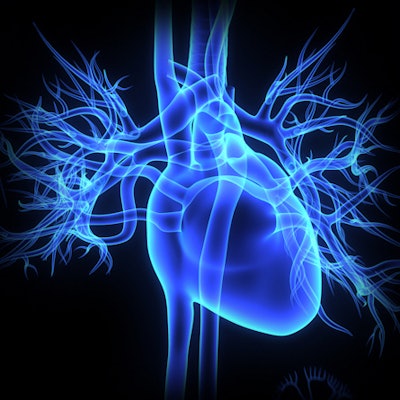
An artificial intelligence (AI) algorithm can predict heart attacks and death better on coronary CT angiography (CCTA) studies than risk-assessment methods such as the Coronary Artery Disease Reporting and Data System (CAD-RADS), according to research published online June 25 in Radiology.
In a retrospective study involving nearly 7,000 patients, a team of researchers led by Dr. Kevin Johnson from Yale University School of Medicine in New Haven, CT, found that machine learning yielded significantly higher accuracy than CAD-RADS for predicting all-cause mortality, coronary heart disease deaths, and myocardial infarctions. The algorithm also could increase the number of patients who benefit from early administration of statins, compared with the number of patients who will receive the treatment if CAD-RADS is used.
"Machine learning can improve the use of vessel features to discriminate between patients who will have an event and those who will not," the authors wrote.
CCTA is a promising tool for risk assessment of coronary artery disease (CAD) and has spurred the development of CAD-RADS for summarizing CCTA results. CAD-RADS, however, emphasizes stenoses -- a focus that may not consider key information about the arteries, said Johnson in a statement from the RSNA.
To take advantage of the additional data visible on CCTA, Johnson and colleagues developed a machine-learning algorithm that was trained to discriminate between patients with and without subsequent death or cardiovascular events by using 64 CCTA image features. Of the 6,892 patients who received CCTA between February 2004 and November 2009 and were included in the study, two-thirds were used for training and one-third was held for final testing of the model. The median follow-up on the patients was nine years.
The researchers collected data on risk factors from CCTA studies and scored arteries using CAD-RADS and four other risk-assessment methods. Using area under the curve (AUC), they then compared those results with prognostic scores derived from a machine-learning algorithm that analyzed the same risk-factor data.
Performance for predicting subsequent death or cardiovascular events from CCTA | ||
Prediction parameter | CAD-RADS | Machine-learning algorithm |
All-cause mortality | 0.77 | 0.72 |
Coronary heart disease deaths | 0.79 | 0.85 |
Coronary heart disease deaths or myocardial infarction | 0.80 | 0.85 |
The researchers also found that the machine-learning model would have a big impact on appropriate decision-making for initiating statin treatment.
"When deciding whether to start statins, if the choice is made to tolerate treating 45 patients to be sure to include one patient who will later die of coronary disease, the use of the machine-learning score ensures that 93% of patients with events will be administered the drug; if CAD-RADS is used, only 69% will be treated," the authors wrote.
In an accompanying editorial, Drs. U. Joseph Schoepf and Christian Tesche of the Medical University of South Carolina said the study results may have substantial clinical implications for data analysis and for CCTA data-derived risk stratification and outcome prediction.
"It appears that machine-learning methods may provide improved opportunity for individualized patient care compared with our conventional CT angiography scoring systems," they wrote.
They cautioned, however, that these machine-learning algorithms need to be validated in large multicenter evaluations and independent datasets before being placed into clinical use. The cost-effectiveness of these models also needs to be assessed.