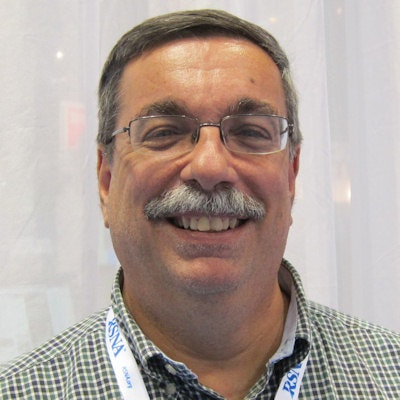
Many radiologists are concerned about the impact artificial intelligence (AI) will have on their future. From what I have seen so far, they needn't worry. For many reasons, the AI market is taking at least two to three times longer to develop than it should have. It has been a case of ready, fire, aim.
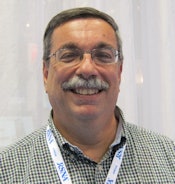
Misinformation about AI has contributed to greatly stunting the growth of AI -- and continues to do so. Early on, the "AI will replace radiologists" refrain sparked fear among the imaging community. That caused an immediate knee-jerk reaction and pushback from most radiologists. Yet it wasn't just misinformation that hurt the industry's development.
Research studies that highlight how AI can beat radiologists for a certain diagnosis show up almost daily and continue the drumbeat. It's understandable why some radiologists would wonder if they indeed want the wolf guarding the henhouse.
The resistance to the concept of AI taking the place of radiologists has been strong. Thankfully, much of this has been replaced with a more balanced, reasonable, and realistic approach in which AI is presented as a complementary technology to the radiologist's interpretation. Still, suspicions of AI remain, and rightfully so.
Not having the U.S. Food and Drug Administration (FDA) 510(k) clearance needed to use AI clinically has also hurt the industry. Interestingly, though it has been more than five years since the first AI algorithm showed up in the marketplace, less than one in three companies (40 to be exact, as of August 2019) have received FDA 510(k) clearance. That means 100 or so vendors have AI software that can't be included as part of the final diagnostic report, at least for now.
Reimbursement/ROI
Issues related to reimbursement/return on investment (ROI) that initially kept chief financial officers from writing checks have been addressed by some vendors who have been able to demonstrate AI's benefits in a different way. These tend to focus on improved patient care or implementing faster treatment protocols.
The suits in the C-suites getting these messages can often appreciate the value AI brings to the table, yet they need to overcome continually misdirected marketing messaging and so many other issues. Ideally, AI also needs to be seamlessly integrated into the interpretation workflow.
Sadly, very few companies have been able to show the integration of AI with PACS. Instead, most use AI in a standalone mode. This can significantly impact the interpretation process and negate many of the other benefits AI provides.
One of the biggest challenges we see in AI is the number of companies that are participating in the AI market. At last count, close to 140 vendors were offering AI solutions -- with more showing up every day in a market that, at best, can support a few dozen independent vendors. Over 100 AI vendors will be displaying their wares in the AI Showcase at RSNA 2019, more than double the number of vendors who displayed at RSNA 2017 just two years ago.
Subtle distinctions
When you are evaluating imaging modalities like CT, MRI, and ultrasound, the various brands are usually distinct enough that a customer can use features and functionality to decide on what unit is best for them. AI isn't nearly as easy. The distinctions here are much subtler and include factors such as research study sample size, sensitivity, and specificity.
The differences between various studies can at first glance seem statistically significant, but they aren't really important. Bigger isn't always meaningfully better. The difference in sample sizes of 10,000, 100,000, and 1 million studies might seem significant, but it usually represents a very small (less than 1%) increase in the confidence level. Most AI algorithms also don't achieve a correlation with radiologists' findings that exceeds 98%, so all the discussion of the sample size often becomes moot and only further confuses the buyer.
So how will the more than 140 vendors compete in the market? Some, like consolidators and all-in-one solutions, will survive by providing options to end users that are flexible and cost-effective. Some single-algorithm providers will survive when they become the de facto standard for a particular diagnosis -- detecting pneumothorax or intracranial hemorrhage, for example -- and end users purchase and implement them. Other companies will either establish OEM relationships with the major modality vendors or be bought out by them, especially if the price is right.
