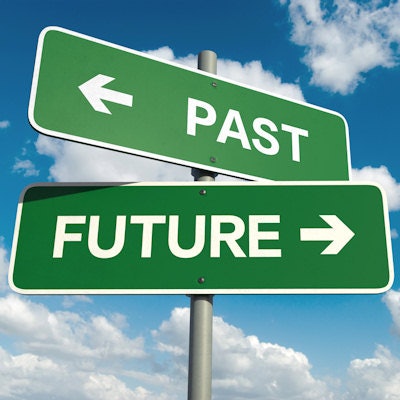
Much of what radiologists do today will be automated in the future, and it would behoove them to adopt artificial intelligence (AI) early to ensure their future value in healthcare, according to a presentation on April 27 at the Canadian Association of Radiologists (CAR) annual meeting in Montreal.
Speaking at the meeting about how AI can make healthcare better, Dr. James Min noted that machines are already outperforming radiologists in some tasks. It's not a good use of a radiologist's time to duplicate readings of images if deep learning, as an example, yields 93% accuracy, he said.
"Machines are better than us in almost everything we do where pattern recognition is involved and the task is redundant," said Min, who is a professor of radiology and medicine at Weill Cornell Medical College and director of the Dalio Institute for Cardiovascular Imaging at NewYork-Presbyterian Hospital in New York City. "We offer added value which a machine can never do."
Min said he believes the role of the radiologist in the future will likely no longer be to read 100% of the imaging studies. Instead, it will evolve into identifying the 7% of the time that the machine was incorrect.
Still in its infancy
Machine learning is definitely not going away, according to Min, with venture capital (VC) spending in deep learning expected to climb more than $1 billion in 2018, according to data from CB Insights. Still, he described AI in medicine as being in its infancy and not in a robust state, characterized by the existence of many VC-funded start-up companies -- some of which are offering single-use algorithms to assist in diagnosis.
He noted that the field of radiology has been suffering assaults on its raison d'être from the likes of Geoffrey Hinton, a cognitive psychologist and computer scientist who works with Google. Hinton has declared that medical schools need not continue to train radiologists because machines will be able to do all of the things that radiologists can do.
"The question is whether this is true or not," said Min, adding that it's unclear how AI will affect the care pathway.
"We were taught in science experiments to come in with a hypothesis, change one variable, and see if changing that variable affects the outcome," Min explained. "The machine does the opposite. It takes the data, and it finds a pattern in an unbiased fashion."
Augmenting risk stratification
In his own specialty as a cardiovascular imager, Min said the identification of vulnerable plaques that could lead to a patient experiencing a myocardial infarction is an example of where AI may assist in the future. It is clear that AI should not be limited to assisting in diagnosis; it should be explored for its ability to augment risk stratification, he said.
As an example of the potential utility of AI in this type of application, Min pointed to a recent advance in cardiovascular imaging that now helps to identify those at elevated risk for coronary artery disease (CAD). A 2015 study involving more than 27,000 patients led to the development of a scoring system -- based on clinical variables -- that can be used to identify patients at high and low pretest probability of having high-risk CAD. Identifying these patient populations can lead to personalized therapy, such as assigning medical therapy to some patients and more invasive approaches to others.
The plethora of data available to a healthcare professional involved in imaging -- an individual who also has to multitask on any given day, responding to email, participating in meetings, and being on conference calls -- means that the healthcare professional cannot devote time to processing images that contain millions of pixels.
"How can we process all of the data in medical imaging?" asked Min. "One of the answers is machine learning."
Embrace the change
Radiologists will face change in their professional lives with the entry of AI into their workspace, and it would behoove them to embrace that change, according to Min.
"In terms of where we will go in the future as a field of imagers, I think it is almost a certainty that the majority of what we do today will be automated in the future," he said, urging CAR attendees to adopt machine learning early on.
The application of AI to radiology reports involves natural language processing and will be feasible with structured radiology reports, he noted. However, AI needs to be well-studied and validated before it is incorporated into clinical practice, he said in a subsequent interview with AuntMinnie.com.
AI has to be held to a high standard: Algorithms based on small and not well-annotated datasets should likely be discarded, according to Min. The required size of a dataset will depend on the indication.
"If you are looking for nuances, then you will need lots of datasets that have [images that have] irregular shapes, sizes, and locations," he said. "In terms of the process of providing care, the computer does not do that better than we do."