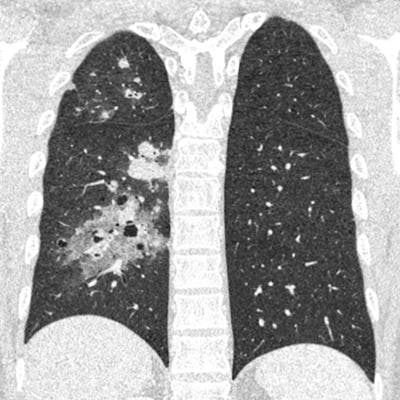
An ultrahigh-resolution CT scanner substantially improved the quality of imaging data acquired from patients with various chest diseases, compared with a standard CT scanner, in a new study, recently published online in the Journal of Computer Assisted Tomography. The advanced CT technology may enhance diagnostic imaging and disease characterization.
Prior preclinical research has demonstrated the capacity of the ultrahigh-resolution CT scanner (Aquilion Precision, Canon Medical Systems) to improve the image quality of coronary stents within imaging phantoms, effectively doubling the resolution of conventional CT scans without altering radiation dose.
The ultrahigh-resolution CT scanner offers a spatial resolution of 0.15 mm, compared with resolutions for standard CT scanners ranging from 0.23 mm to 0.35 mm. This improvement, in turn, allows for the use of a reconstruction matrix that, at 1024 x 1024 pixels, is twofold larger than the 512 x 512-pixel matrix for standard CT.
"Previous work has demonstrated the ability of the prototype scanner to achieve 0.15-mm resolution with high fidelity in high-contrast structures such as a coronary stent," senior author Dr. Marcus Chen from the U.S. National Institutes of Health (NIH) and colleagues wrote (J Comput Assist Tomogr, September 5, 2019). "However, it was unclear how this prototype scanner and higher reconstruction matrices would perform in the in vivo setting across a wide range of disease conditions."
For the current study, Chen's team set out to validate the prototype ultrahigh-resolution CT scanner in a clinical setting, which included the examination of metastatic cancers, lung nodules, and infections, among other diseases.
The researchers compared the quality of the chest imaging data of 101 patients who underwent ultrahigh-resolution and conventional CT scanning. Two physician readers independently rated the CT scans on a five-point scale ranging from excellent (1) to nondiagnostic (5) based on the European Guidelines on Quality Criteria for Computed Tomography. All the CT scans turned out to meet the standard for diagnostic image quality.
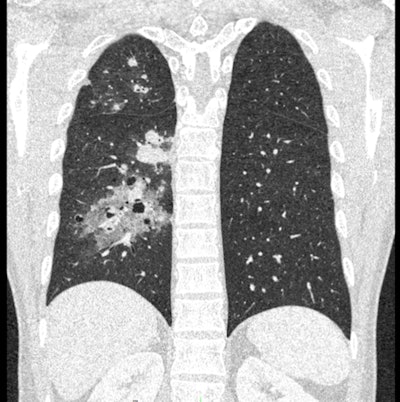
The group's comparative analysis showed that the ultrahigh-resolution CT scans offered statistically significant improvements in overall diagnostic confidence, as well as in the detection of details in small structures, lesion conspicuity, image sharpness, image contrast, and artifact presence.
However, the improved image quality of the ultrahigh-resolution CT scans came at the cost of significant increases in image noise for all tissue types.
Conventional CT vs. ultrahigh-resolution CT for chest imaging | ||
Conventional CT (512 matrix; 0.3-mm pixels) | Ultrahigh-resolution CT (1024 matrix; 0.15-mm pixels) | |
Diagnostic confidence | 1.18 | 1.09 |
Detection of details in small structures | 2.02 | 1.06 |
Lesion conspicuity | 2.02 | 1.08 |
Sharpness | 2.02 | 1.09 |
Contrast | 1.62 | 1.5 |
Presence of artifacts | 1.5 | 1.37 |
Noise | 1.75 | 1.98 |
Qualitative improvements to image quality for ultrahigh-resolution CT were exemplified by the more refined delineation of the limits of indistinct, fine, hazy structures such as ground-glass opacities, as well as the more accurate detection of tumor margins and the cystic components of diseased lung tissue.
"This enhancing visualization of heterogeneity within pathologic tissues may reasonably lead to improved tumor staging, classification, tissue characterization, and ultimately the use of these CT findings to measure and guide response to therapy," the authors wrote.
Furthermore, the improved image resolution could bolster training for new machine-learning or texture-analysis algorithms geared toward detecting a wide range of diseases, they noted.