A significant advantage
Several incumbent vendors already have their FDA clearances and can cite several clinical implementations as well. These firms will have a significant advantage over those that are just entering the marketplace. The larger players, some of which have little to no true healthcare experience but an easily recognizable name, will also play a role -- even if the products they are developing aren't quite ready for prime time.
While this seems to defy logic, teaming up with a billion-dollar firm with star power instead of a bootstrap startup seems to instill a higher degree of confidence in an end user. This is true even if nothing has been shown to be real yet or those few sites that have had AI installed to great fanfare have produced less than stellar results. As for all other players, sadly, most will die on the vine, having not raised the $50 million or more it takes to survive in this marketplace for the next three to five years.
Even with only 40 vendors that can offer products where an AI interpretation can be included in the final report, 12 algorithms are already available for the brain, 10 for the breast, nine each for cardiac and lung, four for the liver, two for musculoskeletal applications, and eight in other areas. It's great that end users have so many options to choose from, but identifying the differences between each of these is a daunting task for the average radiologist.
In addition to all the commercial offerings, home-grown algorithms are also being developed by some of the major teaching institutions and others. I am sure all the algorithms are different -- and some of the differences may even be considered significant -- but who is going to perform the evaluation prior to implementation? And what are the specific criteria used to evaluate one algorithm over the next? Each algorithm will no doubt have its own clinical studies that document its performance so the "my algorithm can beat up your algorithm" game can continue to be played.
Difficult buying decisions
It will be up to the end user to decide which AI algorithm -- and/or company -- best meets their needs. Sadly, I would also venture to say that in most cases, the vendor with the lowest price will more often than not get the deal. End users simply don't have access to enough information to make an objective decision.
Just like with PACS, when you put all these algorithms side by side, they tend to blur. Yes, the algorithms are different, but is it worth $X more to pay for that difference? Will one of the algorithms show an ROI faster or serve patients better? Often the answer is yes, but most of the companies in the AI market don't know how to promote their own products. Some might say that statement is a tad harsh, but, if anything, it's overly generous.
At last year's RSNA meeting I talked with more software developers than I cared to. Few had a clue about what is in a radiology department, let alone things like a radiologist's workflow and how to talk about process improvement. I still chuckle at the young entrepreneur who told me he would save a radiologist two minutes reading a chest x-ray that's typically interpreted by a first-year resident in under 15 seconds.
Most AI vendor presentations haven't gotten much better in the past year either. Why? Because very few of these companies have staff who know anything about radiology and cardiology basics, let alone how to integrate AI into the interpretation workflow.
Venture capital spending
Nearly all the venture capital money raised by radiology AI start-ups has been going into research and development (R&D). Not nearly enough of these funds have been reserved for sales and marketing -- the two areas that ultimately determine whether a company lives or dies. There are a handful of exceptions, but very few.
When that glorious day arrives and a company's AI product is finally ready to launch, the company often finds out that the well has run dry. And unless it can convince more venture capital firms to pony up more money, the company will dry up as well.
Dale Carnegie is probably rolling over in his grave right now; this is NOT the way to win friends and influence people, PACSMan. But venture capitalists in AI provide very little direction to the companies and instead tend to give them carte blanche to spend the money they have invested as they see fit.
Since most of the entrepreneurs at the helm of AI companies come from the R&D side of the house, most of the money they get goes into R&D. That is a fatal flaw -- almost as bad as hiring a high-priced marketing and PR firm to promote AI products by sending the wrong message to the wrong clientele -- as a few AI vendors have already.
The AI market needs to understand how to aim before firing. Without that, AI firms might have better luck using a slingshot.
Michael J. Cannavo is known industry-wide as the PACSman. After several decades as an independent PACS consultant, he worked as both a strategic accounts manager and solutions architect with two major PACS vendors. He has now made it back safely from the dark side and is sharing his observations.
His healthcare consulting services for end users include PACS optimization services, system upgrade and proposal reviews, contract reviews, and other areas. The PACSman is also working with imaging and IT vendors developing market-focused messaging as well as sales training programs. He can be reached at pacsman@ix.netcom.com or by phone at 407-359-0191.
The comments and observations expressed are those of the author and do not necessarily reflect the opinions of AuntMinnie.com.