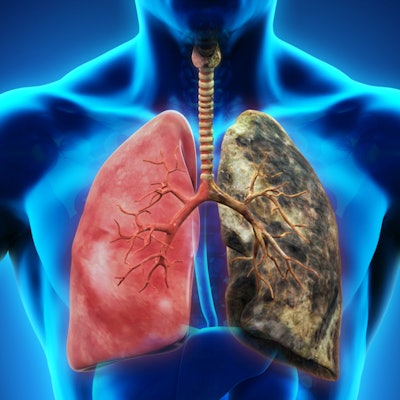
Artificial intelligence (AI) algorithms can accurately identify the presence of cancer and changes in disease burden described on radiology reports, potentially paving the way for personalized medicine on a large-scale basis, according to research published online July 25 in JAMA Oncology.
First author Dr. Kenneth Kehl and colleagues at the Dana-Farber Cancer Institute in Boston reported that deep natural language processing (NLP) yielded areas under the curve (AUCs) of over 0.90 for ascertaining if lung cancer was mentioned on radiology reports and if the cancer had responded to treatment. The NLP algorithm also performed similarly to human curators of the radiology reports for measuring disease-free survival, progression-free survival, and time to improvement or response.
"By reducing the time and expense necessary to review medical records, this technique could substantially accelerate efforts to use real-world data from all patients with cancer to generate evidence regarding effectiveness of treatment approaches and guide decision support," the authors wrote.
As part of their effort to apply AI to extract valuable cancer outcomes from unstructured radiology reports, the researchers had eight human curators use a structured framework to annotate a total of 14,230 radiologic reports from 1,112 patients who had undergone tumor genotyping at their institution for lung cancer. After training and validating the models, the researchers tested the algorithms on a test set of 109 patients.
Deep-learning model performance on test set of radiology reports | |
Deep-learning model (AUC) | |
Detecting any cancer | 0.92 |
Ascertaining worsening/progression of cancer | 0.94 |
Determining improvement/treatment response | 0.95 |
In a separate set of 1,294 patients with 15,000 additional radiology reports that hadn't been manually curated, the researchers found that the NLP algorithm's findings of worsening or progression of cancer were associated with lower overall survival (hazard ratio for mortality: 4.04). Meanwhile, findings of improvement or treatment response were associated with higher overall survival (hazard ratio: 0.41), according to the authors.
Furthermore, the NLP models were able to annotate imaging reports for the entire lung cancer cohort in approximately 10 minutes, while the human curators could annotate only approximately three imaging reports per hour.
The researchers said they next plan to test their technique in other healthcare systems and clinical environments, as well as apply it to evaluate associations among tumor profiles, therapeutic exposures, and oncologic outcomes.
In an accompanying editorial, Dr. Andrew Daniel Trister, PhD, of Oregon Health & Science University said that efforts to leverage the vast amount of data already digitized in the health system are reasonable first steps toward fulfilling AI's promise for lowering barriers to care and improving outcomes for large populations.
"Just as our practice of oncology has been transformed by molecular techniques in the past decade, artificial intelligence will transform how we care for patients in the next decade," Trister wrote. "As both the generators of the data being in these solutions and ultimate end users of clinical neural networks, it is incumbent upon clinicians to help shape how we work together to improve the lives of patients."