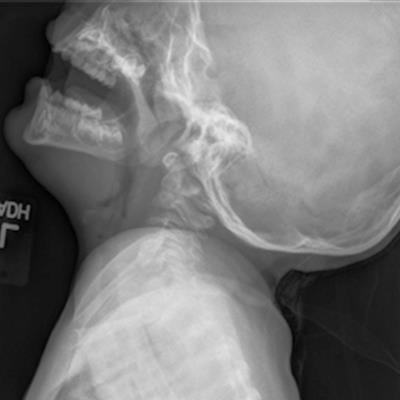
Artificial intelligence (AI) in the form of deep-learning classifiers can help distinguish between poor- and adequate-quality pediatric lateral airway x-rays acquired in the emergency department, according to a study published September 30 in Radiology: Artificial Intelligence.
The findings could improve patient care by reducing the need for repeat x-ray exams, wrote a team led by Elanchezhian Somasundaram, PhD, of Cincinnati Children's Hospital Medical Center in Ohio.
"Automated approaches to real-time detection of inadequate images have the potential to facilitate efficient workflow and standardize image quality by removing human bias, ultimately improving the quality of care for patients," the group wrote.
Lateral neck x-rays help clinicians evaluate the airway and soft tissues of children presenting with difficulty breathing, stridor, airway infection, obstructive sleep apnea, or suspected foreign body blockage. But the exam's effectiveness depends on correct positioning -- which can be difficult to accomplish in the pediatric population, the authors wrote.
"In children, it can be challenging to obtain optimal images, and at times, repeat imaging may need to be performed," they noted. "The need for repeat imaging may be subjective and can be driven by radiologists, radiography technologists, or the ordering provider. Ideally, a decision regarding the need for repeat imaging should be made at the point of care to avoid reporting on suboptimal examinations and the need for patient callback(s)."
Somasundaram and colleagues investigated whether a deep-learning algorithm could distinguish between adequate and inadequate lateral airway x-rays by conducting a study that included 1,200 exams acquired in the emergency department between January 2000 and July 2019. Two radiologists categorized the adequacy of the x-rays; any interpretation differences were resolved by a third radiologist. These categorizations were then considered "ground truth."
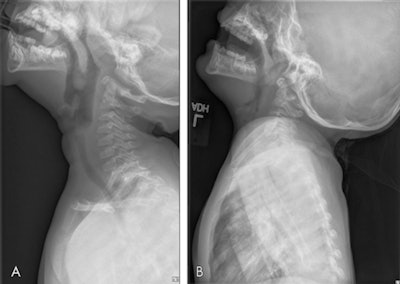
Of the 1,200 x-rays, 961 were used to train the deep-learning algorithm. Three technologists and three radiologists classified the adequacy of a deep-learning test dataset of 239 images, and their performance was compared with the algorithm's.
Overall, the algorithm performed comparably to or better than the radiologist readers and outperformed the technologist readers.
Deep-learning algorithm vs. human readers for assessing pediatric lateral airway x-ray images | |||
Performance measure | Technologist reader | Radiologist reader | Deep-learning algorithm |
Sensitivity | 70% | 95% | 90% |
Specificity | 78% | 64% | 82% |
Area under the curve | 0.74 | 0.80 | 0.86 |
Interreader/algorithm agreement with ground truth (Cohen's kappa) | 0.49 | 0.66 | 0.76 |
Interreader/algorithm agreement with ground truth (Fleisss kappa) | 0.36 | 0.59 | 0.80 |
"Our results suggest that the deep-learning classifiers developed in this work perform similarly to a group of board-certified pediatric radiologists as indicated by consensus agreement with ground truth ... and by [area under the curve] analysis ... with no significant difference observed," the group wrote. "However, when compared with the performance of the group of radiography technologists ... [the deep-learning classifier] outperformed significantly."
Using deep learning in this way shows promise for improving patient care, according to the authors.
"Such an algorithm has the potential to standardize image quality while also hastening patient output," they concluded.