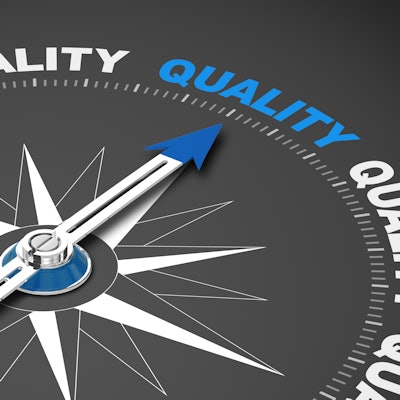
An artificial intelligence (AI) algorithm helped Harvard Medical School researchers conclude that radiologists in the same subspecialty division can vary significantly in how often they recommend follow-up imaging examinations, according to an article published online May 7 in Radiology.
After utilizing a machine-learning algorithm to identify follow-up recommendations among more than 300,000 radiology reports, the researchers led by Dr. Laila Cochon, PhD, determined that variation between the follow-up recommendations of radiologists could be as high as nearly sevenfold.
"This suggests that interventions to reduce unwarranted variation in follow-up recommendations may be targeted to individual radiologists," Cochon and colleagues wrote.
The retrospective study encompassed a cohort of 318,366 CT, x-ray, MRI, and ultrasound studies interpreted by the institution's abdomen, chest, cancer institute, emergency, musculoskeletal (MSK), and neuroradiology divisions in 2016. Using a natural language processing algorithm that had been developed and previously validated by the researchers, Cochon's group found that 38,745 (12.2%) of these radiology reports contained recommendations for additional imaging. Multivariable logistic regression analysis was then performed to assess each radiologist's likelihood of recommending follow-up studies.
Variation in follow-up imaging recommendations by radiology division | ||||||
Cancer institute | Chest | Abdomen | MSK | Neuro | Emergency | |
Variation in adjusted probability of follow-up recommendations among attending radiologists | 2.8-fold difference | 3-fold difference | 3.6-fold difference | 5.1-fold difference | 5.9-fold difference | 6.8-fold difference |
"Modality was [a] significant [predictor of interradiologist variability] across all division levels, and other factors had varied effect in each division, which highlighted considerations that are exclusive to subspecialized imaging," the authors wrote. "Individual radiologist factors, not including radiologist sex or years of experience, had the largest contribution to the observed variation. Our findings suggest that the same diagnostic finding may yield different follow-up recommendations depending on the radiologist reading the images, which could result in important implications for patient care."
In other results, radiologists were more likely [odds ratio, 1.01 for each additional year] to recommend follow-up imaging for older patients and for CT studies (odds ratio, 4.2 compared with radiography). They were less likely (odds ratio, 0.9) to recommend follow-up imaging for male patients, however.
"Interventions could include feedback reports that show follow-up recommendation rates for individual radiologists, educational efforts to improve awareness and acceptance of evidence-based imaging guidelines, and improved decision support tools," the team wrote. "Future studies will be needed to assess the effect multifaceted interventions have on reducing interradiologist variation in follow-up recommendations and the effect on quality of care."
In an accompanying editorial, Dr. Eric Russell of Northwestern University in Chicago noted that initiatives for improving uniformity could be aided by broader adoption of rules such as the Fleischner criteria for pulmonary nodules and rules for decreasing variation in follow-up imaging recommendations for thyroid nodules. AI and deep learning may be helpful for continuously analyzing the success of these strategies, according to Russell.
"Recognition of variability is a valuable tool to drive improvement, and it can encourage us to devise methods or protocols to make recommendation rates more uniform among radiologists with variable thresholds and experience," Russell wrote.