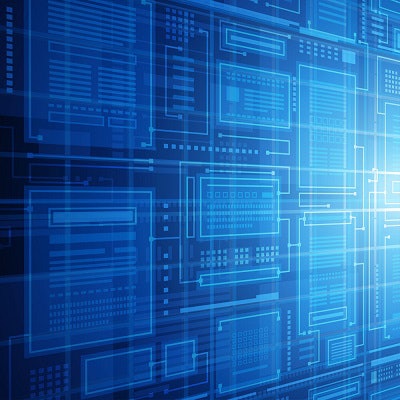
Will radiologists be replaced within 20 years by computers powered by artificial intelligence (AI)? Dr. Bradley Erickson, PhD, and Dr. Eliot Siegel debated this question on May 25 in a wide-ranging discussion at the U.S. National Cancer Institute (NCI) Shady Grove Campus in Rockville, MD.
Although Erickson from the Mayo Clinic in Rochester, MN, and Siegel from the University of Maryland School of Medicine differ on some aspects of how deep learning will affect radiology, both agree that computers will be able to handle many tasks currently performed by radiologists and will be able to provide quantitative imaging and biomarker measures on structured reports for review by radiologists.
Radiologists themselves will be harder to replace, contrary to the predictions of observers from outside medicine. Erickson and Siegel believe there will be many applications for AI that, instead of taking radiologists' jobs, will help radiologists provide better patient care.
"Whether or not we're talking about making radiology findings or replacing radiologists, there are all kinds of amazing opportunities for machine learning to revolutionize the way that we practice medicine and the way that radiology is practiced also," Siegel said.
Erickson and Siegel gave their presentation as part of the NCI's Center for Biomedical Informatics and Information Technology (CBIIT) Speaker Series, which features talks from innovators in the research and informatics communities.
Rapid development
Erickson predicted that within three years, and perhaps even sooner, deep-learning algorithms will be able to create reports for mammography and chest x-ray exams. That list will grow within 10 years to include CT of the head, chest, abdomen, and pelvis; MR of the head, knee, and shoulder; and ultrasound of the liver, thyroid, and carotids. Within 15 to 20 years, deep-learning algorithms will be able to produce reports for most diagnostic imaging studies, Erickson said.
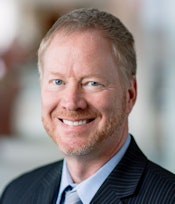
Computers will be able to reliably identify normal screening exams or special-purpose exams, and they will also create high-quality preliminary reports for most common exams, he said.
These reports "will have quantitative data and will follow a well-defined structure," Erickson said. "And then because it has that well-defined structure that [the algorithm] knows it's created, if the radiologist corrects it, [the algorithm] knows it has a learning example that it has to go back and figure out. And that's going to accelerate the learning phase even more."
Siegel agreed that computers will indeed be able to reliably identify normal screening or special-purpose exams, as well as provide high-quality preliminary reports.
"The key word is preliminary," Siegel said. "It will make some findings but certainly will not come anywhere close to replacing a radiologist."
Seeing the unseen
More important for patients, deep learning will likely "see" more than radiologists do today, Erickson said.
"It will produce quantitative imaging, which I think is a critical thing that we as radiologists fall short on," he said. "I think it will also mean that structured reporting will become routine, and that will accelerate the databases that are available."
With the power of deep learning, computers can see things humans can't or can't reliably detect such as genomic markers, according to Erickson.
"These are the sorts of things that computers can do that are quantitative and can add significant value to the management of patients," he said. "And this is where computers clearly are able to surpass humans."
Siegel also agreed that AI will lead to increased use of quantitative imaging and structured reports, but he noted that it's a far cry from replacing the radiologist. Instead, deep-learning algorithms will be tools for the radiologist. While computers can do things humans cannot, that argument works both ways; it also makes the case for computers working hand in hand with humans, Siegel said.
"Telescopes can see things that astronomers can't see, but that doesn't mean telescopes are replacing astronomers," he said. "They are tools for astronomers."
Focusing on the patient
Deep learning will also allow radiologists to focus on patients. It will improve access to medical record information and give radiologists more time to think about what's going on with patients, as well as perform invasive procedures, Erickson said.
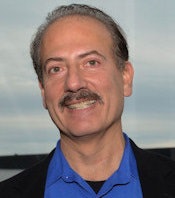
Those concerned that AI will lead to the demise of radiologists might consider the example of accountants, Erickson said. In 1978, accountants were worried that the introduction of the VisiCalc accounting software would take away their jobs. But that hasn't happened. VisiCalc changed what the accountant does, however.
"I think the same will happen with physicians," he said. "The computers will take the grunt work away and let us focus on the more cerebral things."
Erickson also referred to Siegel's challenge from a few years ago: that he would wash the car of the first software developer to create a program that finds the adrenal glands more consistently than an 8-year-old child taught to do so in less than 15 minutes. A team from the U.S. National Institutes of Health (NIH) recently was able to perform that task, producing Dice coefficients -- a statistical measure of similarity -- of around 65% to 80% as of November 2016.
While acknowledging the historically impressive achievement from Dr. Ronald Summers, PhD, and his team from the NIH, Siegel said the Dice coefficients suggested overlap with the correct answer was only around 65% to 68%. A fifth grader with 15 minutes of training would exceed that performance, Siegel said.
"And so if we can't even find the adrenals, then how are we going to essentially replace radiologists and do the things that need to be done?" he asked. "If I can't even find the adrenals, how can I identify an adrenal mass and determine how it enhances, etc. I can't even begin to do the job of the radiologist if I can't even find [the adrenals]."
