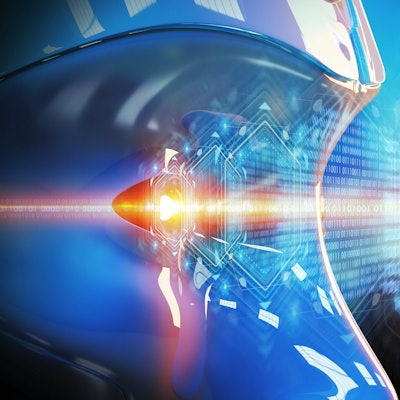
Artificial intelligence (AI) algorithms can perform comparably to radiologists in detecting lung cancer on low-dose CT lung cancer screening exams, according to research published online October 27 in Radiology: Artificial Intelligence.
A multinational team of researchers led by Colin Jacobs, PhD, of Radboud University Medical Center in Nijmegen, the Netherlands compared the performance of three high-performing deep-learning models to that of 11 radiologists on 300 cases. Although the mean radiologist performance was higher than all three algorithms, the difference was only statistically significant for one of them.
"These results offer several opportunities to optimize the reading of screening CT scans in lung cancer screening," the authors wrote.
Seeking to determine if algorithms that performed well in a public AI competition could yield similar results to radiologists, the researchers selected the top three algorithms from the Data Science Bowl 2017. That contest challenged developers to train software that could accurately determine when lung lesions were cancerous, according to the researchers.
The three best models from that challenge -- grt123, Julien de Wit and Daniel Hammack (JWDH), and Aidence -- were applied to an enriched data set of 300 exams, half of which were from the competition dataset and half of which were from the Pan-Canadian Lung Screening Trial. Of the 300 cases, 100 included cancer. All studies were also interpreted by 11 radiologists with varying levels of experience.
Performance of AI and radiologists for CT lung cancer screening | ||||
grt123 AI algorithm | Aidence AI algorithm | JWDH algorithm | 11 radiologists | |
Area under the curve (AUC) | 0.876 | 0.881 | 0.883 | Average = 0.917 (range, 0.841 to 0.944) |
Of the three algorithms, only the grt123 algorithm had an AUC that was significantly lower than the radiologists (p = 0.02). The differences for the Aidence and JWDH algorithms with the radiologists did not reach statistical significance (p = 0.26 and 0.29, respectively).
As for the potential clinical utility of these models, the authors pointed out that each of the algorithms produced a score between 0 and 1 that indicates its estimated likelihood that the participant will be diagnosed with lung cancer within a year. These models didn't, however, provide the location of the possible cancer or an explanation of how it arrived at that score.
"Potentially, direct estimation of the malignancy risk may be an effective way to optimize current guidelines in the future," the authors wrote.
As an alternative, these types of AI algorithms could potentially be utilized to triage normal studies, with only the possibly abnormal scans being reviewed by the radiologist. Although it hasn't been investigated yet, this triage strategy could substantially improve the cost-effectiveness of screening, according to the researchers.
"If future validation studies show that this approach is feasible, policy changes will be needed because at present, every screening CT scan must be categorized according to Lung-RADS by a board-certified radiologist in the United States to qualify for reimbursement," the authors pointed out.
The researchers recommended that future development of the models should focus on providing users with more information, such as the location of the suspicious pulmonary nodule.
"Subsequently, studies are needed which focus on evaluating how these algorithms can be integrated with the radiologists to positively change the follow-up recommendations in a screening program," they wrote.
For use in clinical practice, the algorithms' predictions will need to be calibrated, and the optimal cut-off points for decision making should be investigated in future studies, according to the authors.