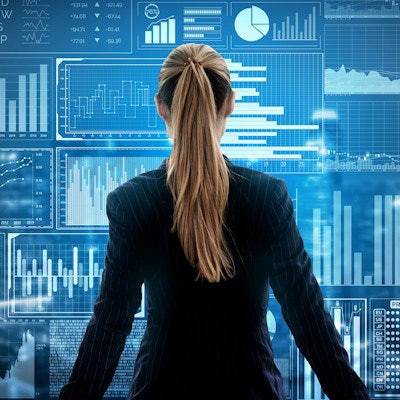
Business analytics based on artificial intelligence (AI) can create value for radiology practices by improving processes, optimizing resources, and enhancing workflow, according to an article published in the current issue of Seminars in Musculoskeletal Radiology.
Although many AI applications in radiology have focused on image interpretation tasks, AI can also tap into the wealth of nonpixel radiology data to shape the practice of radiology, according to Dr. Teresa Martin-Carreras of the Hospital of the University of Pennsylvania in Philadelphia and Dr. Po-Hao Chen of the Cleveland Clinic. In a review article, Martin-Carreras and Chen explored how AI is poised to enhance what the American College of Radiology has called the imaging value chain.
"The advent of AI in radiology lends a quantitative lens to the imaging practice to create more value for the patient and the referring physicians," the authors wrote (Seminars in Musculoskeletal Radiology, February 2020, Vol. 24:1, pp. 65-73). "We anticipate that integration of AI tools with [business intelligence and analytics] will continue to rise at a rapid pace, particularly as demands for quality and efficiency grow and our imaging informatics infrastructure grows increasingly complex."
Specifically, they believe there are 14 ways AI can create value for the six elements of the imaging value chain:
- Scheduling: optimization of scanner schedules; nonattendance prediction and prevention; delay and patient wait time optimization
- Appropriateness determination: clinical decision support for referring providers
- Protocol optimization: imaging protocol selection; flagging of incorrect orders
- Modality operations and workflow: anomaly detection on real-time dashboard; imaging quality control; and technologist workflow enhancement
- Reporting and communication: optimization of case assignment; automatic population of structured reports; longitudinal tracking of actionable lesions; and reporting at varying medical and literacy levels
- Billing: increased billing code accuracy
Development and adoption of AI in business analytics for radiology will be driven by regulatory drivers such as Medicare's Merit-Based Incentive Program (MIPS), alternative payment models, and the Hospital Readmission Reduction Program (HRRP), according to the authors.
AI could help practices to comply with MIPS by assisting in minimizing inappropriate follow-up recommendations -- differentiating inappropriate indications for preoperative use of cardiac stress imaging, for example -- and recommending alternative diagnostic tools for patients who have already received a recent CT or nuclear cardiology examination, Martin-Carreras and Chen added.
Alternative payment models (APM) such as bundled payments, accountable care organizations, and others may also spur development of AI.
"Emerging AI platforms that offer patient-specific health trajectory prediction by applying advanced AI on data across multiple databases in multiple practices and across multiple specialties may become feasible and valuable for all caregivers participating in the APM," they wrote.
The combination of AI and predictive analytics also shows promise for reducing hospital readmissions, for example, through recommendations for intervention that are based on the overall cost to the healthcare system.
"Specifically, the most salient growth will depend on the guidance of national professional societies such as the American College of Radiology to align AI development along appropriate standards, and the fastest business AI development is likely to arise from the pressure points along various regulatory drivers such as merit-based incentive payments and APMs," Martin-Carreras and Chen concluded.