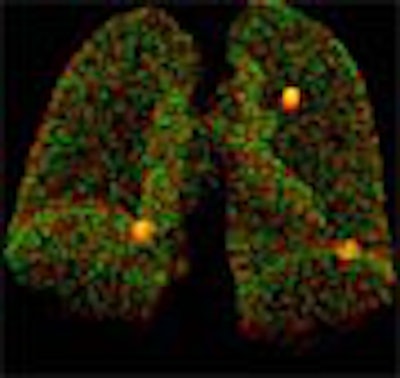
By all accounts, CAD has gotten pretty good at detecting lung nodules -- better than radiologists in many cases. But there's more to lung cancer imaging than finding the dots, and the radiologists whose job it is to connect them are still working mostly unaided.
In several presentations at the 2003 RSNA meeting in Chicago, researchers examined thoracic CT nodules beyond the issue of detection, including nodule tracking, analysis, and follow-up using both automated and manual tools. As for the near-term prospects of automated systems, the research seemed to indicate that if diagnostic robots aren't ready to take over the world yet, they've at least begun to roll up their digital sleeves and pitch in. Another group found that volumetric measuring tools are improving with the use of thin-section imaging, a happy development in light of new research showing that the accuracy of humans who evaluate small nodules remains highly variable.
Offering an early glimpse at thoracic CT's automated future was an all-purpose investigational lung CAD system discussed by Haili Chui, Ph.D., from R2 Technology in Sunnyvale, CA, in cooperation with Brigham and Women's Hospital in Boston.
The system was designed to not only find and measure nodules, but also to combine the findings from two different scans, establish correspondence between the original and followed-up nodule, and measure the growth in between scans, Chui said.
"It's a time-consuming process to track and monitor lung nodules," he said. "Normally you have to find them manually...But CAD can help reduce oversights and misses, provide more accurate and consistent measurements...and help automate the temporal comparison process."
The system works by automatically overlaying the original and follow-up studies, then warping them together using an anatomic landmark-guided algorithm. ImageChecker measures nodule volume, and calculates the rate of change including the doubling rate.
Chui's study was designed to test and validate the accuracy of the system. First, phantoms containing 24 acrylic spheres and cubes 3-10 mm in diameter were used to measure the accuracy and reproducibility of the automated volume measurements. Images were acquired using 1.3-mm section thickness and 0.6-mm intervals, and with mAs varying between 40-240 mAs to assess the role of dose. Fifteen repeat scans were acquired for each "nodule," and accuracy of the measurements was compared with known volumes.
![]() |
Original and follow-up CT lung scans are seen pre-alignment (above) and post-alignment (below) in a prototype CAD scheme that tracks nodules and measures their growth between exams. Workstation view (bottom) shows apparent growth of one nodule by 34% (39.0 cu mm to 52.2 cu mm) at three-month follow-up. Images of R2 Technology's ImageChecker system courtesy of Haili Chui, Ph.D. |
![]() |
![]() |
The results showed that for each volume, the relative bias and corresponding standard deviation of CAD volume measurements remained under 2% (from -2% to +2%). No statistically significant change was seen as a result of varying exposure levels, Chui said.
Next, using a database of patient scans acquired from 1.5 to 13 months apart, the system was instructed to find each nodule, create a correspondence with its counterpart in the later exam, and measure the volume difference, if any, in the size of nodules. The system detected 24/28 nodules (85.7%) in both initial and follow-up scans, and 26/28 (92%) were detected in at least one of the scans. The system determined the correct correspondences in 100% of the scans in which the nodules were detected in both exams. There were an average of 2.5 false-positives per case.
"You can see in this nodule 52% growth over two months," he said of one case. "Another nodule in this (same patient) showed no growth at all...and so basically the system helps the efficiency of the workflow in nodule detection, tracking, and measurement," he said. FDA approval is pending.
Siemens Medical Solutions discussed its similar Real-Time Automatic Matching System at the 2002 RSNA meeting.Automated volume measurement
In another study from the same 2003 RSNA session, Dr. Denis Tack from the radiology department of CHU de Charleroi in Belgium presented research designed to test the reproducibility of nodule volume measurements in vivo. Sixty-four patients underwent three successive thoracic CT scans on the same day; two using standard-dose protocols at 100 effective mAs, and one using a low-dose protocol at 20 effective mAs. All three protocols used 100 kVp, 4 x 1-mm slices, pitch 1.75:1, 1.5 mm collimation, and 1-mm reconstruction interval on a Somatom Volume Zoom scanner (Siemens Medical Solutions, Erlangen Germany).
Lung CARE, the first commercial application of Siemens' Interactive Computer-Aided Diagnosis (ICAD) system, was used to measure volumes in 121 non-calcified lung nodules visualized in 64 patients whose mean age was 63. The nodules were classified as regular (R) or irregularly (I) shaped. A thoracic radiologist and a resident measured each nodule twice, comparing the results to the automated measurement by means of a t-test.
