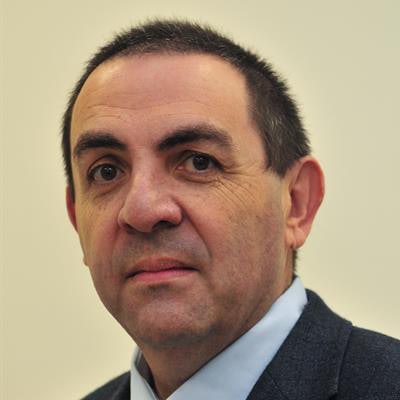
Medical imaging in Israel has a rich heritage and a bright future, and radiologists there are at the cutting edge of technological change and provide outstanding diagnostic and interventional services, Dr. Jacob Sosna, president of the Israel Radiological Association (ISRA), told RSNA 2017 delegates at Tuesday's special session.
About 200 of Israel's 450 radiologists are attending RSNA 2017. Although the population is 8.5 million, the country is relatively small at around 10,700 sq miles (27,800 sq km) or 10% the size of the U.K. The breakdown of the population is 76% Jewish, 20% Arab, and 4% other. Distinct minorities include immigrants, ultraorthodox Jews, and Bedouins, he said.
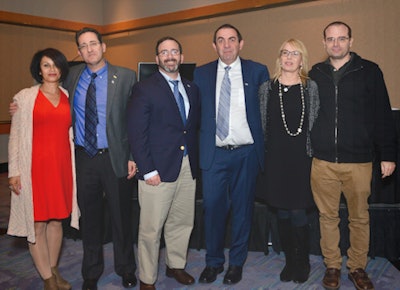
The country has five medical schools and between 500 and 700 Israelis graduate annually. Between 400 and 600 physician immigrants (international medical school graduates) are licensed annually.
Healthcare expenditures were around 7.3% of gross national product in 2014, compared with the average of 9.3% in Organization for Economic Cooperation and Development (OECD) countries. The healthcare indices are similar to those of Europe, but Israel has a higher birth rate, continued Sosna, who is a professor and chairman of radiology at the Hadassah Hebrew University Medical Center in Jerusalem.
ISRA was founded in 1927 by Dr. I. Itzkovitch, who led the organization for 40 years. ISRA has a professional role (setting standards and negotiating salaries), and an academic role (setting residency requirements, responsibility for national boards, organizing annual meetings, and national congresses).
From the outset, radiologists developed a strong reputation for research, Sosna said. An important early paper about tinea capitis was published in German in 1932 by a radiologist from Jerusalem, Dr. Adolf Druckmann.
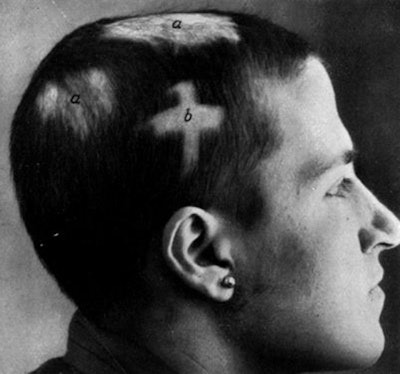
Most radiologists work in hospitals and clinics in the public sector, and all have board certification. Many of them have trained outside of Israel, including fellowships in North America, and about 100 migrated to Israel from the former USSR in the 1990s, according to Sosna.
Undergraduate medical training lasts six years and the average United States Medical Licensing Examination (USMLE) scores are comparable to those of U.S. medical school graduates. Radiology residency programs are similar to the Western European and North American models, he said. The academic syllabus is revised every five years. The clinical rotation comprises internal medicine, surgery, or pediatrics, or any combination, while the basic research element must include a written research plan and a scientific report or publication.
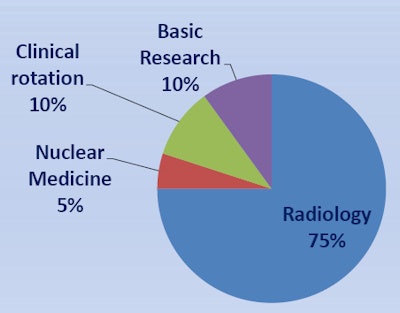
The oral examination consists of 10 sections: gastrointestinal, genitourinary, chest, neuro, ultrasound, pediatrics, interventional radiology, musculoskeletal, cardiac, and breast. Each section lasts 25 minutes and comprises six short cases and six long, comprehensive cases. All residents answer the same standardized cases and must pass at least eight sections. The minimum number of cases for the chest are 800 chest x-rays, 400 CT exams, and 10 interventional procedures, plus 15 CTs and five MRIs for cardiac.
Growth of deep learning
Another presentation focused on Israel's large number of medical imaging deep-learning companies, start-ups, and several deep-learning academic centers that are thriving in the information age.
There has been an exponential growth of visual data over the past decade, which is also apparent in the field of radiology, and deep learning convolutional neural network (CNN) algorithms have emerged as a way to categorize this vast amount of data, according to Dr. Eyal Klang, staff radiologist in the Abdominal Imaging Unit at the Chaim Sheba Medical Center in Tel Hashomer.
For instance, the ImageNet database is a large visual resource that contains millions of hand-annotated images. In 2012, a CNN algorithm showed a remarkable accuracy in labeling these images, and since then, newer CNN algorithms have shown greater accuracy until in 2015 a CNN algorithm achieved a near-human accuracy level in several image categorization tasks, Klang stated.
Artificial neural networks (ANNs) are deep-learning algorithms that are loosely based on the hypothesis of how biological neural networks operate. A large amount of small computational functions ("neurons") are interconnected in hidden deep layers. A key concept of ANNs is backpropagation, in which data are entered into the network and an output is produced. A loss function estimates the error, and based on the estimation of error, the algorithm optimizes the network by backpropagating into the network. The process of forward and backpropagation is iterated on the entire dataset.
CNN is a subtype of ANN, Klang said. This algorithm operates by propagating small filters (small matrices of numbers) across the image to create the neuronal maps. The process of loss estimation and backpropagation is conducted on the network in a similar way to an ANN.
There are similarities between how CNN operates and the thought process of experienced radiologists. "CNN has a gestalt or 'Aunt Minnie' flavor, as unlike previous machine-learning algorithms, there is no feature selection CNN," Klang added.