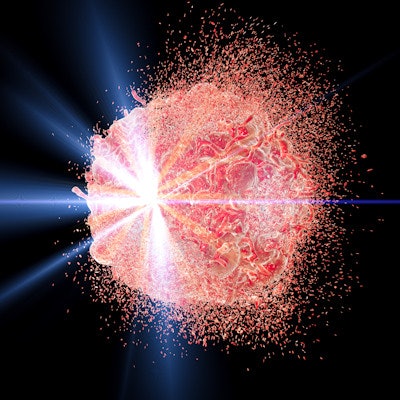
Automatically segmented contours generated on CT scans by a deep-learning algorithm can yield positive results when utilized clinically in radiotherapy planning, according to research published online June 8 in Radiation Oncology.
A team of researchers led by Dr. Jordan Wong from BC Cancer -- Vancouver evaluated the performance of deep learning-based autosegmentation models at two cancer centers for generating contours of organs at risk and clinical target volumes of patients receiving radiotherapy for three types of cancer treatments.
They found the AI-produced contours tended to need little editing and were well-received by radiation therapists/dosimetrists and radiation oncologists.
"Our results suggest that well-trained [deep learning-based auto-segmented contour] models were associated with a positive user experience and did not require any degree of manual editing that would appear to inhibit their usability," the authors wrote. "As this software continues to be utilized by our centers, scenarios associated with consistent [model] underperformance can be identified and targeted with additional training to further improve ... model accuracy."
Manual contouring of organs at risk and clinical target volumes can contribute significantly to treatment planning lead time, so autosegmentation options are often considered to alleviate these workload pressures, according to the researchers. Deep-learning-based methods are believed to provide better results than atlas-based techniques, but they aren't yet widely used in clinical practice, the researchers said.
"One possible factor associated with the slow adoption is the current lack of knowledge and guidelines regarding the commissioning and implementation of such machine learning applications," they wrote.
Following up on a prior study that found auto-segmented contours from a deep-learning algorithm to be closely similar to contours produced by multiple expert radiation oncologists, Wong and colleagues sought to assess the impact of these models in the clinical workflow at two cancer centers in British Columbia. Version 1.0.22 of the Limbus Contour auto-segmentation algorithm (Limbus AI) was used to prospectively and automatically generate contours from planning CT images for patients receiving radiotherapy for central nervous system, head and neck, or prostate cancer.
These contours for organs at risk and clinical target volumes were then imported along with the images into the treatment planning software. Next, the contours were reviewed manually and, if needed, edited prior to use in radiotherapy planning. Afterward, the researchers surveyed the radiation therapists/dosimetrists and radiation oncologists to rate the degree of edits required on the contours and their overall satisfaction. Edits were also assessed objectively.
Of the approximately 551 eligible cases that received contours by the autosegmentation algorithm between September 19, 2019, and March 6, 2020, 203 surveys were received for a response rate of 32%. The researchers noted that the majority of organ at risk contours required minimal edits, according to the surveys. In addition, the mean Dice similarity coefficient compared with edited treatment contours was ≥ 0.90 and the 95% Hausdorff distance was ≤ 2.0 mm.
The AI-developed contours also yielded the following mean satisfaction scores (1-5, 5 highest):
- Mean organ-at-risk contour satisfaction score in prostate structures: 4.6
- Mean organ-at-risk contour satisfaction score in head and neck cancer: 4.4
- Mean organ-at-risk contour satisfaction score for central nervous system cancer: 4.1
- Mean clinical target volume contour satisfaction score (encompassing prostate, seminal vesicles, and neck lymph node volumes): 4.1
Although contouring times couldn't be quantified before and after adoption of the AI autosegmentation software, both centers reported "noticeable time savings," according to the authors.
"Additional [organ-at-risk] and [clinical target volume] models, such as those applicable to breast, thoracic, and gynecological [radiotherapy] treatment planning, are currently being developed and tested in workflow by a variety of groups, including at our own institutions," they wrote.