The variation between automated and manual measurements for the two normal-dose scans was 5.5% for all nodules (R: 4.8%; I: 6.8%) range 0.1% to 34.4%. The results were similar for the low-dose exams. The mean volume differences between both radiologists were 7% for all nodules (R: 6; I 9.2%), according to an accompanying abstract.
The group concluded that nodule volume could vary depending on the operator, the nodule shape, and the radiation dose. Therefore, automated measurements should be undertaken with caution.
Problem solved with ultra-thin CT?
However, increased resolution might be expected to improve accuracy markedly. Dr. W. J. Kostis and colleagues from Cornell University in New York City performed a spherical phantom study that compared automated volume measurements on two scanners: a four-row LightSpeed Plus scanner (1.25-mm resolution), and a next-generation volumetric prototype scanner (VCT) capable of 0.2-mm native isotropic resolution (both from GE Healthcare, Waukesha, WI).
The group found marked reductions in the variability of automated volumes measured on volumetric-scanner images. The coefficient variation for spheres ranged from 1.4% to 4.1% on the four-slice scanner, and from 0.8% to 1.7% on the VCT. The variations for a set of non-spherical phantoms with increasing spatial frequency were 1%, 1.7%, and 2.1% for MDCT, respectively, and 0.4%, 0.6%, and 0.4% on the VCT, according to the abstract.
Kostis concluded that high-resolution VCT imaging yielded far better reproducibility than the multislice machine, and that its advantage over MDCT was more pronounced in non-spherical phantoms.
Malignant nodules enhance more brightly
In another study, Dr. Kyung So Lee and colleagues from the Sunkyunkwan University School of Medicine in Tokyo, Japan, found a high negative predictive value for malignancy in solitary pulmonary nodules (SPN) that do not enhance with the use of contrast.
"Assessment of tumor vascularity by using contrast enhancement has been shown to be useful for the characterization of benign from malignant nodules," Lee said, citing a study by Swensen, et al (Radiology, January 2000, Vol. 214:1, pp. 73-80).
Lee noted that the main problem of such assessments -- wide variations in the visibility of SPN -- are diminishing with the increasing use of thin-section MDCT imaging in recent years.
The group performed thin-section MDCT imaging on 192 patients (mean age 56) with SPN 30 mm or smaller in the study. Images were acquired along the z-axis at 50 mAs, 120 kVp, pitch of 0.75, 0.8-sec rotation, and 2.5-mm collimation with 1.25-mm reconstructions, at 20-second intervals for three minutes. Contrast was administered before CT (120 mL, 300 mg/ml iodinated contrast, 3 mL/sec).
Pathologically proven nodules were found in 12 patients, including benign SPN in 38 patients (37%), and malignant in 64 (63%). The nodules were analyzed for peak attenuation, net enhancement, and on a time-enhancement curve.
Using 30 HU net enhancement as a threshold, the researchers found that peak enhancement was significantly higher in malignant lesions (mean 98 HU, range 82-124 HU) than in benign lesions (mean 79 HU, range 48-110 HU). The pattern was similar in net enhancement (malignant mean 54 HU, range 28-70 HU; benign mean 39 HU, range 11-67 HU). The sensitivity for malignant nodules was 98% (63/64), specificity was 47% (8/19), the positive predictive value 76%, and negative predictive value 95%. False-positives included 6/9 tuberculomas, 4/4 sclerosing hemangiomas, and one each of the following: blastocystoma, abscess, inflammatory pseudotumor, and intrapulmonary lymph node.
"Our dynamic-enhancement study using MDCT showed high sensitivity and negative predictive value for nonmalignant nodules," Lee concluded. "However, there was low specificity due to highly enhancing benign nodules." The 30-HU threshold was used because it yielded the most accurate results, he said in response to a question from the audience.
Nodule characterization is inconsistent
A study presented by Sara Rogers from the University of California, Los Angeles highlighted the difficulties radiologists encounter in the assessment of lung nodules for malignancy. CAD systems capable of evaluating features such as shape and textures are in their infancy, however.
"Lung nodules seen on CT are often characterized by their size, shape, margin, and their attenuation," she said. However, because interobserver variability in determining these characteristics may affect diagnostic accuracy, the group conducted a study to assess variations among readers. They recruited five board-certified thoracic radiologists for the task, who were provided with visual training in nodule descriptors.
The group retrospectively evaluated 11 CT lung studies acquired at 120 kVp, 40-80 mAs, 3-mm collimation, and 1.5 to 2-mm reconstruction intervals. The readers were asked to evaluate each nodule identified using five descriptors for nodule shape and four for nodule margin, and seven descriptors for characteristics relating to nodule attenuation.
