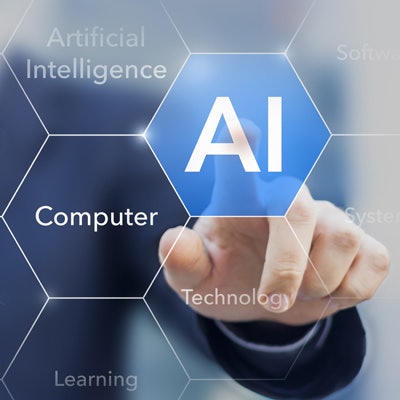
An artificial intelligence (AI) model was highly accurate for detecting urinary tract stones on unenhanced CT studies, enabling priority review of these cases by radiologists and referring physicians in a new study, published online July 24 in Radiology: Artificial Intelligence.
Researchers from Massachusetts General Hospital (MGH) developed deep-learning models based on a "cascade" of two convolutional neural networks (CNNs); the first CNN identified the sections of the CT scan that contained the urinary tract, while the second CNN then analyzed those sections for the presence of stones. In testing on CT studies acquired from scanners by two different vendors, the team's best-performing model yielded 95% accuracy for detecting urinary stones.
"As use of imaging continues to increase, artificial intelligence–assisted workflow may help triage and streamline patients," wrote the researchers led by Dr. Anushri Parakh and Hyunkwang Lee. "CNN models such as ours have the potential to aid in this task by identifying positive examinations to prioritize patient care."
The overall usage of emergency CT for patients with suspected urinary stones has doubled over the past decade. The growth in imaging volume has resulted in longer turnaround times, additional burden for radiologists, and longer hospital stays, according to the MGH researchers. They sought to investigate the accuracy of a cascading deep-learning system for detecting urinary stones on unenhanced images and also wanted to assess the effect of transfer learning to determine if the performance of pretrained models would be consistent across different types of scanners.
Their retrospective study included unenhanced abdominopelvic CT scans performed for suspected urolithiasis on 535 adult patients. Images were acquired on either a Discovery CT750HD (GE Healthcare) system using single-energy CT or a Somatom Definition Force (Siemens Healthineers) scanner with dual-source CT. The researchers utilized single-energy CT-equivalent blended image datasets from the Definition Force system.
Of the 535 adults, 279 had kidney stones and 256 did not. The researchers trained a variety of deep-learning models using different combinations of training data, as well as with and without pretrained CNNs, on 435 studies. They tested the models' performance on the remaining 100 scans, which included 50 exams from each type of CT scanner.
The best-performing model employed CNNs that had been pretrained with ImageNet and then fine-tuned using GrayNet, an in-house dataset of CT images that was created to pretrain models to recognize human anatomy.
Performance of deep-learning model for detecting urinary tract stones | |
Deep-learning model | |
Sensitivity | 94% |
Specificity | 96% |
Accuracy | 95% |
Area under the curve | 0.954 |
Two of the three false-negative results had small-sized stones, according to the researchers. They also noted the one false-positive examination had focal tumor-associated bladder wall thickening and calcification, which, despite being a "false" scan, would warrant radiologist review.
Based on their results, the team's "proposed model thus has the potential to accelerate triage in an urgent setting, allowing for rapid prioritization of examinations for review by radiologists and referring physicians," the authors wrote.