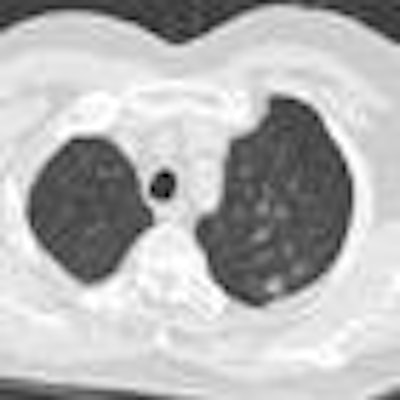
VIENNA - Taking a step beyond lung nodule detection schemes evaluated in previous studies, researchers from the Netherlands have developed an automated method of characterizing nodules as solid, part-solid, or nonsolid, pointing the way to different patient management paths, according to a presentation at ECR 2013.
In results from more than 100 patients who underwent lung cancer screening with CT as part of the Nederlands-Leuvens Longkanker Screenings Onderzoek (NELSON) lung cancer screening trial, researchers from Radboud University Nijmegen Medical Centre in the Netherlands and several other institutions showed that their automated computer-aided detection (CAD) scheme for characterizing nodule types according to Fleischner Society criteria was nearly as accurate as the findings of an expert thoracic radiologist -- who despite his experience, expressed his own doubts about his ability to classify nodules correctly in every case.
Classifying lesions not so easy
The original Fleischner Society guidelines for the management of incidentally detected pulmonary nodules, published in 2005, assess risk based on nodule size but make no distinction for nodule type, except for a side note cautioning that part-solid nodules may require longer follow-up to exclude adenocarcinoma, explained Colin Jacobs, from the department of radiology's Diagnostic Image Analysis Group, in his ECR talk. But updated guidelines issued in 2008 (Radiology, March 2008, Vol. 246:3, pp. 697-722) distinguished solid, part-solid, and nonsolid nodules or ground-glass opacities in their management recommendations that radiologists must now follow.
"Now we have guidelines for solid nodules, for subsolid nodules, and the guidelines for subsolid nodules also differentiate between part-solid and nonsolid nodules," Jacobs said. "Classifying nodules in these three categories is crucial for patient management. The purpose of this study was to develop a CAD tool to do this automatically ... and to compare the performance of our CAD tool to an experienced thoracic radiologist on a large dataset obtained from a screening database."
Also participating in the study were investigators from Fraunhofer MEVIS of Bremen, Germany; Kennemer Gasthuis of Haarlem, the Netherlands; Meander Medical Centre in Amersfoort, Netherlands; and the University Medical Center Utrecht in the Netherlands.
Automatic nodule characterization CAD
The characterization scheme is initiated by the user inputting a seed point in the center of the nodule on the CT image. Then a volumetric segmentation algorithm is applied using different parameter settings to segment the complete nodule, the ground-glass components, and the solid core, Jacobs said. Each segmentation computes volumetric features to describe part of the nodule, and then a supervised classifier is applied to classify the candidates as solid, part-solid, or nonsolid.
"For each segmentation, it computes volume, mass, average density, the fifth percentile, and the 95th percentile of densities inside the segmentation," he said. All features are collected, and a supervised classifier, trained with examples, is used to classify the candidates into one of the three categories.
To be included in the analysis, a scan from one of two sites in the Dutch-Belgium NELSON lung cancer screening trial had to have a solid, part-solid, or nonsolid nodule between 7 mm and 30 mm in diameter. Nodules were originally scanned on a 16-detector-row scanner (0.75-mm collimation, 120-140 kVp, 30 mAs). The study analyzed 137 nodules, including 52 solid nodules, 50 part-solid nodules, and 50 nonsolid nodules as recorded in the NELSON database, with data populated by three radiologists who were asked to characterize them.
In all, three analyses were performed on the data: first, evaluation of the tool that defined solid and subsolid nodules, then testing of the algorithm's performance in detecting three different nodule subtypes, with the final analysis to see how well the application could differentiate subsolid from part-solid nodules, Jacobs said.
"We evaluated the accuracy of the automatic nodule characterization tool using leave-one-nodule-out cross-validation," he said. Experiments included solid/subsolid differentiation (n = 152) and solid/part-solid/nonsolid differentiation (n = 152). Finally came the hardest test for man and machine: part-solid/nonsolid differentiation in 100 datasets.
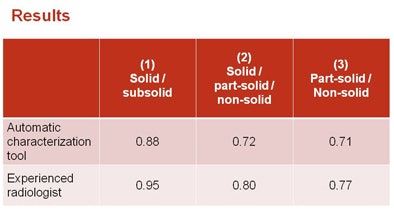
The experienced thoracic radiologist slightly outperformed the algorithm in all three categories; however, the radiologist clearly needed help -- saying that he was uncertain about his classification in 25% of the cases, Jacobs said.
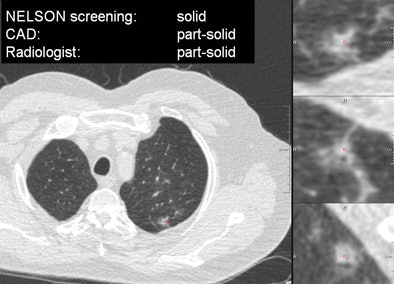
"Nodule characterization is a difficult task, indicated by 25% of the cases in which an experienced radiologist was uncertain about his classifications, especially the differentiation between part-solid and nonsolid nodules. It's a difficult part, both for the CAD and the radiologist," Jacobs said. "And we did not yet assess the interobserver variability of the radiologist."
Future work will incorporate size measurements of solid component as indicated by the new guidelines for subsolid nodules, he said.
"A novel automated nodule characterization tool has been developed and evaluated on a large database," Jacobs said. "It shows promising performance, and we think this could aid radiologists in selecting the appropriate workup for pulmonary nodules."
In order to combine the tool with other needed capabilities, "we're also working on automated detection of pulmonary nodules, and then we're working on screening workstations, and then to classify the malignancy likelihood, because the likelihood is higher for subsolid nodules than for solid nodules," Jacobs said in response to a question from session moderator Dr. Thomas Mang, from the Medical University of Vienna. Jacobs also confirmed that, absent histologic analysis, the ground truth regarding each nodule was all based on radiologists' opinions.