Training issues
Having data to train these algorithms remains a key hurdle for the use of AI in medical imaging. Siegel noted that the National Lung Screening Trial cost hundreds of millions of dollars and took many years to complete. And that was just a lung nodule study, he said.
"How many databases would you to need to collect in order to demonstrate other chest pathology?" Siegel said. "The bottom line is that you will need millions of studies ... to even begin to be able to train the computer and to be able to do all of the annotations that are required. And how about other areas of the body and other diseases? There are upward of 20,000 diseases, and how are you going to train the system to do that?"
Virtually nobody thinks there is any chance of having general artificial intelligence that will enable machines to demonstrate an average human level of intelligence in 20 years, Siegel said.
"So in order to replace radiologists we need to have thousands of 'narrow' algorithms, each of which is going to require a validated database," he said. These databases "will need the annotations and the dollars to be able to support that. Is that going to happen in 100 years? Maybe. Is it going to happen within 20 years? Absolutely not."
Replacing the radiologist with a computer would also produce medicolegal issues, Siegel said.
"Who [would be sued] if the computer makes a mistake?" he asked. "Would it be the algorithm's authors, the physician who ordered the study, the hospital, the IT [department], the [U.S. Food and Drug Administration (FDA)], or everybody?"
Regulatory hurdles
Siegel noted that it took many years and extensive lobbying to get mammography computer-aided-detection (CAD) software cleared by the FDA as a second reader. The software doesn't do anything autonomously, and very few mammographers change their diagnosis because of CAD. Furthermore, there have been almost no second-reader applications -- much less a first-reader application -- approved in more than a decade, according to Siegel.
"Does anybody think that the FDA has the resources currently or a model to approve a few, much less dozens, much less hundreds or thousands of new applications for these algorithms that would be required [to replace radiologists]?" he said.
The "black box" nature of deep learning is also very much counter to the FDA's requirements for documentation of the development process, Siegel said.
"We don't even understand exactly how the computer is actually doing this," he said.
As a result, FDA reviewers and healthcare workers will feel extremely uncomfortable or hesitant to allow a system that cannot tell you how it works to do primary interpretation, according to Siegel.
Erickson believes the regulatory hurdles can be overcome, however.
"There's been a huge financial investment, and that will come with some of the political clout and resources that will be required," Erickson said. "There's also a strong interest in cutting the rate of healthcare cost increases, and the target is clearly on the back of us radiologists."
He also pointed out that the FDA has approved deep learning-based software. In January, imaging software developer Arterys received FDA clearance for its Arterys Cardio DL application, which uses deep learning. The FDA cleared the software based on its performance, Erickson said.
"The Arterys software was approved without having to show the mechanism or what the specific things were that the network was seeing, but rather it was showing the comparison with the human," he said.
As a result, the "black box" argument is not that big of a concern, he said. It's a myth that the FDA won't approve convolutional neural networks (CNNs) because it can't be understood how these deep-learning algorithms work.
"First of all, there are ways to figure out what the deep-learning algorithm sees," Erickson said. "But also, as long as you can show the robustness of the system to a wide variety of conditions, that's a manageable thing."
Understanding the algorithms
CNNs can be understood with several methods. For example, a presentation at the 2016 Scientific Conference on Machine Intelligence in Medical Imaging (C-MIMI) showed that one can "blank out" features of an image and measure a drop in performance by the algorithm. Also, researchers at Microsoft have been able to essentially convert the rules found by the algorithm into decision trees -- with only a slight loss in performance.
What's more, the U.S. is not the only market for deep learning; China and India desperately need these tools, Erickson said.
"They've been able to buy a bunch of CT and MR scanners, but they can't just go out and buy a radiologist," he said. "That's also really going to drive this market."
Siegel also pointed to some recent deep-learning adversarial examples, which are designed to provide inputs to the algorithm that cause it to make a classification error. For example, an image of a panda was originally classified by a machine-learning algorithm as a panda with 60% confidence; however, after a tiny, visually imperceptible change was made in the background of the picture, the model classified the image as a gibbon with 99% confidence.
"It just goes to show how delicate and how nonintuitive the way these systems work actually is," Siegel said. "We need to do a lot more research."
Erickson said, however, that this type of adversarial network is one way developers can see how well the models are generalizing and how robust they are.
"We actually use that sort of technology to create some of the augmented datasets to assure that we don't fall into those sorts of traps," he said. "I think that is a manageable problem."
It's also easy to create artificial examples where algorithms fail, just as it is easy to create artificial examples where humans fail, according to Erickson.
Appropriate expectations
Erickson said that the big challenge is to have appropriate expectations for deep learning in radiology. Algorithms for machine learning are rapidly improving, while the hardware for machine learning is really rapidly improving, he said.
"The amount of change in 20 years is unimaginable," Erickson said. "We need to be prepared, and we have to be careful about some of the evaluations that are done."
In the end, radiologists will be harder to replace than is commonly appreciated, and other professions are much more likely to be replaced first, Siegel said. Radiologists need to understand the value in their image data and metadata and be engaged in the effort to develop AI in radiology, according to Erickson.
"We are obligated to make sure that we take care of the patients in the best way we can," Erickson said. "That means we have to make sure that there's not improper interpretation of data, because that can lead to poor products and poor patient care. But I also think that noncooperation and saying that 'this will never happen, don't bother me' is counterproductive."