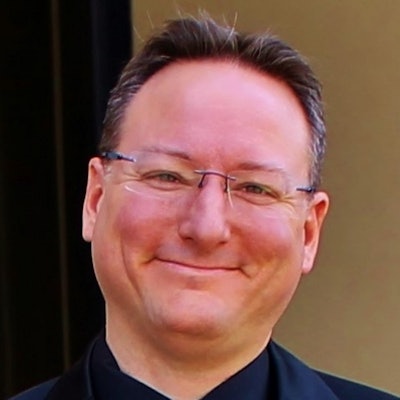
The development of artificial intelligence (AI) has been slowed by the need to test AI algorithms against large volumes of real-world imaging studies. But such databases are rare, and data transfer bottlenecks make it hard to move data from clinical sites to algorithm developers. But there is a better way.
By leveraging the power of vendor-neutral archives (VNAs), we can clear these bottlenecks and lay the groundwork for the more widespread use of artificial intelligence. To start, let's look at some of the VNA team's realities when faced with moving large volumes of imaging data from a clinical site that has the data to a vendor that wants to test an AI algorithm.
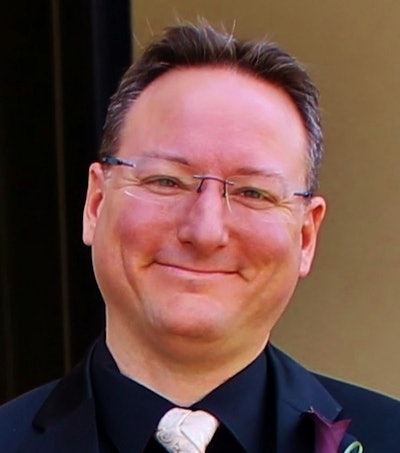
Often, the groups looking to purchase a site's imaging data for AI training are big-data vendors, for which server resources are, in effect, unlimited. When looking at the data from that "unlimited" perspective, requesting all data at once makes perfect sense. It is the fastest and most efficient method and allows them to segment the data at any time.
However, as a VNA owner, I do not typically have the excess capacity to support this type of wholesale data migration. Indeed, it takes many years to migrate high-volume amounts of data into the system. When a VNA is architected, it is built to support a defined operational volume, along with the overhead of migrating data into it.
Many, but not all, VNAs store data in a proprietary format that is like DICOM but is not a straight .dcm file. This means that to get data out, you can't simply copy the file; instead, you must do a DICOM transaction. Whether the data are stored as a DICOM file or not, both data transfer types require the additional step of deidentification, so although DICOM transactions add time, it is not the end of the world.
To put this data transfer into perspective, 1 petabyte is 1,024 terabytes or 1,048,576 gigabytes. In my experience, a single server tops out at somewhere around 15,000 studies per day, which is approximately 500 GB. So, less than 5/100th of 1% of that petabyte of data is moved. Doing the simple math, 10 servers dedicated to nothing but copying the data, ignoring a penalty for deidentification or additional compression, will move 1 PB in 209 days.
These obvious resource constraints make the wholesale data export impractical on the side of the VNA; therefore, a different model is needed, one in which both parties are willing to engage in a longer-term partnership. Whether for clinical research or training an artificial intelligence engine, it is likely that the buyer won't use all the data at once but is instead looking for very specific use cases.
As one AI executive recently told me, "The biggest problem in training AI is getting an appropriate and diverse dataset to train with." The following solution allows the dataset to be evaluated quickly and easily before either side expends significant resources in purchasing and/or moving data.
Instead of dumping billions of images on buyers and letting them sort through it all, I propose that we start by preparing a system that can provide data-rich answers versus volume-overload generic queries. To achieve this targeted approach, we must begin at the level of radiology reports, not images, and define the segment of the population from both a demographic perspective and the diagnosis. This requires building a database that holds all reports (not images) for the enterprise.
Simply start by pulling an extract from the electronic medical record (EMR) for all existing reports and then add the HL7 or Fast Healthcare Interoperability Resources (FHIR) connection to get all new reports. With the reports stored and demographics parsed into specific fields, the data can be queried and segmented in any way desired.
Here it is important that we have asked the right questions of the data buyer: in particular, what diagnosis is being researched or trained? What specific patient population is the target? The output of this query would be accession number, patient ID, date of service, and procedure description. Obviously, there should be a 1-to-1 relationship between the accession number on the report and the images in the VNA, but the additional output data will help if there is an accession number mismatch.
Armed with this export, savvy VNA teams can, instead of drowning buyers in millions of "chest x-rays," provide them with all the images from "nonsmoker males between the ages of 15 and 30 with a lung cancer diagnosis," if that's what they actually want. As a VNA team will be able to move 10,000 to 15,000 requested exams in a matter of days instead of months, this easily repeatable and relatively small-scale transaction can occur on a regular basis -- or whenever the AI or research team identifies more datasets they need.
This long-term relationship represents a win-win solution, one in which the buyer can evaluate the data upfront and identify and purchase a targeted set of data. The seller is able to monetize the data and move it in such a way that it does not impact daily operations.
With such a system in place, the overall state of healthcare and artificial intelligence will be improved!
Kyle Henson's 17-year career in healthcare IT began after he proudly served as an officer in the U.S. Army. He began in the payor space before quickly transitioning to the imaging sector, where he has spent the past 15 years expanding his knowledge base from PACS vendor to imagining consultant to hospitals. Kyle currently serves as director of enterprise imaging for a large multihospital system. These work experiences have allowed him to develop a deep, industry-encompassing understanding of current issues, trends, and successes. His work passion is finding solutions to the industry's problems (opportunities!) while always keeping a patient-centric focus. He can be reached by email or through his blog.
The comments and observations expressed are those of the author and do not necessarily reflect the opinions of AuntMinnie.com.