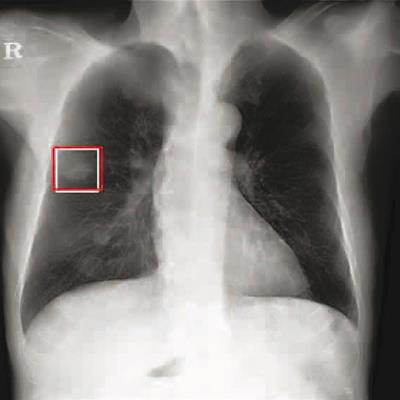
A group in Korea has developed an artificial intelligence (AI) model that could help radiologists detect pulmonary nodules on digital chest x-rays, according to a study published January 31 in JAMA Open Network.
A team led by Hwiyoung Kim, PhD, of Yonsei University College of Medicine in Seoul added a bone-suppression algorithm to a deep-learning model they previously developed in 2019. In a reader study, the model helped radiologists reach sensitivities of 92% for detecting lung nodules, the group found.
"The DLBS [deep-learning-based bone suppression] model could be beneficial to radiologists in the detection of lung nodules in chest radiographs without need of the specialized equipment or increase of radiation dose," the team wrote.
Dual-energy chest radiography is a technique that improves diagnostic accuracy in chest imaging by separating soft tissue from bones during a single x-ray exposure and thus improving the visualization of overlying and underlying anatomy. Yet the technique requires increasing radiation exposure to patients as well as specialized equipment, according to the authors.
In previous work, the group developed a deep-learning model that identifies high-quality bone- and soft tissue-only images using standard equipment. In this study, the researchers aimed to improve the model further by adding a bone-suppression algorithm and then tested its use by radiologists.
Kim and colleagues used data from x-rays taken from 1,449 patients with primary lung cancer, metastases, or benign tumors used imaging between November 2015 and July 2019. The x-rays were included regardless of the type of acquisition system (computed radiography or digital radiography) or the manufacturer of the radiography device.
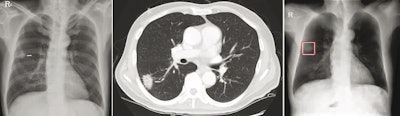
First, the investigators compared the performance of the DLBS model with the original model for detecting lung nodules using two external datasets of 246 and 205 patients. The bone-suppression model performed better, achieving a sensitivity of 91.5% for identifying lung nodules compared with 79.8% for the original model.
Next, for the reader study, the researchers used datasets of 244 normal and 207 lung nodule x-rays. Three thoracic radiologists reviewed all images to discriminate normal from nodule chest x-rays with and without the aid of the DLBS model. They demonstrated improved sensitivity of nodule detection when assisted by DLBS algorithm, at 92.1% using the DLBS model compared with 77.5% without it.
"The main finding was that our bone-suppressed model could more accurately detect pulmonary nodules on chest radiographs compared with the original model," the group noted. "In addition, radiologists experienced improved nodule-detection performance when assisted by the DLBS model."
As this was a decision analytical modeling study, the authors conceded limitations, namely that a high ratio of abnormal to normal radiographs in data used in the study would differ from that seen in clinical practice, and that other lung diseases, such as pneumonia, interstitial lung disease, and pleural effusion, were not considered.
Nonetheless, the researchers say the results are promising and warrant further studies.
"Further research is warranted to determine the applicability of this synthetic bone-suppressed model in a prospective multi-institutional study," the authors concluded.