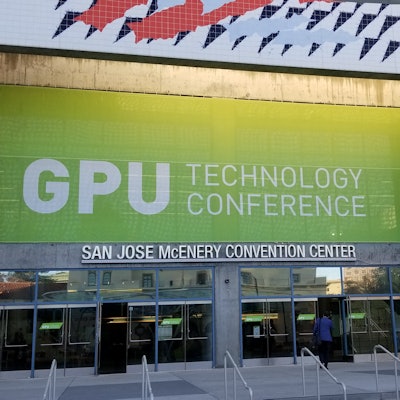
SAN JOSE, CA - Radiologists appear to have overcome their fears of artificial intelligence (AI) and are moving on to the nuts and bolts of making the technology work in clinical practice, according to a series of talks on March 28 at the NVIDIA GPU Technology Conference (GTC 2018). But the task won't be easy, and challenges remain.
The attitude of radiologists toward AI has shifted dramatically in just the past two years, according to Dr. Keith Dreyer, PhD, of Massachusetts General Hospital (MGH), who gave a talk on AI and radiology in his role as chief science officer at the American College of Radiology's (ACR) Data Science Institute. While Dreyer's talk at last year's GTC meeting assured radiologists that their jobs would not be taken by machines, this year no such warning was necessary.
"I don't see a lot of fear of AI anymore from a cultural standpoint," Dreyer said. "It was 90% against AI two years ago, and it's probably 90% in favor now."
Getting it done
Dreyer was one of several speakers on a Wednesday morning panel devoted to the challenges of making AI a tool for routine clinical use. In the year since GTC 2017, interest in artificial intelligence has risen to a fever pitch, with the technology dominating both RSNA 2017 and the European Congress of Radiology (ECR) earlier this month.
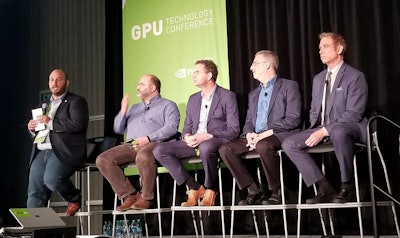
While progress has been made, all speakers on the GTC 2018 panel agreed that significant barriers still exist to AI being used on an everyday basis by radiologists. The technology is still in its infancy, and few AI algorithms have achieved widespread adoption.
To some extent, that could be because there is a disconnect between the developers of AI algorithms and the radiologists who will be using them, Dreyer said. A developer might focus on a specific problem, such as characterizing a lung nodule or developing a model for predicting malignancy. But sometimes these tasks don't meet the needs of radiologists.
For example, does it make a difference to a radiologist if an algorithm says a nodule has a 20% instead of a 30% likelihood of malignancy? What is a radiologist supposed to do with that difference?
Instead, there needs to be a closer collaboration between end users such as radiologists and algorithm developers, Dreyer believes. This can be facilitated by professional organizations, which can create the clinical use cases that developers can target for their apps.
Creating use cases
That's exactly what's being done at the ACR's Data Science Institute, according to Mike Tilkin, chief information officer at the ACR. The institute has been working on developing clinical use cases for AI that can be used to drive the development of new algorithms.
The work involves addressing a number of problems, Tilkin said. How will apps be used? What will be the thresholds for clinical action? How will apps be integrated with workflow?
Tilkin provided an example of how AI could be integrated into radiology workflow based on ACRassist, the group's clinical decision-support application in development. A radiologist might be working on a case using speech recognition-based reporting software, with a template for the type of case being reported. The radiologist would populate some fields, such as the size of the nodule, and AI would deliver findings and recommendations based on that information within the ACRassist screen, such as the Lung-RADS category of the nodule.
"We have a lot of hooks in place to start to plug AI in," Tilkin said. "For us, the key is going to be to identify the most useful places to start, the validation process, and the ongoing monitoring for adoption."
The challenge of challenges
An increasingly common technique for fostering the development of artificial intelligence is to hold a challenge, in which a particular problem is posed to the developer community in a public forum. Algorithms are developed to address the problem, and a winner -- who often receives a cash prize -- is chosen.
But challenges have challenges of their own, according to Wiro Niessen, PhD, a professor of biomedical image analysis at Erasmus University Medical Centre and Delft University of Technology in the Netherlands. Niessen is also president and board chair of the Medical Image Computing and Computer Assisted Intervention (MICCAI) Society, which has organized a number of challenges.
While such challenges have been great for publicizing algorithm development, they haven't solved the problem of getting AI apps into clinical use, Niessen said. There is often a wide variety and lack of standardization in the metrics used to judge the algorithms, and the results are not often reported in academic journals, making it difficult to reproduce them.
To address these issues, MICCAI has begun working with the ACR to have the ACR contribute its clinical use cases, which will become the subject of challenges presented to MICCAI members. Members from both groups plan to sign a memorandum of understanding on the collaboration at this week's meeting.
"You can define for all these use cases challenges, and you could have people working on algorithms, and these algorithms could be objectively compared using the criteria that are included in the different clinical use cases," Niessen said.
Getting involved
As organized radiology tackles the details of making AI more routine, some culture change is still needed to convince radiologists that the technology will help them. Fortunately, radiologists are no longer terrified that AI will take their jobs; in fact, Dreyer compared the current situation with AI to the arrival of MRI in the 1980s.
"There was this fear that MRI was going to be so good in its image quality that radiologists wouldn't be needed because everyone would see the anatomy," Dreyer said. "As we explain [AI] to our colleagues internally, we say that this is going to be changing constantly for years, for decades. This is going to have to be something either that you own, or you ignore and you'll get boxed out. And we see that in response to that, people are very positive -- they want to get deeper involved."