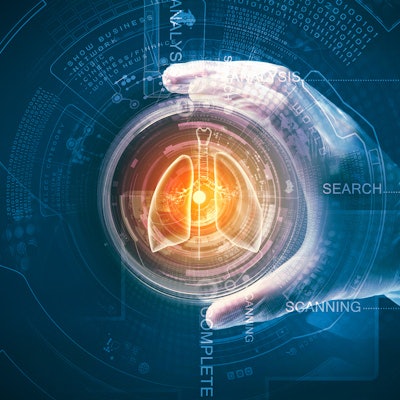
An artificial intelligence (AI) algorithm was able to distinguish benign from malignant nodules in cases of suspected non-small cell lung cancer on CT scans by analyzing the area immediately outside of the nodule, according to a December 18 paper in Radiology by researchers from Case Western Reserve University.
Adenocarcinomas are the most common type of non-small cell lung cancer, but they can be confused with noncalcified granulomas, a benign finding. It can be difficult for radiologists to differentiate between the two, especially in CT lung cancer screening programs.
One possible solution is the use of artificial intelligence technology such as convolutional neural networks (CNNs) to analyze nodule radiomics, or computer-extracted imaging features that can help indicate whether a nodule is malignant or benign.
But most AI algorithms focus on analyzing the nodule itself and its features, such as shape and texture. Could the performance of algorithms be improved by including the area immediately outside of the nodule in the analysis? A team led by Niha Beig, a doctoral candidate at Case Western, wanted to find out.
The researchers evaluated the effectiveness of AI algorithms in assessing nodule shape and textural patterns, as well as the perinodular area up to 30 mm immediately outside of the nodule. They hypothesized that this area outside of the nodule could provide key information in differentiating adenocarcinomas from granulomas, making AI more effective.
Beig and colleagues used a dataset of 290 patients; they divided it into a training set of 145 patients with 73 adenocarcinomas and 72 granulomas and a test set of 145 patients with 72 adenocarcinomas and 73 granulomas. The researchers used a variety of AI techniques to analyze the data.
Analyzing data both within and immediately outside of the nodule produced 80% accuracy for differentiating benign from malignant nodules, compared with 60% accuracy from a radiologist, the researchers found. The features most useful for predicting malignancy were located a distance of 5 mm from the nodule.
The group hypothesized that the algorithm's use of intranodular and perinodular features could make it more predictive than algorithms that rely solely on the analysis of nodule shape features. In a commentary on the paper, Dr. Mizuki Nishino of Brigham and Women's Hospital noted that the findings by Beig et al paralleled work in the field of breast MRI, where features just outside of the tumor area were analyzed and found to be predictive of a patient's pathologic complete response to treatment.
"The study added another promising, noninvasive, imaging-based strategy to achieve the ultimate goal of distinguishing malignant versus benign nodules with high accuracy and without delay," Nishino concluded.