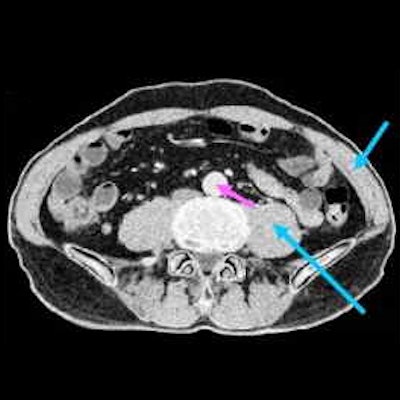
An artificial intelligence (AI) algorithm was able to extract more data from conventional CT images, allowing single-energy CT to approach the performance of dual-energy CT without the additional radiation, according to a study published October 19 in the journal Patterns.
The combination could translate to better patient care, noted first author Wenxiang Cong, PhD, of the Rensselaer Polytechnic Institute in Troy, NY, and colleagues. Cong and coauthor Ge Wang, PhD, collaborated with researchers from Shanghai First-Imaging Tech and GE Research.
"We hope that this technique will help extract more information from a regular single-spectrum x-ray CT scan, make it more quantitative, and improve diagnosis," Wang said in a statement released by the institute.
CT visualizes the shape of tissues in the body, but not their composition, even when contrast agents are used, the group noted. Dual-energy CT (dual-energy CT) can do this, but it requires higher doses of radiation and tends to be a more expensive exam.
"Conventional single-spectrum CT reconstructs a spectrally integrated attenuation image and reveals tissue morphology without any information about the elemental composition of the tissues," the team noted. "Dual-energy CT acquires two spectrally distinct datasets and reconstructs energy-selective (virtual monoenergetic) and material-selection (material decomposition) images. However, dual-energy CT increases system complexity and radiation dose compared with single-spectrum CT."
Wang and colleagues sought to apply a deep-learning algorithm to CT scans to produce images that would usually be generated by dual-energy CT. They used dual-energy CT images to train the algorithm.
"With traditional CT, you take a grayscale image, but with dual-energy CT you take an image with two colors," Wang said in the institute's statement. "With deep learning, we tried to use the standard machine to do the job of dual-energy CT imaging."
The model produced "high-quality approximations" of dual-energy CT exams on conventional CT with a relative error rate of less than 2%, the group found.
"[Our model] was trained on clinical dual-energy data, showing an excellent convergence behavior ... the [study] results showed excellent agreement between our [virtual monoenergetic] images and the two-spectrum DECT counterparts," the group wrote.
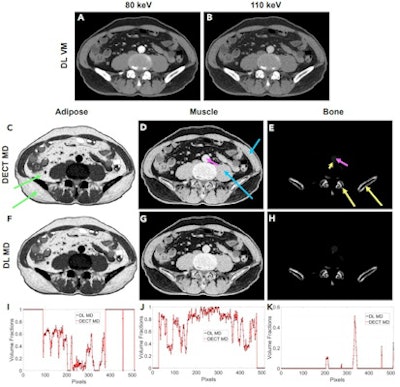
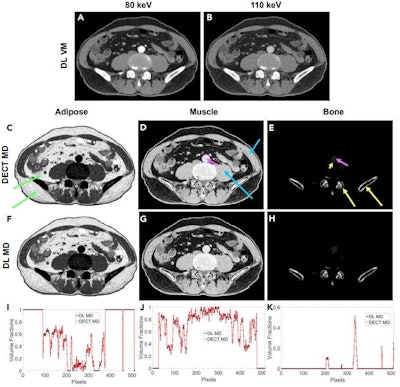
Multimaterial decomposition [MMD] into three basis materials (adipose, muscle, and bone). (A) and (B) deep-learning [DL] virtual monoenergetic [VM] reconstructions at 80 and 110 keV, respectively, as the input for MMD; (C), (D), and (E) MMD images from dual-energy CT [DECT] for adipose, muscle, and bone, respectively; (F), (G), and (H) MMD images from our DL approach for adipose, muscle, and bone, respectively; (I), (J), and (K) the profiles along the vertical midlines through the adipose, muscle and bone images, respectively, showing excellent agreement between the DECT and DL results. The adipose tissue is bright only in the adipose image (green arrows), the muscle and kidneys are bright only in the muscle image (blue arrows), and the bone is bright only in the bone image (yellow arrows). However, iodine in the contrast-enhanced abdominal aorta (pink arrows) causes this structure to appear in both the muscle image and (faintly) the bone image. Notably, the calcified plaque in the abdominal aorta (yellow arrowhead) appears in the bone image and is substantially brighter than the blood. Images and caption courtesy of Patterns.
The findings suggest that conventional CT could have more versatility, allowing it to be used for such applications as proton therapy planning as well as for photon-counting micro-CT for in vivo preclinical applications, according to Cong and colleagues.
"It has been clearly demonstrated in our study that a conventional CT energy-integrating dataset coupled with deep learning can deliver a close approximate of DECT," they concluded. "Thus, it is potentially feasible to just use conventional CT to perform some important tasks of DECT."