that individuals who had a negative baseline screening result, i.e., no nodules greater than 4 mm, could skip the first annual follow-up screening round. A major advantage of this design is its simplicity, but the disadvantage is that it offers only one threshold for the number of delayed diagnoses, Schreuder said.
-
Patient characteristics model. The Bach, Spitz, Liverpool Lung Project, Etzel, Kovalchik, and Pittsburgh Predictor models, among others, are exclusively based on patient characteristics, including age, smoking pack-years, and prior diagnoses of related lung diseases.
The patient characteristics model serves as a reference point for the best achievable accuracy before the first scan is taken, Schreuder said. However, by leaving out information from CT scans, the model significantly increases the number of scans needed to diagnose (NND) cancer, ultimately delaying diagnosis.
- Diameter model. Nodule size is arguably the best individual predictor of lung cancer, but like the Patz model, the diameter model is likely to delay the diagnosis of multiple cases of cancer because it relies on a single variable, he said.
-
PanCan model. The Pan-Canadian Early Detection of Lung Cancer (PanCan) risk prediction model calculates risk based on a mixture of patient characteristics and CT scan data, but it requires that at least one nodule be present to make accurate predictions.
"The PanCan model is well-known, thoroughly validated, and one of the few which takes scan outcomes into consideration," he said. It is included in the British Thoracic Society guidelines for nodule management.
Lowering costs, radiation exposure
Out of the five types of models assessed, the polynomial model was the most accurate -- as determined by the area under the curve from receiver operating characteristic analysis -- for predicting which patients would not develop lung cancer between the first and second year after baseline screening.
Comparison of lung cancer risk models for follow-up CT screening | |||||
Measure | Patz model | Patient characteristics model | Diameter model | PanCan model | Polynomial model |
Area under the curve | 66.6% | 69.3% | 69.7% | 72.7% | 78.5% |
Delayed diagnoses | 71 | 0 | 73 | 0 | 0 |
CT scans avoided | 18,052 | 318 | 18,162 | 658 | 2,558 |
Sensitivity | 59.2% | 100% | 58% | 100% | 100% |
With the polynomial model, 2,558 patients (10.4%) could have skipped the one-year follow-up screening without a single delay in diagnosis -- nearly four times more than with the PanCan model and eight times more than with the patient characteristics model.
If a higher threshold for delayed diagnosis was used for the polynomial model, even more NLST participants could have avoided a one-year follow-up CT exam. However, that benefit would come at the risk of delaying cancer diagnosis in an increasing number of individuals. If 7,544 participants skipped the first round of follow-up screening, eight of them would have had a delayed diagnosis; 10,947 skipped exams would have led to 17 delayed diagnoses, 16,710 skipped exams would have led to 44 delayed diagnoses, and 20,023 skipped exams would have led to 70 delayed diagnoses.
Furthermore, implementing personalized follow-up screening intervals could reduce the cost of screening programs, participants' exposure to radiation, and radiologist workload, without delaying the diagnoses of too many cancers, the authors noted.
Limitations of this retrospective study included variation among the nodule detection rates of different radiologists in the NLST, only three screening time points (up to two annual screens following the baseline exam), and a lack of automated nodule volume measurements via computer-aided diagnosis (CADx) or artificial intelligence (AI) algorithms, according to the researchers.
Improving prediction models
"Lung cancer prediction is difficult; it would not surprise me if similar accuracy is measured by other models consisting of other variables and coefficients," Schreuder said. "Automatic scan segmentation using machine learning should pave the way toward improved prediction models."
Though the researchers have yet to clinically apply their polynomial model to follow-up screening, they hope to do so in the near future.
"It is expected that the advent of a national CT lung cancer screening program in the Netherlands (as well as in many other countries) is not too far away," he said. "It would be fantastic if our model were to be applied immediately, but that remains to be seen. If possible, external validation should be performed on other cohorts in the meantime."