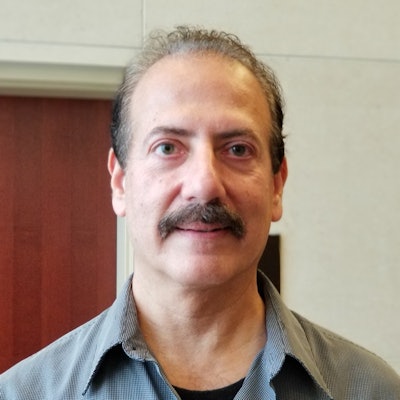
The second article in a two-part series on Dr. Eliot Siegel's presentation at AuntMinnie.com's Fall 2020 Virtual Conference (AMVC) details how artificial intelligence (AI) could help radiologists be more efficient and productive -- as well as less stressed -- during and after the COVID-19 pandemic. For part 1, click here.
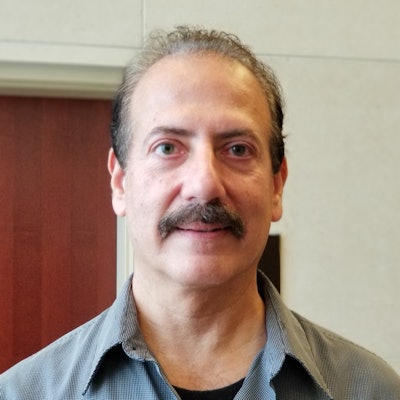
The COVID-19 pandemic will result in lasting changes to the practice of radiology. AI can help radiologists and radiology practices navigate this future in a variety of ways, including enhancing efficiency, speeding up imaging times, and improving workflow, according to Dr. Eliot Siegel.
Radiology practices today typically don't have the "elasticity" to handle the major swings in imaging volume that have been experienced during pandemic, said Siegel, of the University of Maryland and the Baltimore VA Medical Center. He noted that companies such as telemedicine firm TeleRay have developed what they refer to as an "elastic" telemedicine platform that takes advantage of the Coronavirus Aid, Relief, and Economic Security (CARES) Act that offers full Medicare reimbursement and waives geographic restrictions.
"I have little doubt that in the future, elastic solutions like this will become increasingly commonplace as practices struggle to deal with wide increases in volume, especially small radiology groups," he said.
There's also a trend toward larger radiology groups being better able to anticipate and respond more quickly than smaller groups to variability in imaging volumes, according to Siegel.
"We're also going to see AI algorithms to optimize having the right radiologist at the right time with the right expertise and the right availability to do image interpretation," he said. "And these larger groups have developed software to allow them to use predictive modeling to determine how to route imaging studies most intelligently and in a way that is optimized for efficiency, productivity, and patient care and safety."
Reducing scanning times
The COVID-19 pandemic will increase the pressure to scan more patients more rapidly in order to reduce patient and staff exposure, Siegel said.
"And what we're seeing now is an application of AI or deep learning to reconstruction of CT images, MR images, PET images, etc.," he added.
The major MRI, CT, and nuclear medicine vendors are in the process of applying deep learning to improve image quality and also reduce scan times and doses, according to Siegel. In CT, for example, deep learning has the potential to be able to maintain image texture, which is so important for AI algorithms and diagnosis, without a reduction in other important diagnostic features and without giving up increased noise," he said.
Generative adversarial networks (GANs) can utilize a large library of medical images to predict what a higher-resolution MR image would look like based on analysis of a low-resolution MR image, Siegel said. Similarly, GANs could take a short-acquisition PET scan and determine what it might have looked like with a longer acquisition or take a very low-dose CT scan and predict what it would have looked like at a higher dose. These techniques offer dramatic potential for faster scan times and lower dose for patients, he said.
Deep learning is just starting to be applied to CT, and it offers tremendous potential for noise reduction and ultralow-dose scanning, as well as the possibility to create super-resolution images and reduce artifacts and motion, Siegel said. For example, deep learning could enable significant reductions in the amount of time required for model-based iterative reconstruction.
"This can result in fairly dramatic improvements in image quality at very low doses," Siegel noted.
Stress, burnout, and fear
The next killer app for AI over the next five years in radiology will enable radiologists to improve their efficiency and productivity, while reducing the stress and burnout that they're experiencing, he said. Although all radiologists are looking to become more efficient and productive in the pandemic and postpandemic era, practicing radiology nowadays results in fatigue and burnout.
Previous research performed in the early 1990s at the Baltimore VA Medical Center found that radiologists spend as much as 85% of their time waiting for images on PACS, arranging images, and reporting cases; only 15% was spent on the cognitive aspects of image interpretation, Siegel noted.
In his AMVC talk, Siegel shared a story he had heard about a radiologist who had increased his efficiency level to nearly 75%, a productivity achievement that was enabled by the use of six staffers called radiology scribes. These scribes were responsible for retrieving images from one or more PACS and then letting the radiologist know that the studies were ready. The radiologist said what he thought as he scrolled through the concatenated sequences of images, and the scribes then created a cogent report for the radiologist to sign, according to Siegel.
"These scribes did a phenomenal job of allowing him to minimize stress and fatigue while being as efficient as possible," Siegel noted. "The key question is whether or not AI software could emulate some of the functions of the scribe and could [the radiologist] use AI software to arrange the images in a logical way that the radiologist finds most efficient? Could AI applications be able to allow the radiologist to speak in free form and then arrange the report in a similarly cogent manager and then to make the study available to be signed? I think this is the ultimate challenge for AI efficiency in asking how can one emulate these six scribes and be able to see such dramatic improvements in efficiency, while minimizing stress and minimizing fatigue."
AI challenges
Siegel also noted that there are still challenges in validating AI algorithms that are developed on one type of scanner, or in one geographic location with one patient population, etc. In addition, another challenge is determining what the gold standard should be for image interpretation.
"So does one teach an AI algorithm to be a high-sensitivity reader or a relatively high-specificity reader or does one go somewhere in between?" he said. "And so this whole idea of being able to have AI that gets trained in the lab that actually then is tailored to either an individual radiologist, or a particular set of machines or geographic location, or to a particular racial or ethnic population is a really compelling idea."
FDA assesses adaptive AI
In an exciting development, the U.S. Food and Drug Administration (FDA) has begun discussing internally whether it would be reasonable to have AI-based software as a medical device that remains safe and effective as it continues to learn based on different machines or patient populations or a particular radiologist, Siegel said.
As Berkman Sahiner, PhD, said recently at the Conference on Machine Intelligence in Medical Imaging (C-MIMI) 2020, it would then be possible to have AI algorithms that are either locked after approval, or at the other extreme, that are adaptive and allow continuous learning as they are used in clinical practice.
"This is a really exciting prospect that may allow us to be able to have algorithms that learn," Siegel added. "Particularly in the era of a pandemic such as COVID-19, the adaptive capability to be able to learn a new disease entity is really interesting and exciting and could revolutionize how AI algorithms are developed to allow for continuous improvement of the algorithms for a particular site -- more in line with personalized medicine."
Initiatives such as the FDA's interest in supporting continuously learning AI algorithms and the CMS' new technology add-on payment (NTAP) status for Viz.ai's stroke detection algorithm will result in fundamental changes in reimbursement and the way AI software is used in the next few years, according to Siegel.
"And it will have an enduring positive impact on the practice of diagnostic imaging in the future," he said.
To access AuntMinnie.com's Fall 2020 Virtual conference, click here. Presentations will be available for on-demand viewing for 30 days after the meeting concludes on September 23.