"Radiologists marked all the nodules they found, and for each nodule they marked they completed a nodule worksheet ... (that) keeps track of ID, diameters, how confident they are it's a nodule, how subtle it is, and how suspicious for cancer," Brown said.
The attenuation characteristics including "calcified and ground-glass descriptors had good agreement among the readers," she said. Kappa values for calcified and ground-glass descriptors were above 0.8. "The 'soft-tissue' descriptors had moderate agreement (k=0.52); the 'mixed' attenuation only sight agreement (k=0.18) between the readers," she said.
Shapes defined as "round/ovoid" or "triangular/polygonal" showed a fair amount of agreement, (k=0.31 and k=0.34, respectively), while evaluations of "lobular," "ill-defined" and "other" shapes had poor agreement. Agreement was also poor for the margin descriptors (smooth, spiculated, serrated, poorly defined or other) with kappa values under 0.2.
![]() |
Radiologists' descriptions of lung nodules varied widely depending on the attribute. All images courtesy of Sara Rogers, MS. |
![]() |
![]() |
![]() |
![]() |
There was little agreement about nodules of mixed attenuation and generally poor interobserver agreement regarding shape and margin characteristics, Brown concluded. Poor agreement on such subjectively obtained nodule characteristics "may affect the ability to use these measurements in correlative studies of malignancy rates" among different types of nodule, she said.
Rads need CAD
"I'm afraid I'm going to do a little more radiologist bashing in terms of looking at our ability not necessarily to characterize nodules, but to be able to identify them in the first place," said Dr. Geoffrey Rubin from Stanford University in Stanford, CA, who presented the closing study.
"I think that as we emerge into an era of CAD and alternative methods of imaging lung nodules...we need to review the question of what is ground truth," he said. "And this (question) is very goal-dependent. If we're looking exclusively for a diagnosis of cancer, then a pathological standard is probably the most reliable. But when we consider the task of ... radiologists interpreting chest CT scans, what we're really doing is trying to have a standard for what is a nodule -- something that we might want to perform further characterization of, and further follow-up." Radiologists know that CT's specificity for the appearance of benignity or malignancy "is not so great," he said. "So we're stuck looking for a radiologic standard if we're talking about nodule detection."
The goal was to determine the adequacy of traditional CT viewing methods for establishing consensus panel-based reference standards for the presence of pulmonary nodules. Twenty consecutive patients (mean age 64) underwent CT imaging to evaluate lung nodules or establish their presence. CT images were acquired using a four-row multislice scanner with 1.25-mm thickness and 0.8-mm reconstruction intervals.
On a standard clinical workstation, two thoracic radiologists reviewed the studies using cine paging of transverse sections, and flexible window/level width selection, recording and identifying the parameters of each nodule. Then, three additional radiologists reviewed the same 20 scans with the aid of a CAD system developed at Stanford.
Then, each of the 1,294 nodules identified by CAD and all the readers were randomized and re-examined by the consensus panel of two thoracic radiologists. Special software enabled the assessment in three planes using stacked paging, thin-slab MIPs, cartwheel views, and volume renderings. Finally, a free search was conducted to detect additional nodules.
The initial consensus panel found 88 nodules 3 mm and larger, and 13 calcified nodules. After the three radiologists plus CAD had had their turn, the second consensus panel found 195 nodules 3 mm and larger, and 39 calcified nodules in 19/20 patients.
"We see a substantial difference -- a 122% increase in the number of nodules detected -- by creating a reference standard where radiologists are arbiters in looking at detections made by other radiologists and by CAD," Rubin said.
In addition, the uncalcified nodules ³ 3 mm tended to be larger (6.4 mm ± 2.6 mm versus 4.1 mm ± 1.3 mm p<0.01) and less commonly peripherally located (59% versus 71%, p=0.08) than those identified in the second consensus panel.
"It flies in the face of conventional wisdom in that many of us believe that peripheral lung nodules are easier to detect than central lung nodules," he said. But the second standard helped find more peripheral nodules, perhaps owing to the fact that with very thin sections there is more confusion in the identification of vessels versus nodules.
"I think, particularly when we look to the literature, that when we simply rely on radiologists to detect nodules, we're going to be missing important lesions," he said. "Traditional approaches to establishing nodule presence fail to identify substantial numbers of pulmonary nodules, particularly when applied to large thin-section MDCT scans. Incorporation of 3-D analytical tools facilitates their confident identification on thin-section CT."
By Eric BarnesAuntMinnie.com staff writer
March 5, 2004
Related Reading
Pulmonary nodules not uncommon in lung transplant recipients, February 6, 2004
Lung cancer risk may be higher in female smokers, February 5, 2004
U.S. panel to consider lung nodule screening tool, February 3, 2004
Lung CAD makes strides in nodule detection, January 30, 2004
Copyright © 2004 AuntMinnie.com