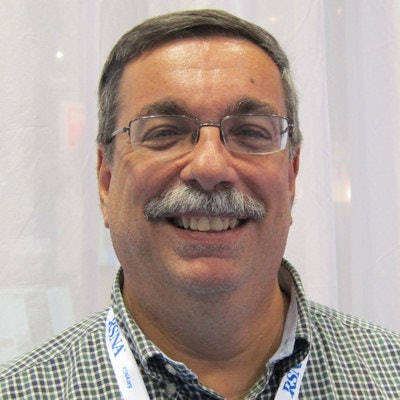
There's no doubt that research and commercial activity is surging for artificial intelligence (AI) in radiology applications: AuntMinnie.com published more than 175 articles on AI news alone in the past year. The fear, uncertainty, and doubt (FUD) factor remains a big hurdle to clear, however.
To be sure, there are real issues that need to be addressed. For example, few seem even to consider that there needs to be a justification for the purchase of AI -- like all other clinical systems and software used in the healthcare environment. Preferably, this justification should be in black and white; that's the world that people who control the purse strings tend to live in.
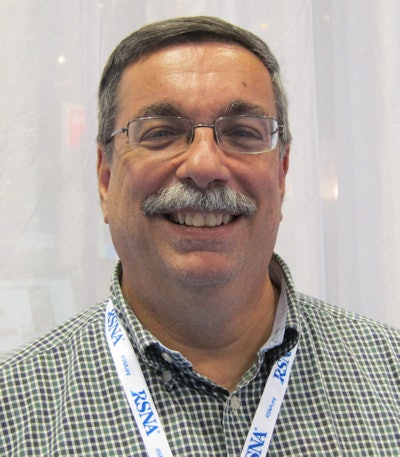
No one would debate that artificial intelligence offers the capability to significantly improve outcomes and with them, patient care. But how AI saves or makes money for a facility remains to be seen. How long will it take before regulators and insurance companies reduce their payments after recognizing that AI can generate a more accurate clinical support decision than one produced by clinicians alone?
Just this week an article came out about reduced dosage and scanning times through the use of AI. Both of these are positives and may indeed bring more business to a facility. But it's at best a "Field of Dreams" mentality ("If you build it, they will come") that primarily addresses patient care -- something you can't easily charge for now or address in a hard-dollar amount in a return-on-investment (ROI) model.
Fitting in the workflow
You also need to look at how AI fits into the existing PACS clinical workflow. No single company has the market on all or even some AI algorithms. Instead, every vendor seems to address one or just a few areas.
Collaboration and interoperability here are key. Some companies are joining forces, but not every vendor works with or wants to work with everyone else. As I noted in my 2017 PACSman Awards, AI is a market that's still in its infancy and barely touching the surface of its full potential. Since RSNA, we have seen several new algorithms released and virtually every major imaging vendor now entering the market with an AI solution, but we still have a very long way to go.
Another issue is that most of the preliminary assessments of the value of AI have been done at teaching and other luminary sites. While these are excellent sites to do an evaluation of the technology with participation of some of the top radiologists in the nation, their patient mix might not reflect the same patient population at a small regional community hospital.
More than 80% of the hospitals in the U.S. have fewer than 300 beds, so this has a significant "real world" impact. No one would question that there's value in knowing how many prostate cancer patients are most likely to benefit from multiparametric MRI scans, for example, but the average community hospital is not exactly tripping over patients wanting or needing to know this information. The same can be said for some of the newest algorithms that have been released.
Ethical issues?
Long gone are the days when a chest film was a standard part of a hospital admission. That said, even if that were the case, would the use of an AI lung nodule scan be indicated if the patient presented with no symptoms upon admission? I am far from a medical ethicist, but I'm not sure about any ethical issues related to looking for something that isn't dictated in the patient's clinical history.
