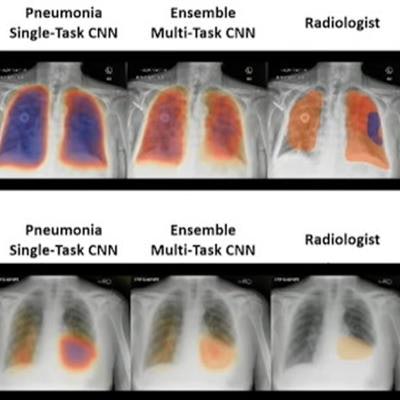
Researchers have developed an artificial intelligence (AI) model that can distinguish between pneumonia and pulmonary edema on single chest x-rays, according to research presented at the 2023 American Roentgen Ray Society (ARRS) annual meeting in Honolulu.
In an April 19 session on chest imaging, Dr. Melina Hosseiny of the University of California, San Diego described first how her group previously developed two single-task convolutional neural network (CNN) algorithms -- one that detects pneumonia and another that estimates serum levels of NT-proBNP, a protein released in the blood due to pulmonary edema.
The algorithms were promising in clinical tests, but were beset with false-positive findings, as these conditions often overlap, she said.
"We were thinking maybe we need a new strategy to combine these models in a multi-task CNN that actually can work better in patients who have pneumonia and pulmonary edema," Hosseiny said.
Both pulmonary edema and pneumonia involve the build-up of fluid in the lungs, but they are different conditions requiring different treatments, with pneumonia caused by infection. Distinguishing between them on imaging can be difficult, and researchers hypothesize that AI algorithms can help clinicians tell them apart.
To that end, Hosseiny and colleagues developed a multitask CNN algorithm that combined the two previous models and then tested its performance for detecting both conditions.
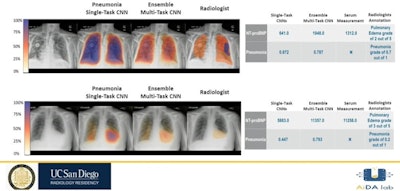
The researchers trained the multitask algorithm using two patient x-ray datasets, one from their university that included 26,798 images and one from RSNA that included 24,986 images. The datasets included a mixture of "less reliable" and "very reliable" images (as determined by expert radiologists) and were either labeled with the location of pneumonia or included NT-pro BNP lab measurements taken within 24 hours of the x-ray.
In a separate test dataset of 249 images, the multitask CNN model showed greater agreement with the expert radiologists for predicting pneumonia and similar agreement with radiologists for predicting pulmonary edema compared with the original single-task models, Hosseiny said.
Agreement with expert radiologists by type of AI algorithm | ||
Single-task model | Multitask model | |
Detecting pneumonia | 78% | 80% |
Detecting pulmonary edema | 83% | 84% |
"Our proposed multitask CNN outperformed single-task CNNs for detecting pneumonia and demonstrated excellent performance in detecting concurrent pneumonia and pulmonary edema in patients," Hosseiny said.
Ultimately, the value of the work is that it shows a multi-task CNN algorithm can successfully be used to aggregate different training data developed for multiple tasks, a relatively novel approach in AI research, she noted.
"This algorithm has the potential to serve multiple purposes, including assisting radiologists in reading chest x-rays, especially when patients have both pneumonia and pulmonary edema," Hosseiny concluded.