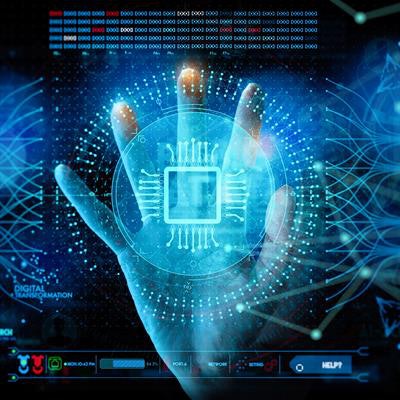
Delays in on-call radiology turnaround times can be predicted with a high level of accuracy by an artificial intelligence (AI) algorithm, according to research published online June 27 in Academic Radiology.
Researchers from the University of California, San Francisco (UCSF) led by first author Vaibhavi Shah and senior author Dr. Jae Ho Sohn used over 15,000 CT exams to train and evaluate a machine-learning algorithm for predicting turnaround time delays during nonbusiness hours. In testing, the model achieved an area under the curve (AUC) of 0.85 for predicting longer-than-average turnaround times for on-call radiology exams.
"This algorithm can be applied clinically when a physician is ordering the scan to reasonably predict delayed turnaround time," the authors wrote. "Such a model can be leveraged to identify factors associated with delays and emphasize areas for improvement to patient outcomes."
The researchers set out to create an ensemble machine-learning model to predict interpretation delays in on-call radiology cases, as well as to identify the issues that contributed most to delays. They hypothesized that factors such as inpatient status, study description, body part, time of the day, postgraduate level in radiology training, hospital campus, and written clinical history of the patient could be utilized to predict a delayed turnaround time.
Of the 15,117 CT reports from two different campuses included in the study, 12,849 (85%) were used for training and validation of the machine-learning algorithm and 2,268 (15%) were utilized for testing.
The authors first extracted the total time (the first radiologist communication time minus the clinician order time) and the interpretation time (the first radiologist communication time minus the scan completion time) from the RIS and free-text reports. The first communication time was defined as the earlier of either the preliminary report completion time -- an automatically generated variable -- or the verbal communication time as reported in the free-text report.
The average total time for the 15,117 CT exams was approximately 245 minutes, while the average interpretation time was 56.6 minutes.
In testing, the algorithm yielded the following performance:
- Prediction of total time more than 245 minutes: AUC = 0.85
- Prediction of interpretation time more than 57 minutes: AUC = 0.71
The most important factors used by the model were study description, time of day, and the on-call radiologist's postgraduate year, according to the researchers. They noted that the algorithm can be an important measure of past quality in the radiology workflow, enabling an opportunity to reveal areas that may benefit from a formal improvement process.
However, clinicians could also benefit from an estimate on whether or not a radiology report would be delayed based on the institution's past data, the group said.
"This can allow the physician to gauge when to expect results and handle care in the intermittent time," the authors wrote. "Clinicians can prepare for the delay by better triaging patients and seeking alternative diagnostic approaches if necessary."