All the focus has been on AI, yet it is important to recognize that without machine learning (ML) and deep learning (DL), there can be no AI. Machine learning trains software to perform a task and improve capabilities by feeding it data and information so it can "learn" over time. A machine-learning algorithm has to be told how to make an accurate prediction using the data it is fed. Good data produce good results; bad or inaccurate data yield more questionable results. The larger the dataset, the higher the probability of a more accurate interpretation. We need to look closely at the size of the dataset before determining the particular value of each algorithm being used.
Deep learning is a subset of machine learning and processes data like a human would using an approach known as an artificial neural network (ANN). In ANNs, there are "neurons" that have discrete layers and connections to other "neurons." Each layer picks out a specific feature to learn, such as curves/edges in image recognition. It's this layering that gives deep learning its name: Depth is created by using multiple layers as opposed to a single layer.
Cost justification
So how do you justify the cost of AI? What is the target market? And what is the true cost of AI? For any AI to work, it has to seamlessly integrate with the PACS software. What vendors have done this so far? None that I am aware of -- at least not on a large scale.
AI algorithms that have been developed for a special area such as cancer screening stand the greatest chance of being adopted by places that focus on cancer prevention, such as women's health centers. Cancer treatment centers are also another obvious target. But these are limited in number and alone can't support the development of a costly product like AI.
What about the regional hospital market? Unless an AI vendor is willing to put together a software-as-a-service (SaaS) "per-click" model -- for example, an $X charge for each scan done using its AI algorithm -- the cost is likely to be out of range for most. A quick check of ICD-10 codes also shows no reimbursement for the use of AI, so unless it's bundled in the scan cost or absorbed as a marketing cost, it won't be reimbursed. This may change, but as it stands now, AI is an out-of pocket cost.
Even using a best-case per-click scenario, the initial integration and implementation of a dozen or more AI algorithms from various vendors (vendor A for prostate scanning, vendor B for lung nodules, vendor C for brain bleeds, vendor D for digital breast tomosynthesis, etc.) is neither quick nor cheap. PACS vendors will all want to perform their own functional testing on the AI software that is being installed to ensure that it doesn't have a negative impact on their own PACS software operation. This is usually so far down the software development timeline it's not even funny.
A ways to go
So, is AI all it's cracked up to be in healthcare? In the pharmaceutical arena, AI's ability to ingest 25 million Medline abstracts, more than 1 million full-text medical journal articles, 4 million patents, and other data is impressive and light years better than the 300 to 400 manual searches that can be done manually. It can also take private data such as lab reports and help bring together and assess disparate datasets to show relationships and reveal hidden patterns -- combining scalable knowledge with predictive analytics and dynamic visualization. It still has a ways to go before being fully commercialized on a wide-scale basis, but it's already being used in amyotrophic lateral sclerosis (ALS) trials, research into Parkinson's disease, and other areas.
What about the use of AI in radiology? It has phenomenal promise, but realistically it will be at least several years before we start to see any practical applications of AI being used on a widespread clinical basis. Not only does AI need to undergo much further testing, but we also need to figure out just how we will pay for it, what applications it should (and should not) be used for, and, most importantly, how it can function as an adjunct to a radiologist's reporting process and augment the quality brought by years of a radiologist's experience.
The biggest barrier we have to adopting AI isn't the technology itself, though, but fear of the technology. Radiologists need to address the FUD factor (fear, uncertainty, and doubt) that has surrounded AI since its initial introduction. AI is not going to cost them their jobs. It'll be yet another tool to help them provide better care for their patients and, in the process, perform their role faster, more easily, and more accurately.
Michael J. Cannavo is known industry-wide as the PACSman. After several decades as an independent PACS consultant, he worked as both a strategic accounts manager and solutions architect with two major PACS vendors. He has now made it back safely from the dark side and is sharing his observations in this Straight Talk From the PACSman series.
His healthcare consulting services for end users include PACS optimization services, system upgrade and proposal reviews, contract reviews, and other areas. The PACSman is also working with imaging and IT vendors developing market-focused messaging as well as sales training programs. He can be reached at [email protected] or by phone at 407-359-0191.
The comments and observations expressed are those of the author and do not necessarily reflect the opinions of AuntMinnie.com.