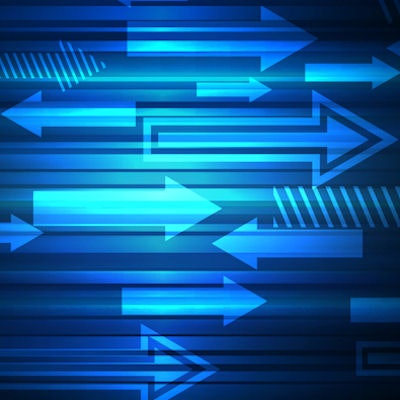
An artificial intelligence (AI) algorithm can prescreen head CT exams for a range of acute neurological events such as stroke, hemorrhage, or acute hydrocephalus, enabling triage of cases that require urgent review by radiologists, according to research published online August 13 in Nature Medicine.
Researchers from the Icahn School of Medicine at Mount Sinai trained a 3D convolutional neural network (CNN) to analyze head CT images and determine -- for the purpose of triaging -- if they contained acute neurological illnesses or noncritical findings. Despite being less accurate than radiologists, the algorithm was found in a simulated clinical environment to be much faster at providing notifications of critical findings. It was also effective at prioritizing urgent cases in a simulated radiologist worklist.
"Although the weakly supervised CNN classifier was less accurate than humans, it was 150 times faster," said senior author Dr. Eric Oermann, an instructor in the department of neurosurgery. "Because the system was designed for workflow triage rather than diagnostics, there was still a net benefit."
Speeding up diagnosis
The deep-learning project was the original study that launched the Mount Sinai AI Research Consortium (AISINAI), a group of scientists, physicians, and researchers in the Mount Sinai Health System dedicated to developing AI in medicine to improve patient care and help doctors accurately diagnose disease, according to the researchers.
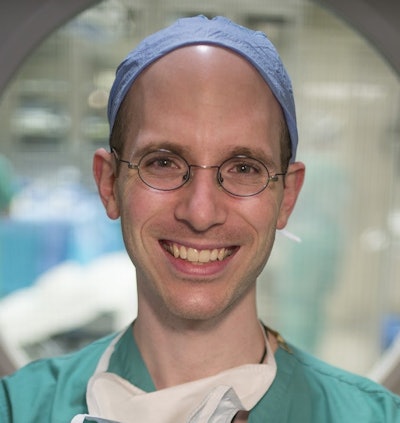
"It was the perfect combination of my personal experiences as a neurosurgeon and my technical training as a mathematician and deep-learning researcher," Oermann told AuntMinnie.com. "I was motivated by my specific experiences with taking care of patients with acute neurologic illnesses where any possible way of reducing the time it took for us to reach them could have potentially improved their outcomes."
Three years ago, Oermann and study first author Dr. Joseph Titano of the department of radiology decided to collaborate on applying deep learning to this challenge, according to Oermann. Oermann, Titano, and colleagues trained a 3D CNN on a dataset of 37,236 head CT scans. Because the images in this large dataset were not specifically annotated, or labeled, with pathology, the researchers had to use a "weakly supervised" learning approach, in which the model was trained using only the images and labels obtained from natural language processing (NLP) of their associated radiology reports. The work builds on a study published earlier this year by AISINAI on using deep learning to identify findings on head CT reports.
"Weakly supervised learning is one of my focuses as a mathematician because it is a more tractable problem from the perspective of building a medical dataset," Oermann said. "We were able to obtain noisy weak labels utilizing natural language processing on tens of thousands of images for over 100 diagnostic entities. It would have been prohibitively laborious and time-consuming to obtain strong labels (segmentations or bounding boxes) across a similar amount of data."
The CNN was trained to flag studies as critical or noncritical; the exams could then be ordered in a radiologist work queue based on the probability of a critical finding, according to the researchers. From testing of the algorithm, they determined that a triage system could be constructed that functions at a human level of sensitivity and could theoretically alert physicians in 50% of critical cases with a 21% false alarm rate.
Much faster performance
To see if the deep-learning technology could meaningfully triage studies, the researchers performed a randomized controlled trial in a simulated clinical environment to assess the method as an alarm mechanism as well as a triage system. The algorithm completed image preprocessing and inference in an average of 1.2 seconds, compared with an average of 177 seconds for radiologists to review the images and provide notification of a critical finding, according to the researchers.
When generating work queues for cases in the simulated clinical environment, the researchers also found that the AI algorithm's ability to triage urgent cases produced a quantifiable benefit. Urgent studies appeared earlier on a prioritized list than routine cases, and the difference in queue position was statistically significant (p = 0.01).
In the future, the researchers plan to compare and study the efficacy of both weakly supervised and strongly supervised classifiers with radiological imaging, as well as to explore approaches that combine the best of both methods.
"The challenge, as always, is to maximize the use of the available data," Oermann said.
The system has not yet been used clinically, as the group is primarily focusing on both theoretical and applied deep-learning research, he said.
"However, we are incredibly interested in finding ways of productionizing our approach to improve patient care," he said. "Our team is primarily compromised of physicians, and improving patients' outcomes is our foremost concern."