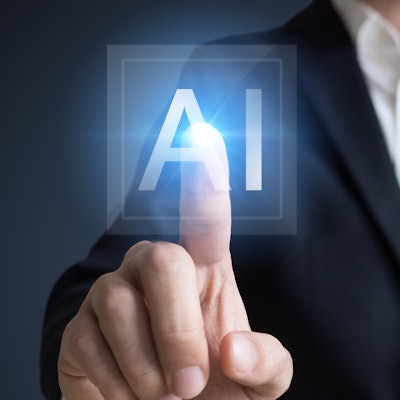
After lagging other sectors for years, the era of artificial intelligence (AI) in healthcare is dawning as its use is becoming more prevalent in a variety of applications -- from medical imaging and ophthalmology to remote monitoring and leveraging electronic health records (EHRs) to identify potential risk for chronic diseases or complications.
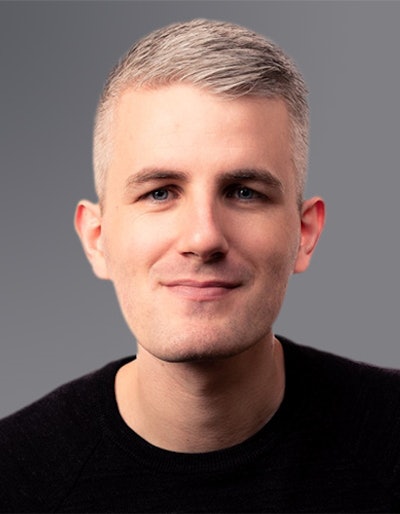
Medical applications of AI are becoming increasingly important because they can deliver significant benefits. These applications can assist with clinical decision-making by analyzing data quickly to deliver faster, better insights for diagnosis and treatment. They can also drive greater efficiency for providers by automating tedious and time-consuming administrative tasks.
The rapid pace of innovation is challenging for regulators who are charged with ensuring that any software used for medical purposes is effective and does not compromise health or safety. Even though the U.S. Congress and the Food and Drug Administration (FDA) are attempting to address these issues, there are still regulatory questions to be answered.
The FDA currently regulates some AI-enabled products, but not all. One of the main challenges is that the FDA's traditional regulatory framework and review processes are not designed to keep pace with this speed of innovation, as AI-enabled medical applications are evolving rapidly, sometimes in unanticipated ways.
Keeping pace
The FDA typically reviews medical devices through an appropriate premarket pathway, such as premarket clearance 510(k), de novo classification, or premarket approval. But these processes were not designed to effectively evaluate adaptive AI or machine-learning (ML) technologies.
Often, retrospective studies were used during the premarket pathways to collect data from clinical sites before evaluation. The studies' measurements of endpoints also usually did not include side-by-side comparisons of clinicians' work with and without AI. In addition, many evaluation studies failed to include multisite assessments of the AI- and ML-based medical products. The premarket pathways lacked sufficient prospective studies.
To address such shortcomings, in 2019, the FDA published a discussion paper that outlined a new approach. The proposed framework was based on four principles:
- Clear expectations on quality systems and good ML practices
- Premarket assessment of software-as-a-medical device (SaMD) products
- Routine monitoring of SaMD products by manufacturers to determine when algorithm changes require FDA review
- Transparency and real-world performance monitoring.
The FDA acknowledges this framework would be a significant change from how it has historically regulated devices and could require Congressional approval. Additionally, outstanding questions remain about how to actually implement the framework, and how it would be applied to specific devices.
An action plan
The FDA more recently published its AI/ML-Based SaMD Action Plan, which was developed in response to feedback from the 2019 framework proposal from key stakeholders and offers a multipronged approach to oversight of AI/ML-based medical software.
The plan is "based on total product lifecycle oversight to further the enormous potential that these technologies have to improve patient care while delivering safe and effective software functionality that improves the quality of care that patients receive," said Bakul Patel, director of the FDA's Digital Health Center of Excellence in the Center for Devices and Radiological Health (CDRH). "To stay current and address patient safety and improve access to these promising technologies, we anticipate that this action plan will continue to evolve over time."
Actions being recommended include:
- Further developing the proposed regulatory framework, including through issuance of draft guidance on a predetermined change control plan for software learning over time
- Supporting the development of good machine-learning practices to evaluate and improve machine-learning algorithms
- Fostering a patient-centered approach, including device transparency to users
- Developing methods to evaluate and improve machine-learning algorithms
- Advancing real-world performance monitoring pilots
While the FDA's framework and action plan are being considered, the momentum behind use of AI and ML in healthcare is only growing stronger. Already, there are nearly 350 AI and ML-enabled devices approved by the FDA, with the vast majority (70%) in radiology, followed by cardiology (12%), and hematology and neurology applications (3%).
The potential for improvements in outcomes and efficiency is even greater as we consider how new and evolving uses of AI and ML could enhance medical care, support busy clinicians, automate basic tasks, and speed diagnosis and treatment.
The FDA's framework and action plan are great steps in the right direction to ensure that the benefits of AI and ML are realized in healthcare, but we need to ensure that inaction or delayed response in addressing regulation of AI and ML do not stall innovation. Going forward, the regulatory process must be flexible and adaptable to ensure that patients and providers can benefit from safe and effective technology in a timely manner.
AJ Watson is chief product officer at image management firm Intelerad Medical Systems.
The comments and observations expressed are those of the author and do not necessarily reflect the opinions of AuntMinnie.com.