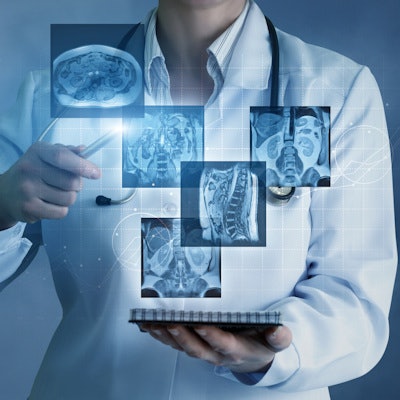
Artificial intelligence (AI) technology has much potential in radiology, but several key issues are limiting its current clinical utility, according to a presentation on Tuesday at a public workshop held by the U.S. Food and Drug Administration (FDA) on the evolving role of AI in radiology.
AI software is being used today for applications such as triage and workflow, quantification, and image preprocessing. However, AI algorithms face pressing challenges related to false positives, interpretability, and validation that are preventing them from reaching their full potential, said Dr. Peter Chang of the University of California, Irvine.
"With the proper collaborations and regulatory framework, hopefully we can start guiding that development to more clinically useful tools," said Chang, who is also a co-founder of Avicenna.ai.
Chang discussed current and future trends and the evolving paradigms in radiology AI software during his Tuesday talk.
Many false positives
Anyone who has used some of the current radiology AI applications immediately notices that they produce a very high number of false positives, according to Chang. A big part of the problem is that available AI tools for imaging don't take nonimaging context into account when analyzing images.
"Without the proper context, the images themselves really can only be interpreted to a certain extent," he said.
Another issue is that the prevalence of disease is very low. Assuming a 10% prevalence of a disease among cases being reviewed, an algorithm that has 80% sensitivity and 80% specificity has a 31% positive predictive value, he said. Increasing the performance to 90% sensitivity and 90% specificity only increases the positive predictive value to 50%.
Also, an AI algorithm that aims to triage emergency cases for radiologist review may slightly decrease turnaround time for the targeted disease but increase turnaround time for all remaining diagnoses, Chang said.
"That really gives us an opportunity to pin down the extremes of algorithm performance," he said. "On one hand, a high negative predictive value algorithm in which a human doesn't have to look at any of those images may potentially add some value to our workflow. On the other extreme, an algorithm that's extremely specific, so it misses a few cases but everything it shows the human is a true positive, that too is also another potentially useful application."
Interpretability
The interpretability of algorithms is an important issue. As stipulated by how that category of software is regulated, computer-aided triage applications are not allowed to annotate the images or provide specific feedback on what it's looking at, Chang noted. That can sometimes be a detriment, though.
"This seems like a trivial thing, but if I look through an image that an AI has marked as positive and I don't see anything, I end up spending more time to clear that negative exam than would otherwise be necessary," he said. "A quick two- or three-minute head CT is now much longer. The ability to localize specifically what you're trying to find is extremely valuable because if I see an artifact or some false positive, I can quickly exclude it and move on in my workflow."
Similarly, algorithms that are trained to provide a binary classification -- i.e., does a patient have a specific condition or not -- are trained very differently and have different underlying architectures than those that are developed to provide specific feedback. As a result, they may produce different types of errors.
In contrast to applications that provide a binary diagnosis, algorithms that are very specific in their feedback -- such as quantifying attenuation or mass effect -- tend to make more humanlike errors -- i.e., equivocal mistakes -- than random errors.
"Neural [networks] are extremely nonlinear, very complex functions that work most of the time, but occasionally may give you a result that is completely unexpected -- [an error] that my first- or second-year resident would not make," Chang said. "Those types of random errors are going to be extremely difficult to tackle in an autonomous AI framework."
Validation issues
There tends to be a discrepancy in what vendors report for their software's validation metrics and what an institution's own experience would be with the algorithms on their own data, according to Chang. This is due to a number of reasons.
For example, vendor performance measures may be based on analysis of a clean, curated dataset, but in clinical use, many aspects of the imaging chain could potentially introduce errors -- such as an exam with patient movement or that was incorrectly performed without contrast -- that would preclude accurate AI interpretation.
"Whether you solve this through another AI system or some other strategy, it is certainly something that you need to be thinking about," Chang said. "It is an imperfect pipeline with degrees of error all along the pathway, and your final performance is really a reflection of the synthesis of all of those different entities, not just the standalone algorithm performance."
Another source of error: Assumptions made about validation data have the potential to be flawed. During validation of algorithms, for example, it's very common for data to accidentally be leaked between different training and validation folds, he said.
But even if that problem is solved correctly, the data used for validation itself may be poorly generalizable. Even if a developer trains an algorithm using a dataset of 10,000 or even 100,000 patients, validation or testing may only be performed on a cohort of a couple hundred patients, Chang said.
In addition, some algorithms may be presented as being usable with all types of vendors and imaging protocols, but that heterogeneity was not reflected in the training dataset, according to Chang. And the ground truth used to assess performance may also be subjective.
"Certainly without question there is an opportunity here for a larger central and regulatory entity to perhaps create a standardized dataset, some sort of benchmark that can be easily compared across different types of applications and industry partners," he said.
Future trends
With the paucity of good, large, heterogeneous datasets, many creative learning paradigms have emerged for training algorithms using data from multiple sites. These include distributed deep learning, federated machine learning, and continuous fine-tuning of algorithms, Chang said.
In a distributed deep-learning concept, a single algorithm is trained simultaneously using data from multiple sites. A federated machine-learning approach can produce algorithms that are 90% trained based on data from other locations, with the last 10% being provided by the local site. Taking that model further, institutions could continuously fine-tune the algorithms using their own data, he said.
With the growing ease of building AI models, academic hospitals or university departments will increasingly take it upon themselves to create their own home-grown algorithms, according to Chang.
"I imagine there will be a rapid blurring between what we often consider a research project at a single institution versus full clinical deployment in the hospital," he said. "And so certainly the question here is what the potential scope of regulatory considerations might be. Will the regulatory burden be placed on companies whose job is to curate and aggregate models from different academic hospitals, or will [it] in fact be on the specific institution if they are producing many models that a lot of different hospitals are using?"
Autonomous readers
Interest is growing in deploying radiology AI algorithms to serve as fully autonomous readers in specific clinical applications, producing reports without any human intervention. Algorithms with high negative predictive value would be popular in this paradigm, enabling a percentage of exams to not require radiologist review because the algorithm feels so confident that the study is negative, Chang said.
"These use cases are being studied most heavily in the CT world: noncontrast head CTs, chest CT screening, [etc.]," he said. "I will also say that cross-sectional modalities in general [are being considered] because they have the least subjectivity compared with something like x-ray or ultrasound."
But what level of performance will the software need to achieve to serve as an autonomous reader? Will it need to be at the level of an expert radiologist, or will it have to have superhuman performance? These questions need to be answered, Chang said.
He noted, however, that if an AI model makes even one of the types of random errors that would have been caught by a junior trainee "it will be unforgivable and difficult to defend from a liability perspective."