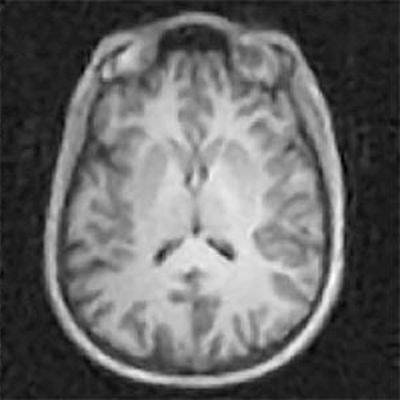
An artificial intelligence (AI) technique developed at Massachusetts General Hospital (MGH) can enhance the reconstruction process for medical images, decreasing radiation dose in x-ray, CT, and PET scans and hastening MRI acquisition, according to research published online March 21 in Nature.
The method, called automated transform by manifold approximation (AUTOMAP), uses a deep neural network to automatically determine the correct image reconstruction algorithm. Producing higher-quality images from less data, the model delivers quantifiable improvements in image quality and noise reduction, according to the team led by Bo Zhu, PhD, a research fellow at MGH's Athinoula A. Martinos Center for Biomedical Imaging.
"In addition to improving the reconstruction performance of existing acquisition methodologies, we anticipate that AUTOMAP and other learned reconstruction approaches will accelerate the development of new acquisition strategies across imaging modalities," the authors wrote.
The best reconstruction algorithm
The conventional approach to image reconstruction involves a chain of signal processing modules that require manual tuning by experts. What's more, these modules often can't handle imperfections in raw data, such as noise, Zhu said in a statement. AUTOMAP, however, automatically determines the correct image reconstruction algorithm using deep learning.
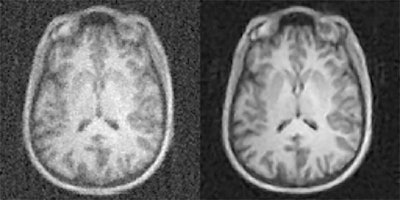
In addition to its image quality and dose reduction benefits, the MGH technique could also enable real-time decisions to be made about imaging protocols while the patient is in the scanner. Image reconstruction can be completed in just tens of milliseconds, said senior author Matthew Rosen, PhD, director of the Low-Field MRI and Hyperpolarized Media Laboratory and co-director of the Center for Machine Learning at the Martinos Center.
"Some types of scans currently require time-consuming computational processing to reconstruct the images," Rosen said in the statement. "In those cases, immediate feedback is not available during initial imaging, and a repeat study may be required to better identify a suspected abnormality. AUTOMAP would provide instant image reconstruction to inform the decision-making process during scanning and could prevent the need for additional visits."
AUTOMAP provides a new paradigm for image reconstruction that learns a reconstruction function for arbitrary acquisition strategies in order to reduce artifacts and improve reconstruction accuracy for noisy and undersampled image acquisitions, according to the researchers.
"We anticipate that the noise robustness attainable with our approach will improve imaging quality and speed for a broad range of applications exhibiting low signal-to-noise ratio, including low-dose x-ray computed tomography, low-light charge-coupled devices, large-baseline radio astronomy, and rapid volumetric optical coherence tomography," the authors wrote.
Furthermore, the researchers believe that their approach could enhance other AI and machine-learning applications in medical imaging, including computer-aided diagnosis.
"Our AI approach is showing remarkable improvements in accuracy and noise reduction and thus can advance a wide range of applications," Rosen said. "We're incredibly excited to have the opportunity to roll this out into the clinical space where AUTOMAP can work together with inexpensive [graphics processing unit]-accelerated computers to improve clinical imaging and outcomes."
The researchers have filed a patent application for the technology related to AUTOMAP.