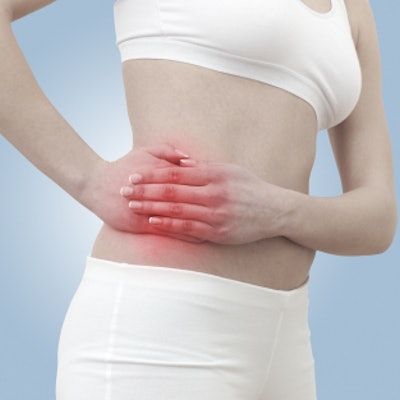
South Korean researchers developed an artificial intelligence (AI) algorithm that was highly accurate and generalizable for detecting acute appendicitis on CT exams in emergency room (ER) patients. The algorithm could, if necessary, potentially fill in for a radiologist, according to research published online June 12 in Scientific Reports.
A team of researchers led by Jin Joon Park and Dr. Kyung Ah Kim, PhD, of the Catholic University of Korea in Seoul developed a 3D convolutional neural network (CNN) for diagnosing appendicitis on abdominopelvic CT scans. Their algorithm yielded at least 90% accuracy in testing both during internal validation and in external validation on CT scans acquired at other institutions on systems from a different vendor.
"Acute appendicitis could be differentiated from a normal appendix without expert radiologists for patients with acute abdominal pain visiting the ER," the authors wrote.
A challenging diagnosis
Although CT has been found to be highly accurate for detecting acute appendicitis, the modality's performance relies on an accurate interpretation and an exam being performed using an appropriate CT protocol, according to the researchers. Due to a number of factors, such as variable positions of the appendix and diverse conditions, clinicians may find it challenging to diagnose a normal versus an inflamed appendix.
"However, radiologists are often not available during off-hours, for example, at night in ERs," the authors wrote. "An alternative method that could carry out the roles of radiologists on their days off, introduce efficiencies to the risk prediction of acute appendicitis, and provide decision support for clinical care of patients with abdominal pain in the ER would be very helpful."
The research group sought to apply deep learning to see if it could help. They trained and validated a CNN using abdominopelvic CT exams from 667 patients who visited their hospital's ER with acute abdominal pain between December 2018 and May 2019. These included 215 cases with acute appendicitis and 452 with a normal appendix. All studies were acquired on a Discovery CT 750 HD scanner (GE Healthcare).
Generalizable performance
Next, the team performed external validation using datasets from two other institutions. The first external validation set contained 60 CT image sets acquired on a Somatom Definition Edge scanner (Siemens Healthineers) and included 26 patients with acute appendicitis and 34 patients with a normal appendix. The second external test set of 40 cases included 20 patients with acute appendicitis and 20 with a normal appendix who were scanned on a Somatom Perspective scanner (Siemens).
AI performance for diagnosing appendicitis on internal and external test sets | |||
Internal validation using eight-fold cross validation for institution #1 (667 total cases) | External validation for institution #2 (60 cases) | External validation for institution #3 (40 cases) | |
Sensitivity | 90.2% | 88.5% | 95% |
Specificity | 92% | 91.2% | 100% |
Accuracy | 91.5% | 90% | 97.5% |
Area under the curve | 0.96 | 0.96 | 0.95 |
After reviewing the algorithm's "heat maps" for the cases it got wrong, the researchers found that most of the false-negative results were caused by misjudging a collapsed ileum containing small air as a normal appendix. False-positive results were due to secondary changes caused by inflammation or by the algorithm incorrectly identifying ileum with wall thickening or bowel dilatation as an inflamed appendix.
The authors noted that they trained and tested the network using 3D isotropic cubes (4 cm³) from the CT datasets that were manually extracted from the CT exam by radiologists.
"For practical applications, an automatic localization of the appendix region is necessary," they wrote. "Therefore, a future study is needed to develop an automatic localization algorithm of the appendix regions, along with a classification algorithm."