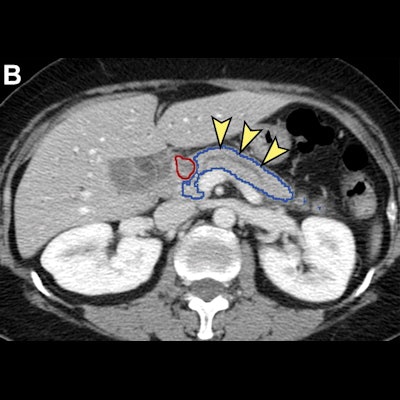
Computer-aided detection (CAD) software based on artificial intelligence (AI) technology can yield strong and generalizable performance for spotting pancreatic cancer on CT exams, according to research published online September 13 in Radiology.
A team of researchers led by Dr. Po-Ting Chen and Tinghui Wu of National Taiwan University in Taipei developed and tested a deep-learning algorithm for pancreatic cancer detection on contrast-enhanced CT. In an external dataset of nearly 1,500 cases, the model achieved an area under the curve (AUC) of 0.95.
"The CAD tool may be a useful supplement for radiologists to enhance detection of [pancreatic cancer]," the authors wrote.
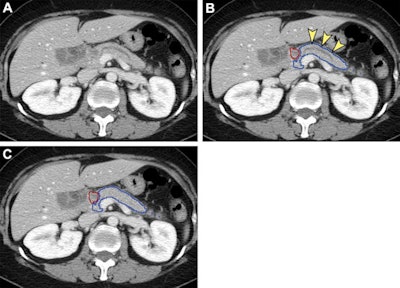
Early detection is the best way to improve the dismal outlook for pancreatic cancer, which has the lowest five-year survival rate among cancers and is projected to become the second leading cause of cancer death in the U.S. by 2030, according to the researchers. CT is the key imaging method for detecting pancreatic cancer, but it misses about 40% of tumors under 2 cm -- the size threshold at which the patient's prognosis worsens significantly.
To see if AI could help, the researchers developed an end-to-end, deep learning-based tool that comprises a convolutional neural network (CNN) for segmenting the pancreas on CT and then an ensemble of five CNNs to detect pancreatic cancer. After producing 89.9% sensitivity and 95.9% specificity on an internal test set of 256 patients, the model was then applied to an external test set of 1,473 CT studies gathered from institutions throughout Taiwan.
Performance of AI-based CAD on external test for detecting pancreatic cancer | |
Sensitivity | 89.7% |
Specificity | 92.8% |
Accuracy | 91.4% |
AUC | 0.95 |
What's more, the algorithm produced 75% sensitivity for detecting pancreatic cancers less than 2 cm in size. And it also yielded comparable sensitivity to radiologists from a tertiary referral center, according to Weichung Wang, PhD, co-senior author of the study and director of the MeDa Lab at National Taiwan University.
Interestingly, the algorithm also correctly identified pancreatic cancer in two cases by analyzing only the nontumorous portion of the pancreas.
"Our results also suggest that the classification convolutional neural networks might have learned the secondary signs of [pancreatic cancer], which warrants further investigation," the authors wrote.
The tool can also speed up radiologist interpretations by highlighting the suspicious region of interest, Wang said in a statement from the RSNA.
The researchers are now planning further assessments of the algorithm.
"While the results of this study provide strong support for the generalizability of the CAD tool in the Taiwanese and perhaps Asian populations, the performance of the CAD tool in other populations needs to be evaluated further," the authors wrote.
They also plan to study the model's performance in real-world clinical settings.
In an accompanying editorial, Dr. Alex Aisen and Pedro Rodrigues, PhD, of Philips Healthcare noted that many believe that AI may soon begin to live up to its hype and gain widespread adoption in augmenting, but not replacing radiologists. For example, a spectrum of AI algorithms designed to detect a large variety of abnormalities could be run concurrently to overread cases and reduce the number of missed findings (Philips was not involved in the study).
"We are not there yet, but we are heading in the right direction," Aisen and Rodrigues wrote. "Algorithms such as the one described here are helping to lead the way."