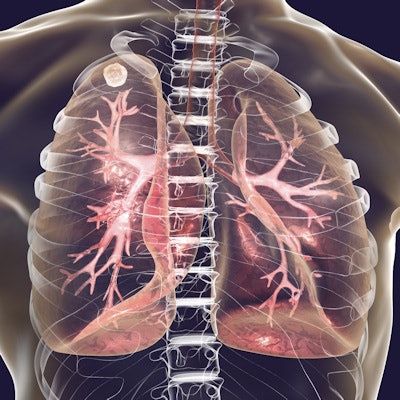
Many research studies reporting on the diagnostic accuracy of artificial intelligence (AI)-based software for detecting pulmonary tuberculosis (TB) on chest x-rays include design shortcomings that call into question how well the algorithms would work in the real world, according to an analysis published online September 3 in PLOS One.
A team of researchers led by Dr. Miriam Harris of McGill University in Montreal and Boston Medical Center found that studies that describe how computer-aided detection (CAD) algorithms work in a testing environment had a greater potential for bias than studies that examined how the algorithms worked in real-world clinical environments.
"AI-based CAD programs are promising, but more clinical studies are needed that minimize sources of potential bias to ensure validity of the findings outside of the study setting," the authors wrote.
Development vs. clinical
The validity of claims made about the accuracy of AI algorithms has become a major point of contention in the discipline. Some AI developers claim that their algorithms perform as well as or better than radiologists -- claims that are often examined skeptically by radiologists themselves, who counter that the algorithms weren't tested under routine clinical conditions.
To examine the question, researchers decided to focus on the evidence base for using CAD software in spotting TB. In particular, they compared claims made in two types of studies: development studies, which report on the creation of a CAD algorithm in terms of training and testing, and clinical studies, which evaluate the clinical performance of an algorithm that had been developed previously.
They searched four databases for articles published on this topic between January 2005 and February 2019. They then used a modified Quality Assessment of Diagnostic Accuracy Studies (QUADAS)-2 approach to assess the risk of bias for the 53 studies.
Of the 53 studies included in the analysis, 40 were development studies and 13 were clinical studies. All clinical studies employed machine learning-based versions of the CAD4TB software.
"In all quality assessments, when the reference standard used for determining a CAD program's diagnostic accuracy was image interpretation by a human reader instead of microbiologic testing of sputum, we judged this as a potential source of bias," they wrote. "This is because human interpretation of [chest x-rays] is moderately specific for [pulmonary TB], has variable sensitivity, is marked by limited interreader reliability, and the reproducibility is limited."
Accuracy of AI for detecting pulmonary tuberculosis, by study type | ||
Development studies | Clinical studies | |
Median area under the curve | 0.88 | 0.75 |
"This [difference between development and clinical studies is] likely because of the greater risk of bias due to the lack of prespecified threshold scores, the use of the same databases for training and testing, and the use of a human reader as the reference standard," they wrote.
In other findings, the researchers determined that deep-learning software yielded a higher median area under the receiver operating characteristic curve (AUC) than machine-learning software (0.91 versus 0.82). That difference was also statistically significant (p = 0.001).
The authors offered some suggestions to improve the clinical applicability of future CAD studies, including that researchers should describe how chest x-rays were selected for training and testing. In addition, AI algorithms should be trained and tested using chest x-rays from distinct databases, according to the authors. Ideally, they should also be tested against a microbiologic reference standard.
"Lastly, if the software has a continuous output, the threshold score to differentiate between a positive or negative [chest x-ray] should be reported, along with how this was determined," the authors wrote. "The U.S. Food and Drug Administration (FDA) requires all of these standards be met and additionally necessitates clear instructions for clinical use in their guidelines of CAD applied to radiology devices."