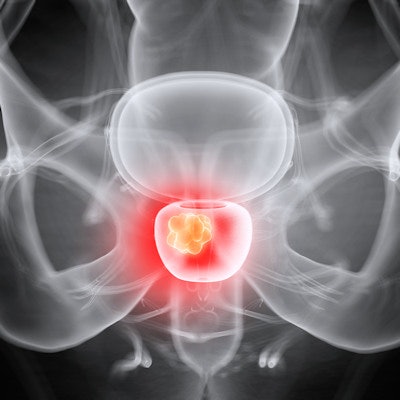
The combination of an artificial intelligence (AI) algorithm and radiomics can accurately and automatically predict the aggressiveness of prostate cancer on dynamic contrast-enhanced MRI (DCE-MRI) exams prior to biopsy, according to research published online September 5 in Clinical Radiology.
After training and validating several different machine-learning models for discriminating between patients with low or intermediate risk and those at high risk of invasive prostate cancer, a research team led by Dr. Bo Liu of the Second Affiliated Hospital of Chongqing Medical University in Chongqing, China, found that the best method yielded 90% accuracy.
"It is a valuable means to help clinicians determine the appropriate treatment for their patients and has wide applicability in clinical practice," they wrote.
Prostate cancer patients with Gleason score (GS) of 7 or lower have a higher biochemical disease-free survival and lower prostate cancer-specific mortality than patients with a score of 8 or higher. As a result, the researchers sought to investigate the clinical value of combining radiomics and machine learning analysis of multiphase DCE-MRI for discriminating prostate cancers with low/intermediate (GS ≤ 7) risk and high invasive potential (GS ≥ 8) prior to biopsy. In a retrospective study, they included 40 lesions from 40 patients with pathologically confirmed prostate cancer and a Prostate Imaging-Reporting and Data version 2 (PI-RADS v2) score of 4 or 5.
All patients had received biopsy within four weeks after a DCE-MRI exam. Using the time-signal intensity curve, lesion segmentation was performed on the first and on the strongest phase of the enhancement of the original DCE-MRI images. Next, 1,029 quantitative radiomics features -- including first-order statistics; shape-and-size features; textural features; and higher-order statistics features -- were automatically calculated for each lesion. The researchers then applied several techniques to select the most worthwhile radiomics features for use with the machine-learning model.
Finally, the researchers trained and tested five different machine-learning approaches: support vector machine based on linear kernel; logistic regression; random forests; decision tree; and k-nearest neighbor. Each method was independently trained and validated using fivefold cross-validation. The researchers also performed correlation analysis to determine the features of the machine-learning model -- logistic regression -- that achieved the best classification performance for the prostate cancer lesion.
Performance of best classifier for classifying prostate cancer invasiveness | ||||
Sensitivity | Specificity | Accuracy | Area under the curve | |
Machine-learning algorithm | 86% | 94% | 90% | 0.93 |
"Radiomics features can clearly reveal tiny changes in the histological anatomy and pathophysiology of neoplasms that are hard to identify quantitatively with the naked eye and are often overlooked in clinical practice," the authors wrote.
The researchers noted that features extracted from the first phase of tumor enhancement have a stronger association with the pathophysiology of carcinoma than those extracted from the strongest phase.
"As a new technology, this method is independent of conventional clinical assessment," the authors wrote. "It may be an effective complement to conventional MRI and included in the new version of PI-RADS in the future."