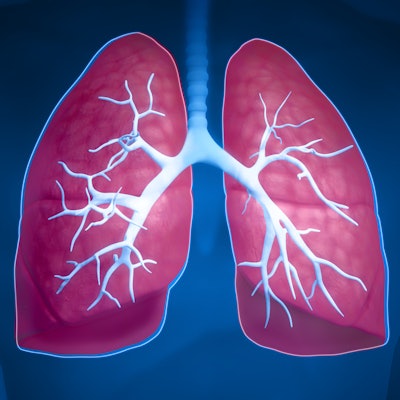
Researchers from the University of Pittsburgh developed a statistical model that combined data from CT scans with patient demographic information to predict an individual's risk of lung cancer on CT lung screening exams. The model worked better than several more-established methods, according to an article published online March 12 in Thorax.
In recent years, numerous groups have promoted the use of prediction models that take into account different combinations of risk-based criteria to determine an individual's cancer risk and, in turn, his or her need for a CT lung screening exam. Several studies have reported that such risk prediction models offer unique advantages compared to standard lung screening eligibility criteria, including reducing costs and increasing the efficiency of the test.
Building upon this earlier work, Dr. David Wilson and colleagues from the University of Pittsburgh developed a lung cancer prediction model -- the Lung Cancer Causal Model (LCCM) -- by applying a machine-learning algorithm to a set of variables that included patient demographics, features evident on CT scans, and clinical data such as smoking history.
The researchers acquired this information from the CT scans and patient histories of 218 high-risk lung cancer cases involved in the Pittsburgh Lung Screening Study (PLuSS). All participants met a specific set of criteria that included having quit smoking within the past 15 years, having a smoking history of at least 30 pack years, and being 55 to 77 years old.
Among all variables, the model identified one factor that was directly linked to an increased risk of lung cancer (the presence of blood vessels around lung nodules) and two factors that were inversely linked to lung cancer risk (the number of nodules a patient had and the length of time since the patient quit smoking). The three associations were statistically significant (p < 0.05).
Wilson and colleagues then trained the LCCM algorithm on a subset of cases and found that it predicted lung cancer more accurately than other models cited in the literature, including the Bach model and the Prostate, Lung, Colorectal, and Ovarian (PLCO) cancer model. The differences were statistically significant (p < 0.001) for all models except for the Brock full model, as based on area under the curve (AUC).
Accuracy of LCCM vs. other models for CT lung cancer screening | |||||
PLCO model | Bach model | Brock parsimonious model | Brock full model | LCCM | |
Area under the curve | 0.56 | 0.72 | 0.7 | 0.79* | 0.88 |
The LCCM algorithm's accuracy for lung cancer detection improved further after validation on a separate cohort -- achieving an AUC of 0.9, compared with 0.81 for the Brock parsimonious model.
What's more, the prediction model was able to correctly identify 28.3% of benign nodules for patients who had a lung cancer risk of at least 69% without missing a single cancer diagnosis.
This result could potentially eliminate the need for nearly a third of individuals with benign nodules to undergo additional testing -- helping to address concerns about the high false-positive rate associated with CT lung screening, which was 96.4% for the National Lung Screening Trial (NLST), Wilson noted in a statement from the university.
"While it has been known for some time that tumors recruit more vascular support, this is the first time that we've been able to use computer technology to quantify their contribution and incorporate them into a predictive model that decides, with certainty, that some patients don't have cancer," he said.