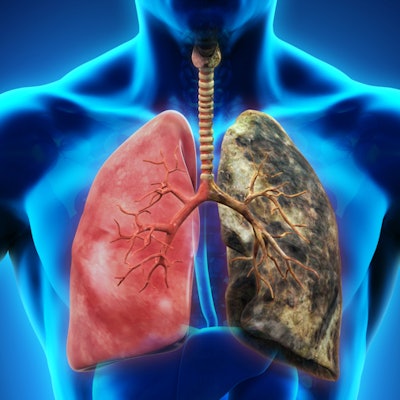
An artificial intelligence (AI) model developed by Google can assess cancer risk on CT lung cancer screening studies as well as, or even better than, experienced radiologists -- potentially enabling automated evaluation of these exams, according to research published online March 20 in Nature Medicine.
The deep-learning model identifies regions of interest and predicts the likelihood of cancer on low-dose computed tomography (LDCT) lung cancer screening exams. It performed comparably in testing to experienced radiologists when a prior CT exam could also be reviewed. When no prior CT study was available for comparison, the algorithm yielded fewer false positives and false negatives than the radiologists, according to the researchers from Google and Northwestern Medicine in Chicago.
"These models, if clinically validated, could aid clinicians in evaluating lung cancer screening exams," the authors wrote.
Shortcomings of Lung-RADS
Although the American College of Radiology's Lung-RADS guidelines have improved the consistency of lung cancer screening, Lung-RADS is still limited by persistent inter-grader variability and incomplete characterization of imaging findings, according to the team of researchers led by Diego Ardila and corresponding author Dr. Daniel Tse of Google.
"These limitations suggest opportunities for more sophisticated systems to improve performance and inter-reader consistency," the authors wrote. "Deep learning approaches offer the exciting potential to automate more complex image analysis, detect subtle holistic imaging findings, and unify methodologies for image evaluation."
The researchers said they sought to move beyond the limitations of prior computer-aided detection (CAD) and computer-aided diagnosis (CADx) software to develop a system that could both find suspicious lung nodules and assess their risk of malignancy from CT scans.
"More specifically, we were interested in replicating a more complete part of a radiologist's workflow, including full assessment of LDCT volume, focus on regions of concern, comparison to prior imaging when available, and calibration against biopsy-confirmed outcomes," the authors wrote.
End-to-end analysis
The Google system comprises three components:
- A 3D convolutional neural network (CNN) that was trained using LDCT volumes with pathology-confirmed cancer cases to perform end-to-end analysis of CT volumes
- A CNN region-of-interest model for detecting 3D cancer candidates in the CT volume, trained with help from additional bounding box labels
- A CNN cancer risk prediction model that was also trained using pathology-confirmed cancer labels
The CNN cancer risk prediction model utilizes outputs from the previous two models and can also incorporate regions from a patient's prior LDCT studies into its analysis, according to the researchers.
They trained and tested the deep-learning model on 42,290 CT cases from 14,851 patients in the National Lung Screening Trial (NLST). Of these patients, 578 developed biopsy-confirmed cancer within the one-year follow-up period, according to the researchers. Of the 14,851 patients, 70% were used for training and 15% were employed for tuning the model. The remaining 15% were utilized for testing. The model produced an area under the curve (AUC) of 0.94 for the test dataset of 6,716 NLST cases.
Next, the researchers performed a two-part retrospective reader study with the participation of six U.S. board-certified radiologists with an average of eight years of clinical experience. In 583 cases, the radiologists read a single full-resolution screening CT volume, with access to associated patient demographics and clinical history. The deep-learning model produced an AUC of 0.96 based on review of the images alone.
However, the six radiologists performed at a level trending at or below the model's AUC. Furthermore, the model produced a statistically significant improvement in sensitivity for all three cancer malignancy risk cut-off score thresholds and in specificity for two of the three thresholds.
Better sensitivity, specificity
The second part of the study added access to CT volumes from the previous year. The deep-learning model produced an AUC of 0.93 and generated 11% fewer false positives and 5% fewer false negatives than the radiologists.
"The system can categorize a lesion with more specificity," said co-author Dr. Mozziyar Etemadi of Northwestern University in a statement. "Not only can we better diagnose someone with cancer, we can also say if someone doesn't have cancer, potentially saving them from an invasive, costly, and risky lung biopsy."
The model also yielded an AUC of 0.96 on an additional independent screening dataset of 1,739 cases -- including 27 with cancer -- from an academic medical center in the U.S.
Performance of deep-learning model on screening dataset from U.S. academic medical center | ||||
NLST reader sensitivity (estimated by retrospectively applying LUNG-RADS 3 criteria) | Deep-learning model sensitivity | NLST reader specificity (estimated by retrospectively applying LUNG-RADS 3 criteria) | Deep-learning model specificity | |
Predicting cancer in one year | 77.9% | 83.7% | 90.1% | 95%* |
Predicting cancer in two years | 54.2% | 64.7%* | 90.1% | 95.2%* |
"[The deep-learning model's performance] creates an opportunity to optimize the screening process via computer assistance and automation," the authors wrote. "While the vast majority of patients remain unscreened, we show the potential for deep learning models to increase the accuracy, consistency, and adoption of lung cancer screening worldwide," the authors wrote.
The authors believe their model could assist radiologists during interpretation or be used as a second reader.
"We believe the general approach employed in our work, mainly outcomes-based training, full-volume techniques, and directly comparable clinical performance evaluation, may lay additional groundwork toward deep-learning medical applications," they wrote.
In a blog post, Google Technical Lead Shravya Shetty said that the company is in early conversations with partners around the world to continue additional validation research and deployment of the deep-learning model.