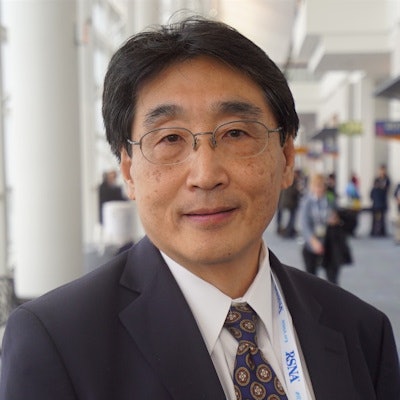
How can certified imaging informatics professionals (CIIPs) prepare for implementing artificial intelligence (AI) in radiology? By building an advanced IT infrastructure, according to Dr. Paul Chang, who spoke on Thursday in a webinar held by the Society for Imaging Informatics in Medicine (SIIM).
"You want to prepare your IT infrastructure to be able to consume all of these things that are going to come down the pike," said Chang, a professor of radiology at the University of Chicago. "You don't want to prepare [just] for AI; prepare for advanced IT. The good news is both big data and analytics as well as AI require the same infrastructure."
The best way to prepare your IT infrastructure is by what Chang calls "drilling for gas" and "building roads."
"AI and advanced IT is like a wonderful race car, but like any car, it still needs gas and roads or it's an expensive waste of metal," he said. "In the world of machine learning, gas is data and the roads are workflow orchestration, which means we need a very capable IT infrastructure."
Unfortunately, that type of infrastructure is lacking today for most institutions, he said.
Drilling for gas
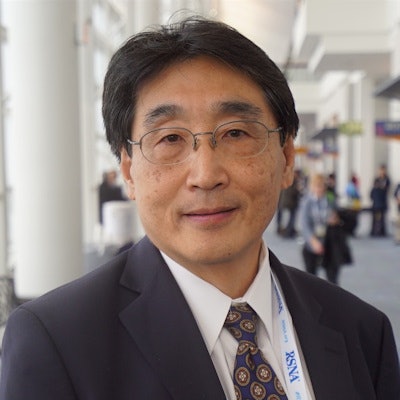
Chang noted that many of the current AI applications in radiology are significantly constrained, often driven largely by data availability rather than truly compelling use cases.
"That's why we have so many bone age algorithms," he said. "The problem is that you don't want an application that is driven by the availability of data; you want to be driven by something that will actually help your patients."
This creates a catch-22: Institutions may find it difficult to invest in more advanced IT infrastructure without compelling use cases for AI, but compelling AI algorithms can't be built without the advanced IT infrastructure, Chang said.
IT infrastructure challenges for "feeding" AI applications include being able to have all desired data accessible at scale and in real-time, being able to trust the data, and being able to reliably correlate data with reliable outcome measures at scale, according to Chang.
Workflow orchestration
And then there are the challenges associated with consuming the results of these systems. Instead of just recapitulating the existing and problematic computer-aided detection (CAD) model, AI applications need to be seamlessly and effectively integrated within existing workflow, Chang said.
"What we need is the yin to the [electronic medical record's (EMR)] yang -- in other words, data and contextually driven human-machine collaborative optimized workflow orchestration," he said. "The problem with that is, right now, we have this EMR-centric architecture, where it requires humans to do all of the workflow; we require humans to do all of this work. This is a problem because in order for machine learning, AI, and deep learning to work, machines have to consume information from other machines, and our systems don't do that."
There are a number of methods for getting data from the EMR and other clinical systems, Chang said. The University of Chicago has implemented an approach based on service-oriented architecture (SOA) to provide enterprise integration.
His strategy for big data and deep learning is to hedge, he explained.
"If I have operational responsibility for the IT staff for imaging, I want to prepare my existing IT infrastructure to be able to feed and consume advanced IT, not just deep learning but all of the advanced IT -- big data, analytics, the whole bit," Chang said. "I want to make sure that I have better data and interoperability. I want to go beyond an EMR-centric view, and I want to be able to go beyond this human dependence on data availability."
Building roads
Better workflow orchestration is required in radiology, he said.
One of the problems is that many of the AI start-ups in radiology are focusing on image analysis applications. However, the early winning use cases will likely focus on applying AI to improve efficiency and decrease variability, according to Chang. This could include, for instance, improving image acquisition time by reducing noise, optimizing radiation dose, and optimizing hanging protocols automatically.
"Help me get rid of all of the busywork that's wasting my time and adds to the burnout," he said. "Augment me, in other words: Have real-time human-machine collaboration whereby my machine buddy is using AI and helps me get through the busywork to [move me from being] a drone worker to more of an executive decision-maker."
Suggestions for CIIPs
Because it's too early to pick a winning model for implementing AI, it's a good idea to hedge and focus on preparing your IT infrastructure to be able produce better data and achieve workflow orchestration -- going beyond the CAD model, Chang said.
"You want to engage with your clinicians to get meaningful [AI] use cases, not 'nice to haves,' " he added. "You want to look for the minimally heuristic use case sweet spot: things that improve efficiency, things that the C-suite cares about. C-suites tend to pay lip service to quality. It's not that they don't care about quality, they [just] don't want to pay for it. What they want to pay for is efficiency, but that's OK, because both quality and efficiency loathe the same enemy, and that's variability."
Deep integration of these technologies into the workflow will be crucial.
"The goal is data-driven, optimized human-machine cybernetic workflow orchestration," Chang said.
He emphasized the importance of staying engaged and avoiding the "arXiv" hype phenomenon; these systems must be critically evaluated by the clinical domain.
"[CIIPs] get the leadership position and are going to basically provide the reality check to the clinicians and the administration who keep saying, 'We need to do something about AI,' " he said.