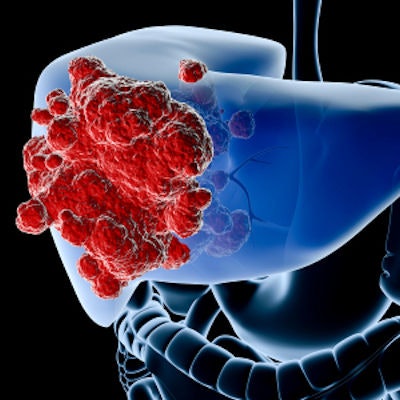
An artificial intelligence (AI) algorithm was able to identify hepatocellular carcinoma (HCC) on liver CT exams with a level of accuracy comparable to radiologists, according to research published August 7 in the British Journal of Cancer.
A team of researchers from China trained a deep-learning model to detect HCC on contrast-enhanced liver CT using 7,512 patients. In testing on both internal and external datasets, the algorithm -- called HCCNet -- yielded statistically comparable accuracy to three experienced radiologists.
What's more, the AI algorithm helped improve the performance of the radiologists.
"The developed deep-learning AI system can be a valuable tool for HCC diagnosis and clinical decision-making in high-risk patients," wrote the researchers, led by co-first authors Meiyun Wang and Fangfang Fu of Henan Provincial People's Hospital, and Bingjie Zheng and Yan Bai of Henan Provincial Cancer Hospital in Zhengzhou, China.
HCCNet was trained using 7,512 patients (including 647 with HCC) from Henan Provincial People's Hospital and then assessed on a separate internal test set of 385 patients. It was then further tested on an external test set of 556 patients (264 with HCC) from Henan Provincial Cancer Hospital.
A weighted mean of predicted probabilities of all images from each patient was used by the model to calculate an overall HCC risk score for the patient.
The researchers then compared the performance of the AI algorithm with three radiologists of eight to 10 years of experience for a subset of 95 patients from the internal test set and 82 cases from the external test set. The radiologists reviewed all CT images and interpreted them according to the LI-RADS guidelines.
A year later, the radiologists were asked to interpret the studies again, but with the help of AI.
HCCNet's performance for identifying HCC on subset of test cases | |||
Radiologists | AI | Radiologists + AI | |
Area under the curve | 0.869 | 0.883 | n/a |
Accuracy | 81.8% | 81.3% | 85.4% |
The difference in the AI algorithm's performance and initial results from the radiologists were not statistically significant. However, the improvement in accuracy after help with the AI accuracy was statistically significant (p = 0.017).
In other findings, the AI model's risk scores were significantly higher for patients with multiple tumors than for those with a single tumor (0.1971 vs. 0.1375, p = 0.006) and for those with severe fibrosis or cirrhosis compared with patients with none to moderate fibrosis (p < 0.001).
After radiologists reviewed the saliency heat maps for the algorithm's findings, the researchers concluded that the maps had an accuracy of 92.1%. Notably, HCCNet performed equally well in detecting small (≤ 5 cm) tumors as in larger tumors, according to the authors.
"To some extent, it could reduce the probability of unnecessary biopsy for smaller neoplasm masses," the authors wrote. "Further clinical trials are required to validate the aforementioned HCCNet diagnostic advantage."
The authors emphasized, however, that HCCNet can't replace radiologists in diagnosing HCC.
"It only uses CT images for analysis but does not take into account other auxiliary diagnostic parameters, such as viral hepatitis, liver cirrhosis, long-term alcohol intake, and aflatoxin," they wrote. "[A] future deep-learning model taking into account radiological image data, laboratory reports, medical history, and pathological graphics will potentially increase the performance of artificial intelligence diagnosis."