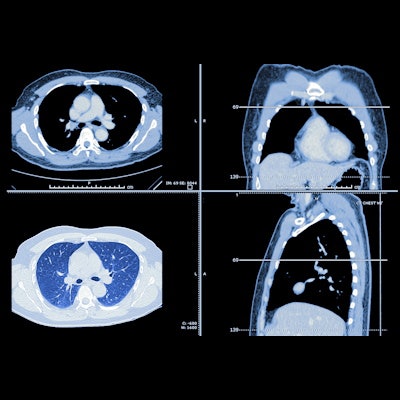
Investigators from the University of Chicago have developed a deep learning algorithm that identifies emphysema on CT lung cancer screening, according to research shared at the recent RSNA meeting.
The study results suggest that regular CT lung cancer screening could also catch other diseases, especially since the eligibility age range for lung screening was expanded this year, both by the U.S. Preventive Services Task Force (USPSTF) and by the U.S. Centers for Medicare and Medicaid Services (CMS), a team led by PhD candidate Jordan Fuhrman wrote in the study abstract.
"With the rapidly increasing global use of CT for lung cancer screening, automatic detection and characterization of other diseases, such as emphysema, within the scan range is critical and achievable through the use of deep learning," the group noted.
The study included 868 lung cancer screening CT exams that were part of the International-Early Lung Cancer Action Program (I-ELCAP). A radiologist reader assessed each scan for emphysema and rated disease severity based on the extent and presentation of the following features:
- Bronchial wall thickening
- Ground-glass opacities
- Micronodules
- Reticular/linear opacities
- Bronchiectasis
- Atelectasis
- Architectural distortions
The reader's findings were used to train the deep-learning algorithm, which evaluates CT scans and sections; features were extracted from CT sections using pre-trained VGG19 convolutional architecture, amalgamated using an attention-based weight factor, and merged with a classifier to identify emphysema on the scan. Fuhrman's group evaluated the performance of the deep-learning algorithm using area under the receiver operating characteristic curve (AUC).
"[Our algorithm consists] of a multiple instance learning machine learning scheme under which the data are organized into bags which have some known ground truth ... each of which are composed of one or more instances of unknown ground truth," Fuhrman told AuntMinnie.com via email. "The key assumption of multiple instance learning is that by investigating the instances, one can determine the bag class information. In my mind, this is analogous to how a radiologist reads a CT scan: They evaluate the CT slices individually and make a clinical decision for the entire CT scan based on the slice evaluation."
Fuhrman's group found that the deep-learning algorithm had an AUC for diagnosing emphysema on lung cancer screening scans of 0.93 -- performance comparable to or better than values published in previous studies, he said.
"There are a large number of nonlung cancer diseases that can be visualized on these scans, however, it could be time-consuming and expensive to manually detect and diagnose each one," Fuhrman told AuntMinnie.com. "Thus, objective, automatic models like the one produced in our study could be utilized for earlier detection and quantification of incidental findings, thus providing a potential prevention of progression or more rapid treatment of symptoms."