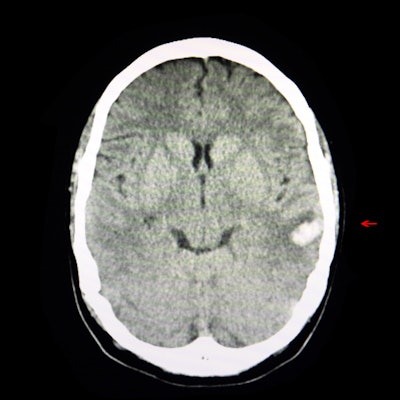
A deep-learning CT model can detect head abnormalities in 17 different regions, suggest research findings published August 21 in Cell Reports Medicine.
Researchers led by Aohan Liu from Tsinghua University found that their model achieved high accuracy marks for detecting four abnormality types in 17 anatomical regions and localizing abnormalities at the voxel level.
"We expect this work and future studies [will] benefit radiologists in the diagnosing and reporting of head CT exams and other medical imaging modalities," Liu and colleagues wrote.
Previous research efforts have shown the potential of deep-learning models for improving cancer detection by radiologists while also streamlining clinical workflows. For head CT, the researchers suggested that applying such a model would increase efficiency and accessibility, helping radiologists make faster and more accurate diagnoses.
Liu and colleagues developed and tested a deep-learning head CT model trained on 28,472 CT scans that consisted of a cross-modality deep learning framework for automatic annotation.
"Cross-deep learning...[uses] a dynamic multi-instance learning approach, enabling the model to not only detect the existence of different types of abnormalities but also to localize them at the voxel level in 3D CT scans," they wrote.
The researchers found that their model had a high average area under the receiver operating characteristic curve (AUROC) of 0.956 in detecting four abnormality types in 17 anatomical regions while also localizing abnormalities. The abnormality types included the following: intraparenchymal hyperdensity, extraparenchymal hyperdensity, intraparenchymal hypodensity, and extraparenchymal hypodensity. They also tested the model in a classification experiment for intracranial hemorrhaging, finding that it achieved an AUROC of 0.928.
Liu and colleagues suggested that such accurate predictions could help prioritize scans with various diseases.
The study authors highlighted three advantages of their model, including the following:
- Using free-text reports allows for automatic and fine-grained annotation for large-scale datasets to build deep-learning models practically and cost-effectively.
- Detecting abnormalities is generalizable to various diseases, meaning the model can be applied more broadly.
- The system can give accurate spatial locations of abnormalities to improve system interpretability and support further expert diagnoses.
"We hold that innovating methods to learn from readily available data sources like imaging reports is important, as it would not only reduce the cost of system development but also enable the utility of more data to help improve system accuracy and generalizability," they concluded.
The full study and model description can be found here.