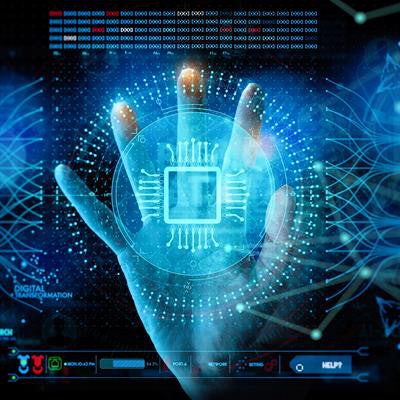
Missed appointments can impact patient care and result in a significant revenue shortfall for radiology departments. However, a machine-learning algorithm could help prevent some of these no-shows, according to researchers from the University of Maryland.
After training a machine-learning algorithm using data from over 4 million scheduled outpatient CT and MRI appointments at their medical system, a team of researchers led by Dr. Steven Rothenberg found that the algorithm yielded promising results when applied prospectively for predicting a patient's risk for missing their radiology appointment.
"These data create an opportunity to prioritize interventions to reduce missed appointments," said Rothenberg, who presented the findings at the recent Society for Imaging Informatics in Medicine (SIIM) annual meeting.
Patients are considered to be a no-shows if they either miss their scheduled appointment or arrive too late to utilize medical resources, according to Rothenberg. These missed appointments waste provider time, underutilize limited medical resources, disrupt scheduler workflows, and cause a loss of revenue for the radiology departments, he said.
"And most importantly, they deprive patients of important medical tests that their physicians ordered," he said.
These no-shows do not include cancellations, appointment modifications, or other situations when prior notice was provided, Rothenberg noted.
The researchers hypothesized that a machine-learning model could accurately predict which patients wouldn't show up, therefore creating opportunities to intervene and ensure that the patients receive the medical resources they need, he said. They trained several different types of algorithms using 39 variables or features from over 4.5 million non-same-day scheduled CT and MRI appointments at their 13-site medical system between January 1, 2017, and January 1, 2020.
After performing cross-validation, the best-performing model achieved an area under the curve (AUC) of 0.93 for predicting missed appointments. The team then applied this model prospectively at a single outpatient imaging center to review upcoming appointments over a six-week period.
The algorithm applied a "no-show" risk score within two weeks prior to every outpatient's scheduled appointment. The group then determined the outcome for all visits by reviewing the electronic medical record (EMR) software after the patient's scheduled visit.
Of the 2,264 scheduled radiology appointments, the researchers found that 123 (6%) were no-shows. The algorithm produced an AUC of 0.73 for predicting a missed appointment, a statistically significant result (p < 0.0005).
Although its performance was lower than on the training data, the algorithm still produced actionable information, Rothenberg said. The patients in the highest-risk category were seven times more likely not to show up for their examinations than those in the lowest risk category and three times more likely than the average patient to miss their appointment.
Based on these risk scores, radiology apartments could consider potential interventions such as text confirmations, providing ride-sharing services, double-booking appointments, offering babysitting services, and ensuring the availability of a translator, Rothenberg said.
He acknowledged the limitations of their model, including its reliance on prepandemic training data. In addition, the group's approach relies on technologists or support staff for appropriate documentation in the EMR, he said.
To improve the model, Rothenberg and colleagues are considering retraining the algorithm on postpandemic data in hopes of improving accuracy. They are also mulling over incentives for correct data entry, as well as adding other features to the model such as traffic, weather, and sports events to the model.
On a cautionary note, Rothenberg noted that there is potential for bias in the model that could result in a decrease in quality of service for patients based on race, zip code, and gender -- especially if double-booking of appointments are utilized as an intervention.